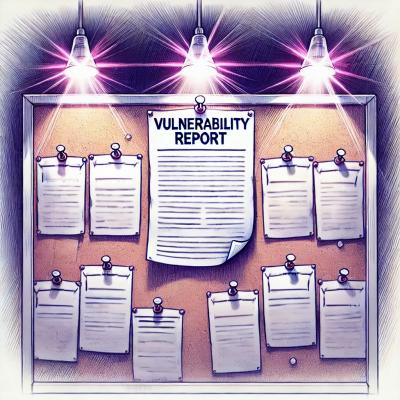
Security News
libxml2 Maintainer Ends Embargoed Vulnerability Reports, Citing Unsustainable Burden
Libxml2’s solo maintainer drops embargoed security fixes, highlighting the burden on unpaid volunteers who keep critical open source software secure.
Create a virtual environment
virtualenv venv --python=python3
Activate the virtual environment
# Macbook / Linus
source venv/bin/activate
# Windows
venv/Scripts/activate
Deactivate
deactivate
Install AreixIO package
pip install areixio
Define trading strategy:
from areixio import (BacktestBroker,CryptoDataFeed, StockDataFeed, CustomDataFeed,
create_report_folder, Strategy, BackTest, Indicator, Statistic)
from collections import defaultdict
from datetime import datetime
from dateutil.parser import parse
class TestStrategy(Strategy):
boll_window = 18
boll_dev = 3.4
cci_window = 10
atr_window = 30
sl_multiplier = 5.2
def initialize(self):
self.boll_up = defaultdict(float)
self.boll_down = defaultdict(float)
self.cci_value = defaultdict(float)
self.atr_value = defaultdict(float)
self.intra_trade_high = defaultdict(float)
self.intra_trade_low = defaultdict(float)
self.long_stop = defaultdict(float)
self.short_stop = defaultdict(float)
self.indicators = {}
for code, exchange in self.ctx.symbols:
self.indicators[code] = Indicator()
def on_order_fail(self, order):
self.error(f"Order [number {order['order_id']}] [{order['status'].name}]. Msg: {order['msg']}")
def on_order_fill(self, order):
self.info(f"({order.aio_position_id}) - {'OPEN' if order.is_open else 'CLOSE'} {order['side'].name} order [number {order['order_id']}] executed [quantity {order['quantity']}] [price ${order['price']:2f}] [Cost ${order['gross_amount']:2f}] [Commission: ${order['commission']}] [Available balance: ${self.available_balance}] [Position: #{self.ctx.get_quantity(aio_position_id = order['aio_position_id'])}] [Gross P&L: ${order['pnl']}] [Net P&L: ${order['pnl_net']}] ")
if not order['is_open']:
self.info(f"========> Trade closed, pnl: {order['pnl']}")
def on_bar(self, tick):
self.cancel_all()
for code, exchange in self.ctx.symbols:
indicator = self.indicators[code]
bar = self.ctx.get_bar_data(symbol=code, exchange=exchange)
# hist = self.ctx.get_history(symbol=code, exchange=exchange)
if bar is None:
continue
indicator.update_bar(bar=bar)
if not indicator.inited:
continue
close = bar.close
self.boll_up[code], self.boll_down[code] = indicator.boll(self.boll_window, self.boll_dev)
self.cci_value[code] = indicator.cci(self.cci_window)
self.atr_value[code] = indicator.atr(self.atr_window)
self.pos = self.ctx.get_quantity(symbol=code,exchange=exchange )
self.debug(f"pos:{self.pos}; cci_value:{self.cci_value[code]}; atr_value:{self.atr_value[code]}; boll_up:{self.boll_up[code]}; boll_down:{self.boll_down[code]}; intra_trade_high:{self.intra_trade_high[code]}; long_stop:{self.long_stop[code]}; intra_trade_low:{self.intra_trade_low[code]}; short_stop:{self.short_stop[code]}; close:{close}")
order = None
close_order = None
if not self.pos:
self.intra_trade_high[code] = bar.high
self.intra_trade_low[code] = bar.low
if self.cci_value[code] > 0:
order = self.buy(symbol=code, exchange=exchange, stop_price=self.boll_up[code], quantity= self.fixed_size[code])
elif self.cci_value[code] < 0:
order = self.sell(symbol=code, exchange=exchange, stop_price=self.boll_down[code],quantity= self.fixed_size[code])
elif self.pos > 0:
self.intra_trade_high[code] = max(self.intra_trade_high[code], bar.high)
self.intra_trade_low[code] = bar.low
self.long_stop[code] = self.intra_trade_high[code] - self.atr_value[code] * self.sl_multiplier
close_order = self.close(symbol=code, exchange=exchange, stop_price=self.long_stop[code],)
elif self.pos < 0:
self.intra_trade_high[code] = bar.high
self.intra_trade_low[code] = min(self.intra_trade_low[code], bar.low)
self.short_stop[code] = self.intra_trade_low[code] + self.atr_value[code] * self.sl_multiplier
close_order = self.close(symbol=code, exchange=exchange, stop_price=self.short_stop[code],)
if order:
self.info(f"Order for {code} [number {order['order_id']}] ({order['order_type'].name} & {order['side'].name}) created, [quantity {order['quantity']}] [price {order['price']}]")
if close_order:
self.info(f"Stop Order for {code} [number {close_order['order_id']}] ({close_order['order_type']} & {close_order['side'].name}) created, [quantity {close_order['quantity']}] [price {close_order['price']}]")
usage:
if __name__ == '__main__':
benchmark_code = 'BTC/USDT'
interval = '1h'
start_date = "2022-01-01 00:00:00"
end_date = "2022-08-22 00:00:00"
# end_date = datetime.now().strftime("%Y-%m-%d %H:%M:%S")
second_timeframe = {
'start_date': "2022-10-01 00:00:00",
'end_date': datetime.now().strftime("%Y-%m-%d %H:%M:%S"),
'interval': "1m",
}
### uncomment the following if has multiple timeframe
second_timeframe = {}
fixed_size = {
'BTCUSDT': 0.5,
'ETHUSDT': 1,
'SOLUSDT': 5,
}
codes = list(fixed_size.keys())
asset_type = 'perpetual'
exchange = 'bybit'
base = create_report_folder()
if isinstance(codes, str):
codes = [codes]
feeds = []
for code in codes:
df = CryptoDataFeed(
code=code,
exchange=exchange,
asset_type = asset_type,
start_date=start_date,
end_date=end_date,
interval=interval,
order_ascending=True,
# store_path=base
)
df.fetch_info()
### uncomment the following if has multiple timeframe
# if second_timeframe:
# hist_data = df.fetch_hist(start=parse(second_timeframe['start_date']), end=parse(second_timeframe['end_date']), interval=second_timeframe['interval'], is_store=True)
# df.update_data(hist_data)
feeds.append(df)
benchmark = CryptoDataFeed(
code=benchmark_code,
exchange=exchange,
asset_type = asset_type,
start_date=start_date,
end_date=end_date,
interval=interval,
min_volume = 0.00001,
order_ascending=True,
# store_path=base
)
### uncomment the following if has multiple timeframe
# if second_timeframe:
# hist_data = benchmark.fetch_hist(start=parse(second_timeframe['start_date']), end=parse(second_timeframe['end_date']), interval=second_timeframe['interval'], is_store=True)
# benchmark.update_data(hist_data)
broker = BacktestBroker(
balance=100_000,
short_cash=False,
slippage=0.0)
trade_history = []
statistic = Statistic()
mytest = BackTest(
feeds,
TestStrategy,
statistic=statistic,
benchmark=benchmark,
store_path=base,
broker=broker,
backtest_mode='bar',
fixed_size = fixed_size,
trade_history=trade_history
)
mytest.start()
stats = mytest.ctx.statistic.stats(interval=interval)
stats['algorithm'] = ['Bollinger Band', 'CCI', 'ATR']
print(stats)
mytest.ctx.statistic.contest_output(path=base, interval=interval, prefix=f'bt_',is_plot=True)
Result:
start 2022-01-01 00:00:00+08:00
end 2022-08-22 00:00:00+08:00
interval 1h
duration 233 days 00:00:00
trading_instruments [BTCUSDT, ETHUSDT, SOLUSDT]
base_currency USDT
benchmark BTCUSDT
beginning_balance 100000
ending_balance 119562.812570
available_balance 117927.612569
holding_values 1635.200000
capital 100000
additional_capitals {}
net_investment 27960.225000
total_net_profit 19562.812570
total_commission 14.107925
gross_profit 209249.519700
gross_loss -189686.707100
profit_factor 1.103132
return_on_capital 0.195628
return_on_initial_capital 0.195628
return_on_investment 0.699666
annualized_return 0.322989
total_return 0.195628
max_return 0.199261
min_return 0.000000
past_24hr_pnl -61.800000
past_24hr_roi -0.000517
past_24hr_apr -0.188705
number_trades 457
number_closed_trades 227
number_winning_trades 100
number_losing_trades 127
avg_daily_trades 2.840000
avg_weekly_trades 13.850000
avg_monthly_trades 57.130000
win_ratio 0.440529
loss_ratio 0.559471
gross_trades_profit 40851.052400
gross_trades_loss -19646.301700
gross_winning_trades_amount 623690.417300
gross_losing_trades_amount 1002871.039600
avg_winning_trades_pnl 408.510524
avg_losing_trades_pnl -154.695289
avg_winning_trades_amount 6236.904173
avg_losing_trades_amount 4910.948168
largest_profit_winning_trade 4735.210100
largest_loss_losing_trade -1039.239400
avg_amount_per_closed_trade 7165.469000
expected_value 93.410000
standardized_expected_value 14.980000
win_days 106
loss_days 113
max_win_in_day 394.250000
max_loss_in_day -532.521400
max_consecutive_win_days 6
max_consecutive_loss_days 6
avg_profit_per_trade($) 93.413000
avg_profit_per_trade 0.000900
trading_period 0 years 7 months 21 days 0 hours
avg_daily_pnl($) 83.960600
avg_daily_pnl 0.000781
avg_weekly_pnl($) 575.376900
avg_weekly_pnl 0.005363
avg_monthly_pnl($) 2454.108200
avg_monthly_pnl 0.022597
avg_quarterly_pnl($) 5512.129000
avg_quarterly_pnl 0.049787
avg_annualy_pnl($) None
avg_annualy_pnl None
var 180.327500
risk_score 0.190000
avg_daily_risk_score 0.210000
avg_risk_score_past_7days 0.190000
monthly_avg_risk_score {'2022-01-31 23:00:00': 0.18, '2022-02-28 23:0...
frequently_traded [{'symbol': 'BTCUSDT', 'asset_type': 'PERPETUA...
sharpe_ratio 2.728096
sortino_ratio 4.154441
annualized_volatility 0.104470
omega_ratio 1.104314
downside_risk 0.068596
information_ratio 0.018749
beta -0.048020
alpha 0.266868
calmar_ratio 8.375185
tail_ratio 1.115830
stability_of_timeseries 0.954381
max_drawdown 0.038565
max_drawdown_period (2022-05-12 14:00:00+08:00, 2022-05-30 03:00:0...
max_drawdown_duration 17 days 13:00:00
sqn 2.286010
monthly_changes {'2022-01-31 00:00:00': 0.0, '2022-02-28 00:00...
daily_changes {'2022-01-01 00:00:00': 0.0, '2022-01-02 00:00...
positions [PositionData(symbol='SOLUSDT', code='SOLUSDT'...
trades [TradeData(order_id='220106-000000000-00066', ...
pnl [{'available_balance': 100000.0, 'holding_valu...
Optimization:
if __name__ == '__main__':
benchmark_code = 'BTC/USDT'
interval = '1h'
start_date="2022-08-01 00:00:00"
end_date= datetime.now().strftime("%Y-%m-%d %H:%M:%S")
.....
mytest = BackTest(
feeds,
TestStrategy,
benchmark=benchmark,
store_path=base,
broker=broker,
exchange=exchange,
fixed_size = fixed_size,
# trade_history=trade_history
do_print=False ### in case print too much log
)
ostats = mytest.optimize(
boll_window=[13,18,22],
boll_dev=[3,3.4,3.8],
# cci_window=[8,10,12],
# atr_window=[28,30,32],
sl_multiplier=[4.8,5.2,5.6],
maximize='total_net_profit',
constraint=None,
return_heatmap=True
)
print('ostats',ostats)
Result:
Name: value, dtype: object, boll_window boll_dev sl_multiplier
13 3.000000 4.800000 636.305780
5.200000 974.743960
5.600000 984.011120
3.400000 4.800000 555.828790
5.200000 715.990480
5.600000 779.417500
3.800000 4.800000 19.314340
5.200000 564.115470
5.600000 564.979500
18 3.000000 4.800000 -156.087610
5.200000 785.291570
5.600000 867.817680
3.400000 4.800000 910.641420
5.200000 1366.349790
5.600000 1567.919300
3.800000 4.800000 237.782640
5.200000 547.324610
5.600000 813.536520
22 3.000000 4.800000 839.132020
5.200000 1274.691530
5.600000 1401.274140
3.400000 4.800000 345.991700
5.200000 966.018210
5.600000 1105.940740
3.800000 4.800000 522.481170
5.200000 1030.565530
5.600000 782.557010
Indicator requires to install ta-lib
Mac:
1.1 Install TA-LIB
brew install ta-lib
1.2 Install TA-LIB Python Wrapper
Install TA-Lib Python Wrapper via pip (or pip3):
pip install ta-lib
Linux:
1.1 Download
wget http://prdownloads.sourceforge.net/ta-lib/ta-lib-0.4.0-src.tar.gz
tar -xzf ta-lib-0.4.0-src.tar.gz
cd ta-lib/
1.2 Install TA-LIB
If the next command fails, then gcc is missing, install it by doing “apt-get install build-essential”)
sudo ./configure
sudo make
sudo make install
if configure error: cannot guess build type you must specify one
This was solved for me by specifying the --build= parameter during the ./configure step.
For arm64
./configure --build=aarch64-unknown-linux-gnu
For x86
./configure --build=x86_64-unknown-linux-gnu
1.3 Install TA-LIB Python Wrapper
Install TA-Lib Python Wrapper via pip (or pip3):
pip install ta-lib
Windows
1.1 Download
Download [ta-lib-0.4.0-msvc.zip](http://prdownloads.sourceforge.net/ta-lib/ta-lib-0.4.0-msvc.zip)
1.2 Unzip
unzip to C:\ta-lib
1.3 Install TA-LIB Python Wrapper
Install TA-Lib Python Wrapper via pip (or pip3):
pip install ta-lib
FAQs
Areix IO Backtesting Framework
We found that areixio demonstrated a healthy version release cadence and project activity because the last version was released less than a year ago. It has 1 open source maintainer collaborating on the project.
Did you know?
Socket for GitHub automatically highlights issues in each pull request and monitors the health of all your open source dependencies. Discover the contents of your packages and block harmful activity before you install or update your dependencies.
Security News
Libxml2’s solo maintainer drops embargoed security fixes, highlighting the burden on unpaid volunteers who keep critical open source software secure.
Research
Security News
Socket researchers uncover how browser extensions in trusted stores are used to hijack sessions, redirect traffic, and manipulate user behavior.
Research
Security News
An in-depth analysis of credential stealers, crypto drainers, cryptojackers, and clipboard hijackers abusing open source package registries to compromise Web3 development environments.