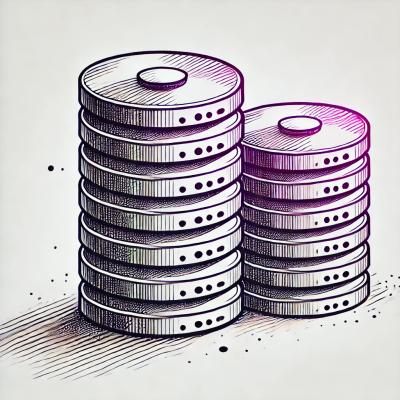
Security News
MCP Community Begins Work on Official MCP Metaregistry
The MCP community is launching an official registry to standardize AI tool discovery and let agents dynamically find and install MCP servers.
This repository contains a JavaScript module designed to facilitate the preprocessing of datasets for machine learning applications. The primary functionality of the module is to scale feature data using normalization or standardization methods, and to pa
Machine Learning Data Preparation Toolkit
XY Splitting, Feature Weighting, Standardization, and MinMax Scaling in JavaScript
xy-scale.js
is a robust toolkit designed for scaling and preparing datasets in JavaScript, specifically tailored for machine learning applications. It offers essential utilities for:
parseTrainingXY
and parseProductionX
allow for flexible handling of features and labels.Install the toolkit via npm:
npm install xy-scale
parseTrainingXY
Processes a dataset for supervised learning by scaling and splitting it into training and testing subsets. It supports configurable options for feature grouping, weighting, and scaling.
Parameters:
arrObj
(Array of Objects): Input data containing features (X) and labels (Y) e.g, [{high: 10, low: 8, close: 9, dayOfTheWeek: 'monday'}, {high: 11, low: 8, close: 10, dayOfTheWeek: 'tuesday'}]
. Each Item an be a number
, string
or boolean
.trainingSplit
(Number, optional): Fraction of data for training (default: 0.8
).yCallbackFunc
(Function): Extracts Y values from each row. Returns null
or undefined
to exclude a row.xCallbackFunc
(Function): Extracts X values from each row. Returns null
or undefined
to exclude a row.groups
(Object, optional): Groups continuous values features into categories after yCallbackFunc
and xCallbackFunc
callbacks are applied (e.g., { ohlc: ['open', 'high', 'low', 'close'] }
). Each feature belonging to a group will be MinMax scaled or standardized using the group's properties (min
, max
, std
).shuffle
(Boolean, optional): Randomizes data order after yCallbackFunc
and xCallbackFunc
callbacks are applied (default: false
).repeat
(Object, optional): Determines feature repetition after yCallbackFunc
and xCallbackFunc
callbacks are applied.balancing
(String, optional): Handles inbalanced datasets applying oversample
or undersample
to X
and Y
(defaults to null
);Returns:
trainX
, trainY
: Scaled training features and labels.testX
, testY
: Scaled testing features and labels.configX
, configY
: Scaling configuration objects for features and labels.keyNamesX
, keyNamesY
: Key names reflecting feature repetition and grouping.parseProductionX
Parses and scales unseen production data for feature preparation.
Parameters:
arrObj
(Array of Objects): Input data containing features (X) and labels (Y) e.g, [{high: 10, low: 8, close: 9, dayOfTheWeek: 'monday'}, {high: 11, low: 8, close: 10, dayOfTheWeek: 'tuesday'}]
. Each Item an be a number
, string
or boolean
.repeat
(Object, optional): Determines feature repetition after yCallbackFunc
and xCallbackFunc
callbacks are applied.xCallbackFunc
(Function): Extracts X values from each row. Returns null
or undefined
to exclude a row.groups
(Object, optional): Groups continuous values features into categories after yCallbackFunc
and xCallbackFunc
callbacks are applied (e.g., { ohlc: ['open', 'high', 'low', 'close'] }
). Each feature belonging to a group will be MinMax scaled or standardized using the group's properties (min
, max
, std
).shuffle
(Boolean, optional): Randomizes data order after yCallbackFunc
and xCallbackFunc
callbacks are applied (default: false
).Returns:
X
: Scaled feature array.configX
: Scaling configuration for features.keyNamesX
: Key names reflecting feature repetition and grouping.descaleArrayObj
Reconstructs original data values from scaled arrays.
Parameters:
scaled
(Array): Scaled data array (e.g., trainX
, trainY
, etc.).config
(Object): Configuration object returned from parseTrainingXY
or parseProductionX
.keyNames
(Array): Key names reflecting feature repetition and grouping.Returns:
arrayToTimesteps
Converts a flat array into sequences for time-series modeling.
Parameters:
arr
(Array): Input array.timeSteps
(Number): Length of each sequence.
timeSteps === 0
, returns the original array.timeSteps < 0
, throws an error.Returns:
timeSteps
elements.import { parseTrainingXY, parseProductionX, descaleArrayObj, arrayToTimesteps } from 'xy-scale';
import { loadFile } from './fs.js';
import * as tf from '@tensorflow/tfjs-node';
(async () => {
const myArray = await loadFile({ fileName: '1d-spy.json', pathName: 'datasets' });
// Callback function for parsing `X` features
const xCallbackFunc = ({ objRow, index }) => {
const curr = objRow[index];
const prev = objRow[index - 1];
if (typeof prev === 'undefined') return null;
const { open, high, low, close, volume } = curr;
return {
open,
high,
low,
close,
change: open - prev.close,
top: high - Math.max(open, close),
bottom: low - Math.min(open, close),
body: open - close,
};
};
// Callback function for parsing `Y` labels
const yCallbackFunc = ({ objRow, index }) => {
const curr = objRow[index];
const next = objRow[index + 1];
if (typeof next === 'undefined') return null;
return {
label_1: next.open > curr.close,
label_2: next.close > curr.close,
};
};
// Parse and scale training data
const {
trainX,
trainY,
testX,
testY,
configX,
keyNamesX,
} = parseTrainingXY({
arrObj: myArray,
trainingSplit: 0.9,
yCallbackFunc,
xCallbackFunc,
groups: { ohlc: ['open', 'high', 'low', 'close'] },
shuffle: true,
repeat: { close: 20 },
balancing: null, //oversample or undersample
});
// Time-stepping and TensorFlow integration
const timeSteps = 10;
const timeSteppedTrainX = arrayToTimesteps(trainX, timeSteps);
const trimmedTrainY = trainY.slice(timeSteps - 1);
const inputX = tf.tensor3d(timeSteppedTrainX, [timeSteppedTrainX.length, timeSteps, trainX[0].length]);
const targetY = tf.tensor2d(trimmedTrainY, [trimmedTrainY.length, trainY[0].length]);
console.log('configX', configX);
console.log('inputX', inputX);
console.log('targetY', targetY);
})();
For more detailed documentation, examples, and support, please visit the GitHub repository.
This project is licensed under the MIT License.
FAQs
This repository contains a JavaScript module designed to facilitate the preprocessing of datasets for machine learning applications. The primary functionality of the module is to scale feature data using normalization or standardization methods, and to pa
The npm package xy-scale receives a total of 23 weekly downloads. As such, xy-scale popularity was classified as not popular.
We found that xy-scale demonstrated a healthy version release cadence and project activity because the last version was released less than a year ago. It has 1 open source maintainer collaborating on the project.
Did you know?
Socket for GitHub automatically highlights issues in each pull request and monitors the health of all your open source dependencies. Discover the contents of your packages and block harmful activity before you install or update your dependencies.
Security News
The MCP community is launching an official registry to standardize AI tool discovery and let agents dynamically find and install MCP servers.
Research
Security News
Socket uncovers an npm Trojan stealing crypto wallets and BullX credentials via obfuscated code and Telegram exfiltration.
Research
Security News
Malicious npm packages posing as developer tools target macOS Cursor IDE users, stealing credentials and modifying files to gain persistent backdoor access.