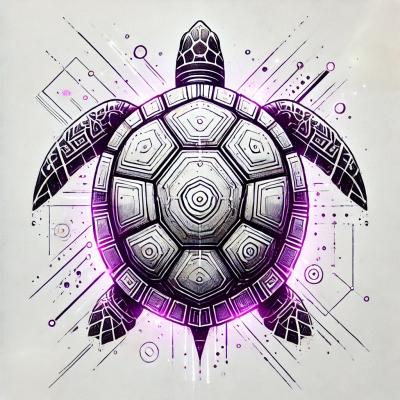
Security Fundamentals
Turtles, Clams, and Cyber Threat Actors: Shell Usage
The Socket Threat Research Team uncovers how threat actors weaponize shell techniques across npm, PyPI, and Go ecosystems to maintain persistence and exfiltrate data.
Argolid
is a Python package for working with volumetric data and generating multi-resolution pyramids. It provides classes for reading and writing pixel data, generating Zarr arrays, and creating multi-resolution pyramids.
You can install Argolid
using pip (pip install argolid
) or using conda
(conda install -c conda-forge argolid
).
Argolid
uses Tensorstore
for reading and writing pixel data. So Tensorstore
build requirements are needed to be satisfied.
For Linux, these are the requirements:
GCC
10 or laterClang
8 or laterPython
3.8 or laterCMake
3.24 or laterPerl
, for building libaom from source (default). Must be in PATH
. Not required if -DTENSORSTORE_USE_SYSTEM_LIBAOM=ON
is specified.NASM
, for building libjpeg-turbo, libaom, and dav1d from source (default). Must be in PATH
.Not required if -DTENSORSTORE_USE_SYSTEM_{JPEG,LIBAOM,DAV1D}=ON
is specified.GNU Patch
or equivalent. Must be in PATH
.Here is an example of building and installing Argolid
in a Python virtual environment.
python -m virtualenv venv
source venv/bin/activate
pip install cmake
git clone https://github.com/sameeul/argolid.git
cd argolid
mkdir build_deps
cd build_deps
sh ../ci_utils/install_prereq_linux.sh
cd ../
export ARGOLID_DER_DIR=./build_deps/local_install
python setup.py install
Argolid can generate 2D Pyramids from a single image or an image collection with a stitching vector provided. It can generate three different kind of pyramids:
Currently, three downsampling methods (mean
, mode_max
and mode_min
) are supported. A dictionary with channel id (integer) as key and downsampling method as value can be passed to specify downsampling method for specific channel. If a channel does not exist as a key in the
dictionary, mean
will be used as the default downsampling method
Here is an example of generating a pyramid from a single image.
from argolid import PyramidGenerartor
input_file = "/home/samee/axle/data/test_image.ome.tif"
output_dir = "/home/samee/axle/data/test_image_ome_zarr"
min_dim = 1024
pyr_gen = PyramidGenerartor()
pyr_gen.generate_from_single_image(input_file, output_dir, min_dim, "NG_Zarr", {0:"mode_max"})
Here is an example of generating a pyramid from a collection of images and a stitching vector.
from argolid import PyramidGenerartor
input_dir = "/home/samee/axle/data/intensity1"
file_pattern = "x{x:d}_y{y:d}_c{c:d}.ome.tiff"
output_dir = "/home/samee/axle/data/test_assembly_out"
image_name = "test_image"
min_dim = 1024
pyr_gen = PyramidGenerartor()
pyr_gen.generate_from_image_collection(input_dir, file_pattern, image_name,
output_dir, min_dim, "Viv", {1:"mean"})
Argolid provides two main classes for working with volumetric data and generating multi-resolution pyramids:
The VolumeGenerator
class is used to create Zarr arrays from image stacks. It handles reading image files, grouping them based on specified criteria, and writing the data into a Zarr array.
Here's an example of how to use VolumeGenerator
:
from argolid import VolumeGenerator
source_dir = "/path/to/image/files"
group_by = "z" # Group images by z-axis
file_pattern = "image_{z:d}.tif"
out_dir = "/path/to/output"
image_name = "my_volume"
volume_gen = VolumeGenerator(source_dir, group_by, file_pattern, out_dir, image_name)
volume_gen.generate_volume()
Here is an example of generating a 3D pyramid from a Zarr array:
from argolid import PyramidGenerator3D
zarr_loc_dir = "/path/to/zarr/array"
base_scale_key = 0
num_levels = 5
pyramid_gen = PyramidGenerator3D(zarr_loc_dir, base_scale_key)
pyramid_gen.generate_pyramid(num_levels)
FAQs
Pyramid Generator For OMETiff
We found that argolid demonstrated a healthy version release cadence and project activity because the last version was released less than a year ago. It has 1 open source maintainer collaborating on the project.
Did you know?
Socket for GitHub automatically highlights issues in each pull request and monitors the health of all your open source dependencies. Discover the contents of your packages and block harmful activity before you install or update your dependencies.
Security Fundamentals
The Socket Threat Research Team uncovers how threat actors weaponize shell techniques across npm, PyPI, and Go ecosystems to maintain persistence and exfiltrate data.
Security News
At VulnCon 2025, NIST scrapped its NVD consortium plans, admitted it can't keep up with CVEs, and outlined automation efforts amid a mounting backlog.
Product
We redesigned our GitHub PR comments to deliver clear, actionable security insights without adding noise to your workflow.