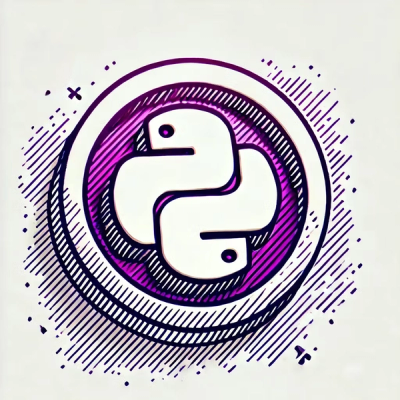
Product
Socket Now Supports pylock.toml Files
Socket now supports pylock.toml, enabling secure, reproducible Python builds with advanced scanning and full alignment with PEP 751's new standard.
github.com/sql-machine-learning/sqlflow
SQLFlow is a bridge that connects a SQL engine, e.g. MySQL, Hive or MaxCompute, with TensorFlow, XGBoost and other machine learning toolkits. SQLFlow extends the SQL syntax to enable model training, prediction and model explanation.
The current experience of development ML based applications requires a team of data engineers, data scientists, business analysts as well as a proliferation of advanced languages and programming tools like Python, SQL, SAS, SASS, Julia, R. The fragmentation of tooling and development environment brings additional difficulties in engineering to model training/tuning. What if we marry the most widely used data management/processing language SQL with ML/system capabilities and let engineers with SQL skills develop advanced ML based applications?
There are already some work in progress in the industry. We can write simple machine learning prediction (or scoring) algorithms in SQL using operators like DOT_PRODUCT
. However, this requires copy-n-pasting model parameters from the training program to SQL statements. In the commercial world, we see some proprietary SQL engines providing extensions to support machine learning capabilities.
CREATE MODEL
statement.None of the existing solution solves our pain point, instead we want it to be fully extensible.
Here are examples for training a TensorFlow DNNClassifer model using sample data Iris.train, and running prediction using the trained model. You can see how cool it is to write some elegant ML code using SQL:
sqlflow> SELECT *
FROM iris.train
TO TRAIN DNNClassifier
WITH model.n_classes = 3, model.hidden_units = [10, 20]
COLUMN sepal_length, sepal_width, petal_length, petal_width
LABEL class
INTO sqlflow_models.my_dnn_model;
...
Training set accuracy: 0.96721
Done training
sqlflow> SELECT *
FROM iris.test
TO PREDICT iris.predict.class
USING sqlflow_models.my_dnn_model;
...
Done predicting. Predict table : iris.predict
SQLFlow will love to support as many mainstream ML frameworks and data sources as possible, but we feel like the expansion would be hard to be done merely on our own, so we would love to hear your options on what ML frameworks and data sources you are currently using and build upon. Please refer to our roadmap for specific timelines, also let us know your current scenarios and interests around SQLFlow project so we can prioritize based on the feedback from the community.
Your feedback is our motivation to move on. Please let us know your questions, concerns, and issues by filing GitHub Issues.
FAQs
Unknown package
Did you know?
Socket for GitHub automatically highlights issues in each pull request and monitors the health of all your open source dependencies. Discover the contents of your packages and block harmful activity before you install or update your dependencies.
Product
Socket now supports pylock.toml, enabling secure, reproducible Python builds with advanced scanning and full alignment with PEP 751's new standard.
Security News
Research
Socket uncovered two npm packages that register hidden HTTP endpoints to delete all files on command.
Research
Security News
Malicious Ruby gems typosquat Fastlane plugins to steal Telegram bot tokens, messages, and files, exploiting demand after Vietnam’s Telegram ban.