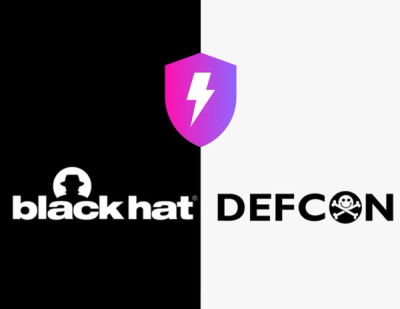
Security News
Meet Socket at Black Hat and DEF CON 2025 in Las Vegas
Meet Socket at Black Hat & DEF CON 2025 for 1:1s, insider security talks at Allegiant Stadium, and a private dinner with top minds in software supply chain security.
A2Perf provides benchmark environments in the following domains:
A2Perf can be installed on your local machine:
git clone https://github.com/Farama-Foundation/A2Perf.git
cd A2Perf
git submodule sync --recursive
git submodule update --init --recursive
pip install -e .[all]
To install specific packages, you can use the following commands:
pip install -e .[web_navigation]
pip install -e .[quadruped_locomotion]
pip install -e .[circuit_training]
Both x86-64 and Arch64 (ARM64) architectures are supported.
Please note that the Windows version is not as well-tested as Linux and macOS
versions.
It can be used for development and testing but if you want to conduct serious (
time and resource-extensive) experiments on Windows,
please consider
using Docker
or WSL with Linux
version.
Environments in A2Perf are registered under specific names for each domain and task. Here are the available environments:
Quadruped Locomotion:
QuadrupedLocomotion-DogPace-v0
QuadrupedLocomotion-DogTrot-v0
QuadrupedLocomotion-DogSpin-v0
Web Navigation:
WebNavigation-Difficulty-01-v0
WebNavigation-Difficulty-02-v0
WebNavigation-Difficulty-03-v0
Circuit Training:
CircuitTraining-ToyMacro-v0
CircuitTraining-Ariane-v0
For example, you can create an instance of the WebNavigation-Difficulty-01-v0
environment as follows:
import gymnasium as gym
from a2perf.domains import web_navigation
env = gym.make("WebNavigation-DifficultyLevel-01-v0", num_websites=10, seed=0)
A beginners guide to benchmarking with A2Perf is described here.
train.py
- defines a global train
function with the following
signature:
def train():
"""Trains the user's model."""
inference.py
- defines the following functions:
def load_policy(env, **load_kwargs):
"""Loads a trained policy model from the specified directory."""
def infer_once(policy, observation):
"""Runs a single inference step using the given policy and observation."""
def preprocess_observation(observation):
"""Preprocesses a raw observation from the environment into a format compatible with the policy."""
requirements.txt
- lists the required Python packages and
their versions for running the user's code__init__.py
- an empty file that allows the submission to be
imported as a Python moduleUnder
a2perf/submission/configs
,
there are default gin configuration files for training and inference for each
domain. These files define various settings and parameters for
benchmarking.
Here's an example of an training.gin
file for web navigation:
# ----------------------
# IMPORTS
# ----------------------
import a2perf.submission.submission_util
# ----------------------
# SUBMISSION SETUP
# ----------------------
# Set up submission object
Submission.mode = %BenchmarkMode.TRAIN
Submission.domain = %BenchmarkDomain.WEB_NAVIGATION
Submission.run_offline_metrics_only = False
Submission.measure_emissions = True
# ----------------------
# SYSTEM METRICS SETUP
# ----------------------
# Set up codecarbon for system metrics
track_emissions_decorator.project_name = 'a2perf_web_navigation_train'
track_emissions_decorator.measure_power_secs = 5
track_emissions_decorator.save_to_file = True # Save data to file
track_emissions_decorator.save_to_logger = False # Do not save data to logger
track_emissions_decorator.gpu_ids = None # Enter list of specific GPU IDs to track if desired
track_emissions_decorator.log_level = 'info' # Log level set to 'info'
track_emissions_decorator.country_iso_code = 'USA'
track_emissions_decorator.region = 'Massachusetts'
track_emissions_decorator.offline = True
Baselines for all tasks are provided and are described in the article supporting A2Perf.
A2Perf keeps strict versioning for reproducibility reasons. All environments end in a suffix like "-v0". When changes are made to environments that might impact learning results, the number is increased by one to prevent potential confusion. This follows the Gymnasium convention.
FAQs
Benchmarking suite for evaluating autonomous agents in real-world domains.
We found that a2perf demonstrated a healthy version release cadence and project activity because the last version was released less than a year ago. It has 1 open source maintainer collaborating on the project.
Did you know?
Socket for GitHub automatically highlights issues in each pull request and monitors the health of all your open source dependencies. Discover the contents of your packages and block harmful activity before you install or update your dependencies.
Security News
Meet Socket at Black Hat & DEF CON 2025 for 1:1s, insider security talks at Allegiant Stadium, and a private dinner with top minds in software supply chain security.
Security News
CAI is a new open source AI framework that automates penetration testing tasks like scanning and exploitation up to 3,600× faster than humans.
Security News
Deno 2.4 brings back bundling, improves dependency updates and telemetry, and makes the runtime more practical for real-world JavaScript projects.