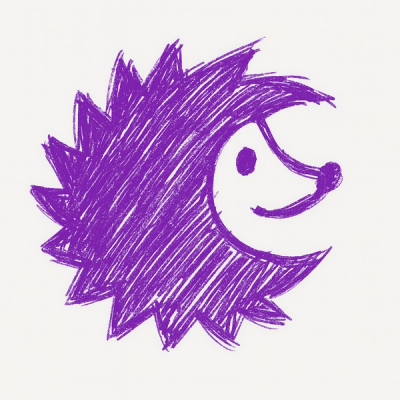
Security News
Browserslist-rs Gets Major Refactor, Cutting Binary Size by Over 1MB
Browserslist-rs now uses static data to reduce binary size by over 1MB, improving memory use and performance for Rust-based frontend tools.
Baycomp is a library for Bayesian comparison of classifiers.
Functions compare two classifiers on one or on multiple data sets. They
compute three probabilities: the probability that the first classifier has
higher scores than the second, the probability that differences are within
the region of practical equivalence (rope), or that the second classifier
has higher scores. We will refer to this probabilities as p_left
, p_rope
and p_right
. If the argument rope
is omitted (or set to zero), functions
return only p_left
and p_right
.
The region of practical equivalence (rope) is specified by the caller and should correspond to what is "equivalent" in practice; for instance, classification accuracies that differ by less than 0.5 may be called equivalent.
Similarly, whether higher scores are better or worse depends upon the type of the score.
The library can also plot the posterior distributions.
The library can be used in three ways.
Two shortcut functions can be used for comparison on single
and on multiple data sets. If nbc
and j48
contain a list of average
classification accuracies of naive Bayesian classifier and J48 on a
collection of data sets, we can call
>>> two_on_multiple(nbc, j48, rope=1)
(0.23124, 0.00666, 0.7621)
(Actual results may differ due to Monte Carlo sampling.)
With some additional arguments, the function can also plot the posterior distribution from which these probabilities came.
Tests are packed into test classes. The above call is equivalent to
>>> SignedRankTest.probs(nbc, j48, rope=1)
(0.23124, 0.00666, 0.7621)
and to get a plot, we call
>>> SignedRankTest.plot(nbc, j48, rope=1, names=("nbc", "j48"))
To switch to another test, use another class::
>>> SignTest.probs(nbc, j48, rope=1)
(0.26508, 0.13274, 0.60218)
Finally, we can construct and query sampled posterior distributions.
>>> posterior = SignedRankTest(nbc, j48, rope=0.5)
>>> posterior.probs()
(0.23124, 0.00666, 0.7621)
>>> posterior.plot(names=("nbc", "j48"))
Install from PyPI:
pip install baycomp
User documentation is available on https://baycomp.readthedocs.io/.
A detailed description of the implemented methods is available in Time for a Change: a Tutorial for Comparing Multiple Classifiers Through Bayesian Analysis, Alessio Benavoli, Giorgio Corani, Janez Demšar, Marco Zaffalon. Journal of Machine Learning Research, 18 (2017) 1-36.
FAQs
Bayesian tests for comparison of classifiers
We found that baycomp demonstrated a healthy version release cadence and project activity because the last version was released less than a year ago. It has 1 open source maintainer collaborating on the project.
Did you know?
Socket for GitHub automatically highlights issues in each pull request and monitors the health of all your open source dependencies. Discover the contents of your packages and block harmful activity before you install or update your dependencies.
Security News
Browserslist-rs now uses static data to reduce binary size by over 1MB, improving memory use and performance for Rust-based frontend tools.
Research
Security News
Eight new malicious Firefox extensions impersonate games, steal OAuth tokens, hijack sessions, and exploit browser permissions to spy on users.
Security News
The official Go SDK for the Model Context Protocol is in development, with a stable, production-ready release expected by August 2025.