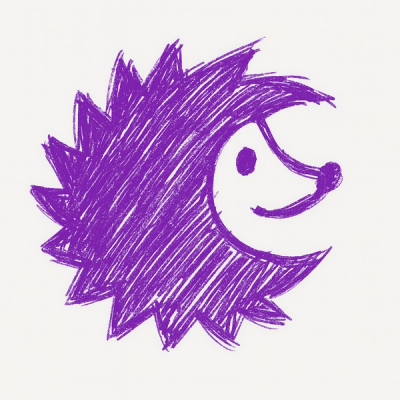
Security News
Browserslist-rs Gets Major Refactor, Cutting Binary Size by Over 1MB
Browserslist-rs now uses static data to reduce binary size by over 1MB, improving memory use and performance for Rust-based frontend tools.
This is a RAG chatbot application based on AWS components and designed to be optimized for a serverless architecture and cost optimized for a high-volume mobile application use case. The AWS Services being used are:
The frameworks I created here abstract out a variety of components to enable easily testing variations. This makes it easier to tune the implementation based on the content being use in my applications. The goal is to play with different LLM's, different embeddings, parameters like temperature, top_p and more. Content loading also needed to be highly refined allowing me to control each source easily.
This library is meant more to provide a complete working set of examples and less to be an out-of-the-box library to use as-is.
Because this framework imports so many variants of embeddings, LLM models, etc. it is not suitable for a direct deployment into something like AWS Lambda... it is a :pig:.
Serious package bloat.
This project uses Poetry to manage dependencies. You can install it with the following command:
pip install poetry
Then you can install the dependencies with the following command:
poetry install
Alternatively, you can install the package from PyPi with the following command:
pip install aws-rag-bot
Where the PyPi package is available at https://pypi.org/project/aws-rag-bot/
This is a very simple, high-level example. Check out the rag_bot_code_samples.ipynb for a more. First step is to have content in your vector database.
from open_search_vector_db.aws_opensearch_vector_database import OpenSearchVectorDBLoader
content_sources = [{"name": "Internal KB Docs", "type": "PDF", "location": "kb-docs"}]
vectordb_loader = OpenSearchVectorDBLoader(os_endpoint=my_open_search_endpoint,
index_name=my_index_name,
data_sources=content_sources)
vectordb_loader.load()
Then you can start asking questions of it
from rag_chatbot import RagChatbot, LlmModelTypes
from prompt_library import DefaultPrompts
chatbot = RagChatbot(my_open_search_endpoint,
model_key=LlmModelTypes.BEDROCK_TITAN_EXPRESS,
prompt_model=NasaSpokespersonPrompts)
chat_history = []
question = "What...?" # Ask a question related to the content you loaded
response = chatbot.ask_question(question, chat_history, verbose=True)
print(response)
You can use the tests/provision_test_index.py to create a test index in OpenSearch. The content loaded supports the test cases in this project
A very simple command line client program has been created as an example and tool to test. It is called chatbot_client.py.
It is a simple command line program that will ask a question and then print the response while retaining the chat history for context.
python chatbot_client.py my-opensource-domain-name
There are two test modules in the tests folder used to run through search and the RAG bot to make sure everything is working as well as provide some additional samples of how to use the framework.
Ragas is one of a variety of evaluation tools for RAG applications.
It can be used to evaluate both the retrieval and generation aspects of the RAG bot.
With an evaluation tool you can then use this project's features to vary whatever aspects you need
and compare the results to make decisions and tune.
A simple example of this can be found in the tests folder.
Vector Database: May references show using Chroma and FAISS, but I needed a solution that worked well in a Lambda serverless environment.
Ideally it would be at AWS keeping my stack uniform.
Ultimately I chose OpenSearch because of cost, support by LangChain and a serverless version I plan to evaluate
Vector Database Loader: LangChain has a great library of DataLoaders for loading data into OpenSearch. I wanted an effective way to scrape a website with help from this article chose to use Selenium.
I also used the directory loader and plan to implement cloud based directory loaders in the future. Primarily S3 and Google Drive.
Langsmith Logging and Debugging: I used LangSmith for logging and debugging. It is a great tool for this purpose.
FAQs
Unknown package
We found that aws-rag-bot demonstrated a healthy version release cadence and project activity because the last version was released less than a year ago. It has 1 open source maintainer collaborating on the project.
Did you know?
Socket for GitHub automatically highlights issues in each pull request and monitors the health of all your open source dependencies. Discover the contents of your packages and block harmful activity before you install or update your dependencies.
Security News
Browserslist-rs now uses static data to reduce binary size by over 1MB, improving memory use and performance for Rust-based frontend tools.
Research
Security News
Eight new malicious Firefox extensions impersonate games, steal OAuth tokens, hijack sessions, and exploit browser permissions to spy on users.
Security News
The official Go SDK for the Model Context Protocol is in development, with a stable, production-ready release expected by August 2025.