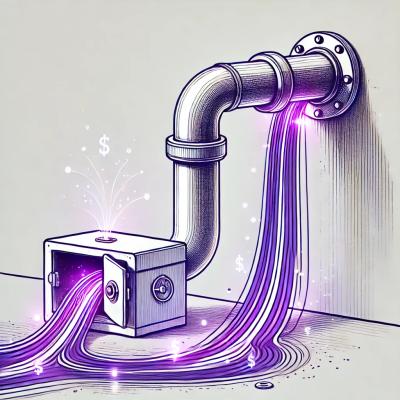
Research
Security News
Malicious npm Package Targets Solana Developers and Hijacks Funds
A malicious npm package targets Solana developers, rerouting funds in 2% of transactions to a hardcoded address.
An exercise to create a Python package, build it, test it, distribute it, and use it. See instructions for details.
This package was created by generally following the Packaging Python Projects with the addition of some pipenv setup to manage virtual environments.
Finance is boring and you hate crunching numbers all day? No problem, Brainrot-Stocks is a Python package designed for handling financial data while keeping you entertained with a constant stream of brainrot. It includes modules for managing stock quotes, performing various operations on financial data, and engaging learning experiences. Powered by AlphaVantage API.
https://pypi.org/project/Brainrot-Stocks/#description
Reyhan Abdul Quayum | Rashed Alneyadi | Sia Chen | Yu Zhang | Chloe Han |
---|---|---|---|---|
quotes.py
: Handles operations related to stock quotes.stock.py
: Manages stock data and related functionality.test_stock.py
: Tests the stock.py
module.See the example usage Python program to see all the functions in action here.
from Financiers.stock import Stock
stock = Stock()
Stock
that contains functions which access data from AlphaVantage's API for your Python programs. To do this, import Stock
and create an instance of it.get_market_mood()
stock = Stock()
mood = stock.get_market_mood()
get_earnings(symbol_string, annual=True, numDays=5)
earnings_df = stock.get_earnings("AAPL", annual=True, numDays=5)
symbol_string
(str): Ticker symbol of the stock (e.g., "AAPL"
).annual
(bool): If True
, retrieves annual earnings; if False
, retrieves quarterly earnings.numDays
(int): Number of recent entries to return.BrainrotDataFrame
with a random brainrot quote and a DataFrame of earnings data.get_price_data(symbol_string)
price_data = stock.get_price_data("AAPL")
symbol_string
(str): Ticker symbol of the stock.BrainrotDataFrame
with the stock's historical price data.forecast_prices(symbol_string, days=30)
forecast_df = stock.forecast_prices("AAPL", days=30)
symbol_string
(str): Ticker symbol of the stock.days
(int): Number of future days to forecast.BrainrotDataFrame
with forecasted dates and predicted prices.company_overview(symbol_string)
overview = stock.company_overview("AAPL")
symbol_string
(str): Ticker symbol of the company.BrainrotWrapper
with a Company
object containing information about the company.plot_top_movers(symbols)
top_movers_df = stock.plot_top_movers(["AAPL", "GOOGL", "TSLA"])
symbols
(list): List of stock ticker symbols.BrainrotDataFrame
with information on the top gainer and loser from the provided symbols. Generates plots for visual analysis.project_future_estimates(symbols, days=30, pattern="neutral")
estimates_df = stock.project_future_estimates(["AAPL", "GOOGL"], days=30, pattern="bullish")
symbols
(list): List of stock ticker symbols.days
(int): Number of days to forecast.pattern
(str): Trend pattern ("bullish"
, "bearish"
, or "neutral"
).BrainrotDataFrame
with forecasted prices for each symbol in the list.calculate_atr(data, window=14)
atr = stock.calculate_atr(data, window=14)
data
(DataFrame): DataFrame containing columns 'high'
, 'low'
, and 'close'
.window
(int): Period to calculate the Average True Range (default is 14).pd.Series
with ATR values.forecast_by_date_range(date_range="last_month", days_to_forecast=30, model_type="linear", confidence_level=0.95, frequency="daily", return_format="dataframe")
forecast = company.forecast_by_date_range(date_range="last_year", days_to_forecast=30, model_type="random_walk")
date_range
(str): Specifies the historical range for data ("last_day"
, "last_month"
, "last_year"
).days_to_forecast
(int): Number of days to forecast.model_type
(str): Forecasting model ("linear"
, "random_walk"
).confidence_level
(float): Confidence level for forecast intervals.frequency
(str): Frequency of forecasted data ("daily"
, "weekly"
, "monthly"
).return_format
(str): Format of the return data ("dataframe"
, "json"
).BrainrotDataFrame
or JSON with forecasted dates, prices, and confidence intervals (if applicable).These classes are designed to wrap data in a custom way, displaying both the data and a randomly selected "brainrot" quote for humorous effect. Each can be instantiated directly or will be returned by functions within the Stock
and Company
classes.
Prerequisites: have latest versions of pip and python installed on your environment.
pipenv
, and install the latest version of the package with the following command:pip install pipenv
pipenv install Brainrot-Stocks
And before moving onto the next step, activate the virtual environment:
pipenv shell
from Financiers.stock import Stock
Then, create an instance of Stock class:
stock = Stock()
And you're ready to go! Refer to this example file to see the example usage of package functions inside the code.
python <example_file>.py
Prerequisites: have latest versions of pip and python installed on your environment.
git clone https://github.com/software-students-fall2024/3-python-package-financeeers.git
cd 3-python-package-financeeers
If pipenv
has not been installed, install using the following command:
pip install pipenv
pipenv install
pipenv shell
.env
file in the root directory of the project, and add the following content:ALPHAVANTAGE_API_KEY = <api_key>
Replace <api_key> with the actual api key, which will be delivered to the admins via discord.
Make changes you want to make, and run the files. For example, if you want to run stock.py, run the following command:
python src/Financiers/stock.py
Make sure you are in the virtual environment by running the following command:
pipenv shell
Run all unit tests with the following command:
pytest
Build the package using the following command:
python -m build
Then, the artifacts will be produced at dist/
.
If you want to exit the virtual environment, use this comand:
exit
FAQs
Get stock data with unwanted brainrot in your Python app.
We found that Brainrot-Stocks demonstrated a healthy version release cadence and project activity because the last version was released less than a year ago. It has 1 open source maintainer collaborating on the project.
Did you know?
Socket for GitHub automatically highlights issues in each pull request and monitors the health of all your open source dependencies. Discover the contents of your packages and block harmful activity before you install or update your dependencies.
Research
Security News
A malicious npm package targets Solana developers, rerouting funds in 2% of transactions to a hardcoded address.
Security News
Research
Socket researchers have discovered malicious npm packages targeting crypto developers, stealing credentials and wallet data using spyware delivered through typosquats of popular cryptographic libraries.
Security News
Socket's package search now displays weekly downloads for npm packages, helping developers quickly assess popularity and make more informed decisions.