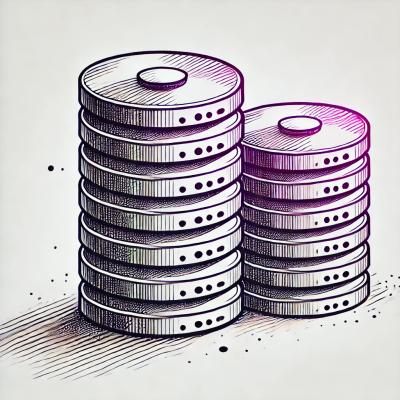
Security News
MCP Community Begins Work on Official MCP Metaregistry
The MCP community is launching an official registry to standardize AI tool discovery and let agents dynamically find and install MCP servers.
github.com/LiYangHart/Hyperparameter-Optimization-of-Machine-Learning-Algorithms
This code provides a hyper-parameter optimization implementation for machine learning algorithms, as described in the paper:
L. Yang and A. Shami, “On hyperparameter optimization of machine learning algorithms: Theory and practice,” Neurocomputing, vol. 415, pp. 295–316, 2020, doi: https://doi.org/10.1016/j.neucom.2020.07.061.
To fit a machine learning model into different problems, its hyper-parameters must be tuned. Selecting the best hyper-parameter configuration for machine learning models has a direct impact on the model's performance. In this paper, optimizing the hyper-parameters of common machine learning models is studied. We introduce several state-of-the-art optimization techniques and discuss how to apply them to machine learning algorithms. Many available libraries and frameworks developed for hyper-parameter optimization problems are provided, and some open challenges of hyper-parameter optimization research are also discussed in this paper. Moreover, experiments are conducted on benchmark datasets to compare the performance of different optimization methods and provide practical examples of hyper-parameter optimization.
This paper and code will help industrial users, data analysts, and researchers to better develop machine learning models by identifying the proper hyper-parameter configurations effectively.
On Hyperparameter Optimization of Machine Learning Algorithms: Theory and Practice
One-column version: arXiv
Two-column version: Elsevier
Section 3: Important hyper-parameters of common machine learning algorithms
Section 4: Hyper-parameter optimization techniques introduction
Section 5: How to choose optimization techniques for different machine learning models
Section 6: Common Python libraries/tools for hyper-parameter optimization
Section 7: Experimental results (sample code in "HPO_Regression.ipynb" and "HPO_Classification.ipynb")
Section 8: Open challenges and future research directions
Summary table for Sections 3-6: Table 2: A comprehensive overview of common ML models, their hyper-parameters, suitable optimization techniques, and available Python libraries
Summary table for Sections 8: Table 10: The open challenges and future directions of HPO research
Sample code for hyper-parameter optimization implementation for machine learning algorithms is provided in this repository.
HPO_Regression.ipynb
Dataset used: Boston-Housing
HPO_Classification.ipynb
Dataset used: MNIST
ML Model | Hyper-parameter | Type | Search Space |
---|---|---|---|
RF Classifier | n_estimators | Discrete | [10,100] |
max_depth | Discrete | [5,50] | |
min_samples_split | Discrete | [2,11] | |
min_samples_leaf | Discrete | [1,11] | |
criterion | Categorical | 'gini', 'entropy' | |
max_features | Discrete | [1,64] | |
SVM Classifier | C | Continuous | [0.1,50] |
kernel | Categorical | 'linear', 'poly', 'rbf', 'sigmoid' | |
KNN Classifier | n_neighbors | Discrete | [1,20] |
ANN Classifier | optimizer | Categorical | 'adam', 'rmsprop', 'sgd' |
activation | Categorical | 'relu', 'tanh' | |
batch_size | Discrete | [16,64] | |
neurons | Discrete | [10,100] | |
epochs | Discrete | [20,50] | |
patience | Discrete | [3,20] | |
RF Regressor | n_estimators | Discrete | [10,100] |
max_depth | Discrete | [5,50] | |
min_samples_split | Discrete | [2,11] | |
min_samples_leaf | Discrete | [1,11] | |
criterion | Categorical | 'mse', 'mae' | |
max_features | Discrete | [1,13] | |
SVM Regressor | C | Continuous | [0.1,50] |
kernel | Categorical | 'linear', 'poly', 'rbf', 'sigmoid' | |
epsilon | Continuous | [0.001,1] | |
KNN Regressor | n_neighbors | Discrete | [1,20] |
ANN Regressor | optimizer | Categorical | 'adam', 'rmsprop' |
activation | Categorical | 'relu', 'tanh' | |
loss | Categorical | 'mse', 'mae' | |
batch_size | Discrete | [16,64] | |
neurons | Discrete | [10,100] | |
epochs | Discrete | [20,50] | |
patience | Discrete | [3,20] |
Please feel free to contact me for any questions or cooperation opportunities. I'd be happy to help.
If you find this repository useful in your research, please cite this article as:
L. Yang and A. Shami, “On hyperparameter optimization of machine learning algorithms: Theory and practice,” Neurocomputing, vol. 415, pp. 295–316, 2020, doi: https://doi.org/10.1016/j.neucom.2020.07.061.
@article{YANG2020295,
title = "On hyperparameter optimization of machine learning algorithms: Theory and practice",
author = "Li Yang and Abdallah Shami",
volume = "415",
pages = "295 - 316",
journal = "Neurocomputing",
year = "2020",
issn = "0925-2312",
doi = "https://doi.org/10.1016/j.neucom.2020.07.061",
url = "http://www.sciencedirect.com/science/article/pii/S0925231220311693"
}
FAQs
Unknown package
Did you know?
Socket for GitHub automatically highlights issues in each pull request and monitors the health of all your open source dependencies. Discover the contents of your packages and block harmful activity before you install or update your dependencies.
Security News
The MCP community is launching an official registry to standardize AI tool discovery and let agents dynamically find and install MCP servers.
Research
Security News
Socket uncovers an npm Trojan stealing crypto wallets and BullX credentials via obfuscated code and Telegram exfiltration.
Research
Security News
Malicious npm packages posing as developer tools target macOS Cursor IDE users, stealing credentials and modifying files to gain persistent backdoor access.