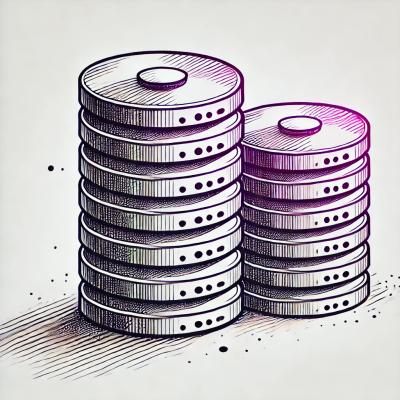
Security News
MCP Community Begins Work on Official MCP Metaregistry
The MCP community is launching an official registry to standardize AI tool discovery and let agents dynamically find and install MCP servers.
optimization-js
Advanced tools
A javascript library implementing useful multivariate function optimization procedures, which allow to find a local minimum of some function of a vector argument. Such argument is a javascript array.
Example zero order optimization in JavaScript (no need to provide gradient information!):
<script src="./optimization.js"></script>
<script>
// objective that needs to be minimized
fnc = function (v) {
var result = 0.0;
for (var i = 0; i < v.length; i++){
result = result + v[i] * v[i]
}
return result;
};
var x0 = [1.0, -1.0, 0.5, -0.5, 0.25, -0.25]; // a somewhat random initial vector
// Powell method can be applied to zero order unconstrained optimization
var solution = optimjs.minimize_Powell(fnc, x0);
</script>
For more examples, check out examples
folder.
For use in browser, use this:
<script src="https://unpkg.com/optimization-js@latest/dist/optimization.js"></script>
In order to use this in node, use npm:
npm install optimization-js
Documentation is hosted on github pages here: http://optimization-js.github.io/optimization-js/.
Gradient free:
Requires gradient:
See JavaScript files in examples
folder for examples on how to use the algorithms.
FAQs
Mathematical optimization in JavaScript.
The npm package optimization-js receives a total of 173 weekly downloads. As such, optimization-js popularity was classified as not popular.
We found that optimization-js demonstrated a not healthy version release cadence and project activity because the last version was released a year ago. It has 1 open source maintainer collaborating on the project.
Did you know?
Socket for GitHub automatically highlights issues in each pull request and monitors the health of all your open source dependencies. Discover the contents of your packages and block harmful activity before you install or update your dependencies.
Security News
The MCP community is launching an official registry to standardize AI tool discovery and let agents dynamically find and install MCP servers.
Research
Security News
Socket uncovers an npm Trojan stealing crypto wallets and BullX credentials via obfuscated code and Telegram exfiltration.
Research
Security News
Malicious npm packages posing as developer tools target macOS Cursor IDE users, stealing credentials and modifying files to gain persistent backdoor access.