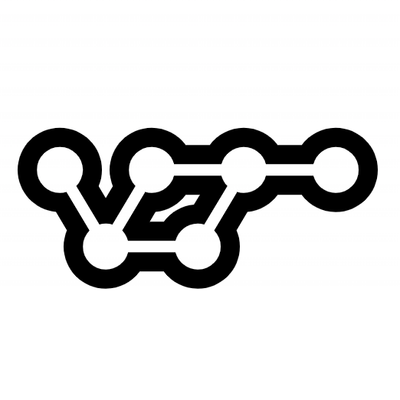
Security News
vlt Launches "reproduce": A New Tool Challenging the Limits of Package Provenance
vlt's new "reproduce" tool verifies npm packages against their source code, outperforming traditional provenance adoption in the JavaScript ecosystem.
The Aurelio Platform SDK. API references
To install the Aurelio SDK, use pip or poetry:
pip install aurelio-sdk
The SDK requires an API key for authentication. Get key from Aurelio Platform. Set your API key as an environment variable:
export AURELIO_API_KEY=your_api_key_here
See examples for more details.
from aurelio_sdk import AurelioClient
import os
client = AurelioClient(api_key=os.environ["AURELIO_API_KEY"])
or use asynchronous client:
from aurelio_sdk import AsyncAurelioClient
client = AsyncAurelioClient(api_key="your_api_key_here")
from aurelio_sdk import ChunkingOptions, ChunkResponse
# All options are optional with default values
chunking_options = ChunkingOptions(
chunker_type="semantic", max_chunk_length=400, window_size=5
)
response: ChunkResponse = client.chunk(
content="Your text here to be chunked", processing_options=chunking_options
)
from aurelio_sdk import ExtractResponse
# From a local file
file_path = "path/to/your/file.pdf"
response_pdf_file: ExtractResponse = client.extract_file(
file_path=file_path, quality="low", chunk=True, wait=-1
)
from aurelio_sdk import ExtractResponse
# From a local file
file_path = "path/to/your/file.mp4"
response_video_file: ExtractResponse = client.extract_file(
file_path=file_path, quality="low", chunk=True, wait=-1
)
from aurelio_sdk import ExtractResponse
# From URL
url = "https://arxiv.org/pdf/2408.15291"
response_pdf_url: ExtractResponse = client.extract_url(
url=url, quality="low", chunk=True, wait=-1
)
from aurelio_sdk import ExtractResponse
# From URL
url = "https://storage.googleapis.com/gtv-videos-bucket/sample/ForBiggerMeltdowns.mp4"
response_video_url: ExtractResponse = client.extract_url(
url=url, quality="low", chunk=True, wait=-1
)
# Set wait time for large files with `high` quality
# Wait time is set to 10 seconds
response_pdf_url: ExtractResponse = client.extract_url(
url="https://arxiv.org/pdf/2408.15291", quality="high", chunk=True, wait=10
)
# Get document status and response
document_response: ExtractResponse = client.get_document(
document_id=response_pdf_file.document.id
)
print("Status:", document_response.status)
# Use a pre-built function, which helps to avoid long hanging requests (Recommended)
document_response = client.wait_for(
document_id=response_pdf_file.document.id, wait=300
)
from aurelio_sdk import EmbeddingResponse
response: EmbeddingResponse = client.embedding(
input="Your text here to be embedded",
model="bm25")
# Or with a list of texts
response: EmbeddingResponse = client.embedding(
input=["Your text here to be embedded", "Your text here to be embedded"]
)
The ExtractResponse
object contains the following key information:
status
: The current status of the extraction taskusage
: Information about token usage, pages processed, and processing timemessage
: Any relevant messages about the extraction processdocument
: The extracted document information, including its IDchunks
: The extracted text, divided into chunks if chunking was enabledThe EmbeddingResponse
object contains the following key information:
message
: Any relevant messages about the embedding processmodel
: The model name used for embeddingusage
: Information about token usage, pages processed, and processing timedata
: The embedded documentsquality
parameter based on your needs. "low" is faster but less accurate, while "high" is slower but more accurate.FAQs
Aurelio Platform SDK
We found that aurelio-sdk demonstrated a healthy version release cadence and project activity because the last version was released less than a year ago. It has 1 open source maintainer collaborating on the project.
Did you know?
Socket for GitHub automatically highlights issues in each pull request and monitors the health of all your open source dependencies. Discover the contents of your packages and block harmful activity before you install or update your dependencies.
Security News
vlt's new "reproduce" tool verifies npm packages against their source code, outperforming traditional provenance adoption in the JavaScript ecosystem.
Research
Security News
Socket researchers uncovered a malicious PyPI package exploiting Deezer’s API to enable coordinated music piracy through API abuse and C2 server control.
Research
The Socket Research Team discovered a malicious npm package, '@ton-wallet/create', stealing cryptocurrency wallet keys from developers and users in the TON ecosystem.