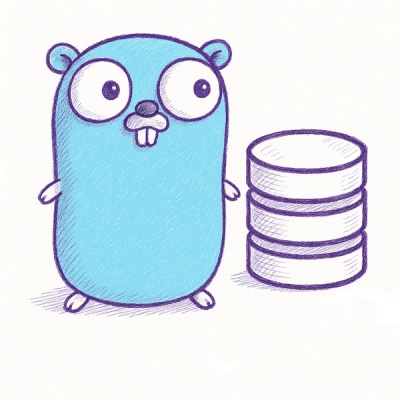
Security News
Official Go SDK for MCP in Development, Stable Release Expected in August
The official Go SDK for the Model Context Protocol is in development, with a stable, production-ready release expected by August 2025.
ADCL-Automatic-Data-Cleaning is a Python package designed to facilitate automated data cleaning, particularly leveraging deep learning techniques for preprocessing tasks essential in data science and machine learning workflows.
data_preprocessing.py
with all preprocessing functions.example_usage.ipynb
, a Jupyter notebook demonstrating how to use the preprocessing functions.To install ADCL directly from PyPI, run the following command:
pip install adcl
You can preprocess your datasets by importing functions from data_preprocessing.py
. For example:
from adcl import process_data
filepath = 'path_to_your_data.csv'
df_train, df_test, y_column_name, date_col = process_data(train_input=filepath)
Handle missing values by choosing an appropriate method from the library. An example usage for time series data:
from adcl import missing_values_handling
X_train_mis, X_test_mis = missing_values_handling(df_train=X_train, df_test=X_test, datetime_col=date_col, imputation_method='auto')
Detect Outliers by choosing an appropriate method from the library. An example usage for time series data:
from adcl import outlier_detection
X_train_out, X_test_out = outlier_detection(X_train=X_train, X_test=X_test, datetime_col=date_col
, method='auto', nu=0.05, kernel='rbf', gamma='scale'
, n_neighbors=20, contamination='auto', n_estimators=100
, encoding_dim=8, epochs=50, batch_size=32
, window_size=20, dtw_window=None)
Encode categorical variables by choosing an appropriate method from the library. An example usage for time series data:
from adcl import encode_data
X_train_enc, X_test_enc = encode_data(df_train=X_train, df_test=X_test, y_column_name,
encoding_method='label', nu=0.05, kernel='rbf', gamma='scale',
n_neighbors=20, contamination='auto', n_estimators=100,
encoding_dim=8, epochs=50, batch_size=32)
For detailed examples, refer to the notebooks in the examples/
directory. These notebooks provide comprehensive guides on utilizing the package's functionalities effectively.
Contributions are welcome! If you have suggestions for improving the library, feel free to fork the repository and submit a pull request.
This project is licensed under the MIT License - see the LICENSE file for details.
For any queries or further information, please contact steve19992@mail.ru.
By providing structured guidance on using the package and clearly explaining what each part of the package does, users of all levels can effectively integrate ADCL into their data cleaning and preprocessing workflows.
FAQs
Data preprocessing and cleaning tools for data science projects
We found that adcl demonstrated a healthy version release cadence and project activity because the last version was released less than a year ago. It has 1 open source maintainer collaborating on the project.
Did you know?
Socket for GitHub automatically highlights issues in each pull request and monitors the health of all your open source dependencies. Discover the contents of your packages and block harmful activity before you install or update your dependencies.
Security News
The official Go SDK for the Model Context Protocol is in development, with a stable, production-ready release expected by August 2025.
Security News
New research reveals that LLMs often fake understanding, passing benchmarks but failing to apply concepts or stay internally consistent.
Security News
Django has updated its security policies to reject AI-generated vulnerability reports that include fabricated or unverifiable content.