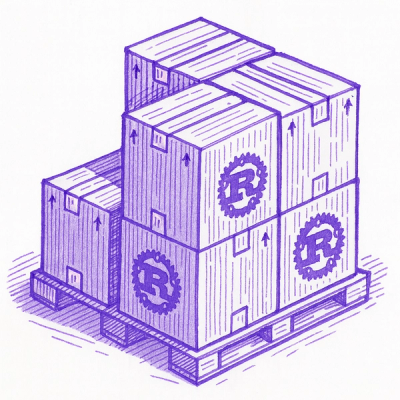
Security News
Crates.io Implements Trusted Publishing Support
Crates.io adds Trusted Publishing support, enabling secure GitHub Actions-based crate releases without long-lived API tokens.
This library aims to provide a tool to obtain an optimal dispach of a Power System comprised of Thermal Generation Units. The approach combines the Ant Colony Optimizer with a non-linear solver provided by CVXOPT.
This is an under development library
A pre-built binary wheel package can be installed using pip:
pip install acopoweropt
Poetry is a tool for dependency management and packaging in Python. acopoweropt
can be installed in a poetry managed project:
poetry add acopoweropt
From a domain perspective, there should be a complete decoupling between an Ant Colony and a Power System, after all ants do not have knowledge of power systems. This approach, although more elegant, is far from trivial to be implemented, mainly because the enviroment where the ants would look for food gets deeply entangled with the domain. For example, the modeling of pheromone matrix for the traveler salesman might not be adequate for a Power Systems Unit Commitment problem.
For that reason, the initial approach was to create two main Entities: A Power System
and a PowerColony
, where the first must be a Power System which can be solved by a mathematical method and the second should be an Ant Colony initialized to seek optimal results of a Power System problem.
Since the dispatch of "multi operative zone" Thermal Generation Units (TGUs) bring non-linearities to the formulation, obtaining a global optimal financial dispach of the system is not a trivial task. The Ant Colony algorithm came in hand as a tool to iteractively seek a global optimal result without having to rely on brute computational force.
The systems configuration should be defined in the systems.json
file. In the example provided, 3 systems where defined: 's10', 's15' and 's40', the names were chosen for convention and will be used by the PowerSystem
class to initialize the desired configuration.
The code below samples a possible configuration which can be used to operate the system and solves this configuration.
from acopoweropt import system
# Instance a PowerSystem class from a configuration file where 's10` defines a system configuration
PSystem = system.PowerSystem(name='s10')
# Randomly selects a possible system operation (there are cases where more than a single unit can be operated in diferent configurations)
operation = PSystem.sample_operation()
# Solve the Economic Dispatch of the units of a specific configuration of the system, in this case, let's use the previously sampled one:
solution = PSystem.solve(operation=operation)
# Prints total financial cost of the operation
print("Total Financial Cost: {}".format(solution.get('Ft')))
# Prints the operation with its power dispach values
print(solution.get('operation'))
Another option is to bring your own sequence of operative zones (1 for each TGU) and build the operation data from it:
from acopoweropt import system
# Intance a PowerSystem class from a configuration file where 's10` defines a system configuration
PSystem = system.PowerSystem(name='s10')
# Define a sequence of operative zones for each of the 10 TGUs
opzs = [2, 3, 1, 2, 1, 1, 3, 1, 1, 3]
# Build a configuration that represents such sequence of operative zones
operation = PSystem.get_operation(operative_zones=opzs)
# Solve the Economic Dispatch of the specific configuration:
solution = PSystem.solve(operation=operation)
# Prints total financial cost of the operation
print("Total Financial Cost: {}".format(solution.get('Ft')))
# Prints the operation with its power dispach values
print(solution.get('operation'))
An Ant Colony should seek for a global optimal solution or "the optimal source of food". The algorithm was proposed by Marco Dorigo, check Wiki for more details.
The code below initializes a PowerColony with a desired PowerSystem as the "environment" for the ants to seek their food. Once instantiated, the PowerColony immediately unleashes their ants for a first seek for solutions, therefore PowerColony.paths
and PowerColony.pheromone
can be observed.
from acopoweropt import colony, system
PSystem = system.PowerSystem(name='s15')
PColony = colony.PowerColony(n_ants=100,
pheromone_evp_rate={'worst': 0.75, 'mean': 0.25, 'best': 0.05},
power_system=PSystem)
Now a PowerColony can seek for optimal sources of food:
PColony.seek(max_iter=20, power_system=PSystem, show_progress=True)
ax = PColony.paths.groupby('iteration').distance.min().plot(y='distance')
PColony.create_pheromone_movie(duration=0.25)
See the LICENSE file for license rights and limitations (MIT).
FAQs
Ant Colony Power Systems Optimizer
We found that acopoweropt demonstrated a healthy version release cadence and project activity because the last version was released less than a year ago. It has 1 open source maintainer collaborating on the project.
Did you know?
Socket for GitHub automatically highlights issues in each pull request and monitors the health of all your open source dependencies. Discover the contents of your packages and block harmful activity before you install or update your dependencies.
Security News
Crates.io adds Trusted Publishing support, enabling secure GitHub Actions-based crate releases without long-lived API tokens.
Research
/Security News
Undocumented protestware found in 28 npm packages disrupts UI for Russian-language users visiting Russian and Belarusian domains.
Research
/Security News
North Korean threat actors deploy 67 malicious npm packages using the newly discovered XORIndex malware loader.