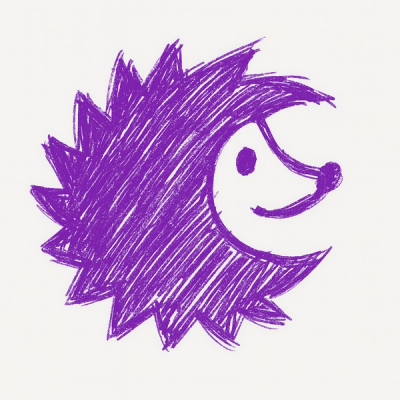
Security News
Browserslist-rs Gets Major Refactor, Cutting Binary Size by Over 1MB
Browserslist-rs now uses static data to reduce binary size by over 1MB, improving memory use and performance for Rust-based frontend tools.
A Python
implementation of the Bayesian Optimization (BO) algorithm working on decision spaces composed of either real, integer, catergorical variables, or a mixture thereof.
Underpinned by surrogate models, BO iteratively proposes candidate solutions using the so-called acquisition function which balances exploration with exploitation, and updates the surrogate model with newly observed objective values. This algorithm is designed to optimize expensive black-box problems efficiently.
You could either install the stable version on pypi
:
pip install bayes-optim
Or, take the lastest version from github:
git clone https://github.com/wangronin/Bayesian-Optimization.git
cd Bayesian-Optimization && python setup.py install --user
For real-valued search variables, the simplest usage is via the fmin
function:
from bayes_optim import fmin
def f(x):
return sum(x ** 2)
minimum = fmin(f, [-5] * 2, [5] * 2, max_FEs=30, seed=42)
And you could also have much finer control over most ingredients of BO, e.g., the surrogate model and acquisition functions. Please see the example below:
from bayes_optim import BO, RealSpace
from bayes_optim.Surrogate import GaussianProcess
dim = 5
space = RealSpace([-5, 5]) * dim # create the search space
# hyperparameters of the GPR model
thetaL = 1e-10 * (ub - lb) * np.ones(dim)
thetaU = 10 * (ub - lb) * np.ones(dim)
model = GaussianProcess( # create the GPR model
thetaL=thetaL, thetaU=thetaU
)
opt = BO(
search_space=space,
obj_fun=fitness,
model=model,
DoE_size=5, # number of initial sample points
max_FEs=50, # maximal function evaluation
verbose=True
)
opt.run()
For more detailed usage and exmaples, please check out our wiki page.
This implementation differs from alternative packages/libraries in the following features:
bayes-optim/SearchSpace.py
: implementation of the search/decision space.bayes-optim/base.py
: the base class of Bayesian Optimization.bayes-optim/AcquisitionFunction.py
: the implemetation of acquisition functions (see below for the list of implemented ones).bayes-optim/Surrogate
: we implemented the Gaussian Process Regression (GPR) and Random Forest (RF).bayes-optim/BayesOpt.py
contains several BO variants:
BO
: noiseless + sequentialParallelBO
: noiseless + parallel (a.k.a. batch-sequential)AnnealingBO
: noiseless + parallel + annealling [WEB18]SelfAdaptiveBO
: noiseless + parallel + self-adaptive [WEB19]NoisyBO
: noisy + parallelbayes-optim/Extension.py
is meant to include the lastest developments that are not extensively tested:
PCABO
: noiseless + parallel + PCA-assisted dimensionality reduction [RaponiWBBD20] [Under Construction]MultiAcquisitionBO
: noiseless + parallelization with multiple different acquisition functions [Under Construction]The following infill-criteria are implemented in the library:
For sequential working mode, Expected Improvement is used by default. For parallelization mode, MGFI is enabled by default.
The meta (surrogate)-model used in Bayesian optimization. The basic requirement for such a model is to provide the uncertainty quantification (either empirical or theorerical) for the prediction. To easily handle the categorical data, random forest model is used by default. The implementation here is based the one in scikit-learn, with modifications on uncertainty quantification.
Bayesian Optimization [Moc74, JSW98] (BO) is a sequential optimization strategy originally proposed to solve the single-objective black-box optimiza-tion problem that is costly to evaluate. Here, we shall restrict our discussion to the single-objective case. BO typically starts with sampling an initial design of experiment (DoE) of size, X={x1,x2,...,xn}, which is usually generated by simple random sampling, Latin Hypercube Sampling [SWN03], or the more sophisticated low-discrepancy sequence [Nie88] (e.g., Sobol sequences). Taking the initial DoE X and its corresponding objective value, Y={f(x1), f(x2),..., f(xn)} ⊆ ℝ, we proceed to construct a statistical model M describing the probability distribution of the objective function conditioned onthe initial evidence, namely Pr(f|X,Y). In most application scenarios of BO, there is a lack of a priori knowledge about f and therefore nonparametric models (e.g., Gaussian process regression or random forest) are commonly chosen for M, which gives rise to a predictor f'(x) for all x ∈ X and an uncertainty quantification s'(x) that estimates, for instance, the mean squared error of the predic-tion E(f'(x)−f(x))2. Based on f' and s', promising points can be identified via the so-called acquisition function which balances exploitation with exploration of the optimization process.
FAQs
A Bayesian Optimization Library
We found that bayes-optim demonstrated a healthy version release cadence and project activity because the last version was released less than a year ago. It has 1 open source maintainer collaborating on the project.
Did you know?
Socket for GitHub automatically highlights issues in each pull request and monitors the health of all your open source dependencies. Discover the contents of your packages and block harmful activity before you install or update your dependencies.
Security News
Browserslist-rs now uses static data to reduce binary size by over 1MB, improving memory use and performance for Rust-based frontend tools.
Research
Security News
Eight new malicious Firefox extensions impersonate games, steal OAuth tokens, hijack sessions, and exploit browser permissions to spy on users.
Security News
The official Go SDK for the Model Context Protocol is in development, with a stable, production-ready release expected by August 2025.