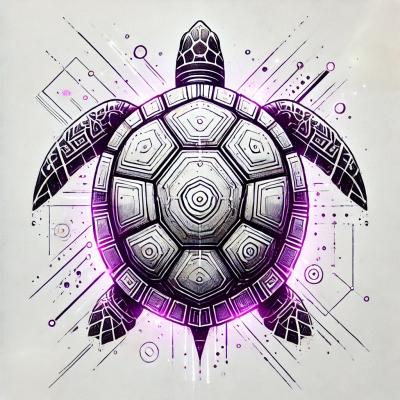
Security Fundamentals
Turtles, Clams, and Cyber Threat Actors: Shell Usage
The Socket Threat Research Team uncovers how threat actors weaponize shell techniques across npm, PyPI, and Go ecosystems to maintain persistence and exfiltrate data.
Implements binscatter methods, including partition selection, point estimation, pointwise and uniform inference methods, and graphical procedures.
Binscatter provides a flexible, yet parsimonious way of visualizing and summarizing large data sets and has been a popular methodology in applied microeconomics and other social sciences. The binsreg
package provides tools for statistical analysis using the binscatter methods developed in Cattaneo, Crump, Farrell and Feng (2024a), Cattaneo, Crump, Farrell and Feng (2024b) and Cattaneo, Crump, Farrell and Feng (2024c). binsreg
implements binscatter least squares regression with robust inference and plots, including curve estimation, pointwise confidence intervals and uniform confidence band. binsqreg
implements binscatter quantile regression with robust inference and plots, including curve estimation, pointwise confidence intervals and uniform conf idence band. binsglm
implements binscatter generalized linear regression with robust inference and plots, including curve estimation, pointwise confidence intervals and uniform confidence band. binstest
implements binscatter-based hypothesis testing procedures for parametric specifications of and shape restrictions on the unknown function of interest. binspwc
implements hypothesis testing procedures for pairwise group comparison of binscatter estimators. binsregselect
implements data-driven number of bins selectors for binscatter implementation using either quantile-spaced or evenly-spaced binning/partitioning. All the commands allow for covariate adjustment, smoothness restrictions, and clustering, among other features.
Matias D. Cattaneo (cattaneo@princeton.edu)
Richard K. Crump (richard.crump@ny.frb.org)
Max H. Farrell (maxhfarrell@ucsb.edu)
Yingjie Feng (fengyingjiepku@gmail.com)
Ricardo Masini (rmasini@princeton.edu)
https://nppackages.github.io/binsreg/
This package was first released in Winter 2019, and had one major upgrade in Summer 2021.
Summer 2021 new features include: (i) generalized linear models (logit, Probit, etc.) binscatter; (ii) quantile regression binscatter; (iii) new generic specification and shape restriction hypothesis testing function (now including Lp metrics); (iv) multi-group comparison of binscatter estimators; (v) generic point evaluation of covariate-adjusted binscatter; (vi) speed improvements and optimization. A complete list of upgrades can be found here.
To install/update use pip
pip install binsreg
from binsreg import binsregselect, binsreg, binsqreg, binsglm, binstest, binspwc
For overviews and introductions, see NP Packages website.
Cattaneo, Crump, Farrell and Feng (2024a): On Binscatter.
American Economic Review 114(5): 1488-1514.
Cattaneo, Crump, Farrell and Feng (2024b): Nonlinear Binscatter Methods.
Working paper.
FAQs
Implements binscatter methods, including partition selection, point estimation, pointwise and uniform inference methods, and graphical procedures.
We found that binsreg demonstrated a healthy version release cadence and project activity because the last version was released less than a year ago. It has 3 open source maintainers collaborating on the project.
Did you know?
Socket for GitHub automatically highlights issues in each pull request and monitors the health of all your open source dependencies. Discover the contents of your packages and block harmful activity before you install or update your dependencies.
Security Fundamentals
The Socket Threat Research Team uncovers how threat actors weaponize shell techniques across npm, PyPI, and Go ecosystems to maintain persistence and exfiltrate data.
Security News
At VulnCon 2025, NIST scrapped its NVD consortium plans, admitted it can't keep up with CVEs, and outlined automation efforts amid a mounting backlog.
Product
We redesigned our GitHub PR comments to deliver clear, actionable security insights without adding noise to your workflow.