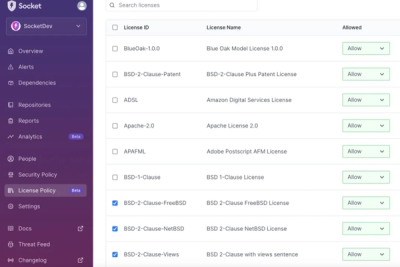
Product
Introducing License Enforcement in Socket
Ensure open-source compliance with Socket’s License Enforcement Beta. Set up your License Policy and secure your software!
Filter for improving compression of typed binary data.
Bitshuffle is an algorithm that rearranges typed, binary data for improving compression, as well as a python/C package that implements this algorithm within the Numpy framework.
The library can be used along side HDF5 to compress and decompress datasets and
is integrated through the dynamically loaded filters
_ framework. Bitshuffle
is HDF5 filter number 32008
.
Algorithmically, Bitshuffle is closely related to HDF5's Shuffle filter
_
except it operates at the bit level instead of the byte level. Arranging a
typed data array in to a matrix with the elements as the rows and the bits
within the elements as the columns, Bitshuffle "transposes" the matrix,
such that all the least-significant-bits are in a row, etc. This transpose
is performed within blocks of data roughly 8 kB long [1]_.
This does not in itself compress data, only rearranges it for more efficient compression. To perform the actual compression you will need a compression library. Bitshuffle has been designed to be well matched to Marc Lehmann's LZF_ as well as LZ4_ and ZSTD_. Note that because Bitshuffle modifies the data at the bit level, sophisticated entropy reducing compression libraries such as GZIP and BZIP are unlikely to achieve significantly better compression than simpler and faster duplicate-string-elimination algorithms such as LZF, LZ4 and ZSTD. Bitshuffle thus includes routines (and HDF5 filter options) to apply LZ4 and ZSTD compression to each block after shuffling [2]_.
The Bitshuffle algorithm relies on neighbouring elements of a dataset being highly correlated to improve data compression. Any correlations that span at least 24 elements of the dataset may be exploited to improve compression.
Bitshuffle was designed with performance in mind. On most machines the time required for Bitshuffle+LZ4 is insignificant compared to the time required to read or write the compressed data to disk. Because it is able to exploit the SSE and AVX instruction sets present on modern Intel and AMD processors, on these machines compression is only marginally slower than an out-of-cache memory copy. On modern x86 processors you can expect Bitshuffle to have a throughput of roughly 1 byte per clock cycle, and on the Haswell generation of Intel processors (2013) and later, you can expect up to 2 bytes per clock cycle. In addition, Bitshuffle is parallelized using OpenMP.
As a bonus, Bitshuffle ships with a dynamically loaded version of
h5py
's LZF compression filter, such that the filter can be transparently
used outside of python and in command line utilities such as h5dump
.
.. [1] Chosen to fit comfortably within L1 cache as well as be well matched window of the LZF compression library.
.. [2] Over applying bitshuffle to the full dataset then applying LZ4/ZSTD compression, this has the tremendous advantage that the block is already in the L1 cache.
.. _dynamically loaded filters
: https://docs.hdfgroup.org/hdf5/rfc/HDF5DynamicallyLoadedFilters.pdf
.. _Shuffle filter
: http://www.hdfgroup.org/HDF5/doc_resource/H5Shuffle_Perf.pdf
.. _LZF: http://oldhome.schmorp.de/marc/liblzf.html
.. _LZ4: https://code.google.com/p/lz4/
.. _ZSTD: https://github.com/facebook/zstd
Bitshuffle might be right for your application if:
Comparing Bitshuffle to other compression algorithms and HDF5 filters:
.. _MAFISC: https://wr.informatik.uni-hamburg.de/research/projects/icomex/mafisc
In most cases bitshuffle can be installed by pip
::
pip install bitshuffle
On Linux and macOS x86_64 platforms binary wheels are available, on other platforms a
source build will be performed. The binary wheels are built with AVX2 support and will
only run processors that support these instructions (most processors from 2015 onwards,
i.e. Intel Haswell, AMD Excavator and later). On an unsupported processor these builds
of bitshuffle will crash with SIGILL
. To run on unsupported x86_64 processors, or
target newer instructions such as AVX512, you should perform a build from source.
This can be forced by giving pip the --no-binary=bitshuffle
option.
Source installation requires python 2.7+ or 3.3+, HDF5 1.8.4 or later, HDF5 for python (h5py), Numpy and Cython. Bitshuffle is linked against HDF5. To use the dynamically loaded HDF5 filter requires HDF5 1.8.11 or later.
For total control, bitshuffle can be built using python setup.py
. If ZSTD support is
to be enabled the ZSTD repo needs to pulled into bitshuffle before installation with::
git submodule update --init
To build and install bitshuffle::
python setup.py install [--h5plugin [--h5plugin-dir=spam] --zstd]
To get finer control of installation options, including whether to compile with OpenMP
multi-threading and the target microarchitecture copy the setup.cfg.example
to
setup.cfg
and edit the values therein.
If using the dynamically loaded HDF5 filter (which gives you access to the
Bitshuffle and LZF filters outside of python), set the environment variable
HDF5_PLUGIN_PATH
to the value of --h5plugin-dir
or use HDF5's default
search location of /usr/local/hdf5/lib/plugin
.
ZSTD support is enabled with --zstd
.
If you get an error about missing source files when building the extensions, try upgrading setuptools. There is a weird bug where setuptools prior to 0.7 doesn't work properly with Cython in some cases.
.. _source: https://docs.h5py.org/en/latest/build.html#source-installation
The bitshuffle
module contains routines for shuffling and unshuffling
Numpy arrays.
If installed with the dynamically loaded filter plugins, Bitshuffle can be used
in conjunction with HDF5 both inside and outside of python, in the same way as
any other filter; simply by specifying the filter number 32008
. Otherwise
the filter will be available only within python and only after importing
bitshuffle.h5
. Reading Bitshuffle encoded datasets will be transparent.
The filter can be added to new datasets either through the h5py
low level
interface or through the convenience functions provided in
bitshuffle.h5
. See the docstrings and unit tests for examples. For h5py
version 2.5.0 and later Bitshuffle can be added to new datasets through the
high level interface, as in the example below.
The compression algorithm can be configured using the filter_opts
in
bitshuffle.h5.create_dataset()
. LZ4 is chosen with:
(BLOCK_SIZE, h5.H5_COMPRESS_LZ4)
and ZSTD with:
(BLOCK_SIZE, h5.H5_COMPRESS_ZSTD, COMP_LVL)
. See test_h5filter.py
for an example.
::
import h5py
import numpy
import bitshuffle.h5
print(h5py.__version__) # >= '2.5.0'
f = h5py.File(filename, "w")
# block_size = 0 let Bitshuffle choose its value
block_size = 0
dataset = f.create_dataset(
"data",
(100, 100, 100),
compression=bitshuffle.h5.H5FILTER,
compression_opts=(block_size, bitshuffle.h5.H5_COMPRESS_LZ4),
dtype='float32',
)
# create some random data
array = numpy.random.rand(100, 100, 100)
array = array.astype('float32')
dataset[:] = array
f.close()
If you wish to use Bitshuffle in your C program and would prefer not to use the
HDF5 dynamically loaded filter, the C library in the src/
directory is
self-contained and complete.
You can use Bitshuffle even in Java and the routines for shuffling and unshuffling
are ported into snappy-java
_. To use the routines, you need to add the following
dependency to your pom.xml::
<dependency>
<groupId>org.xerial.snappy</groupId>
<artifactId>snappy-java</artifactId>
<version>1.1.3-M1</version>
</dependency>
First, import org.xerial.snapy.BitShuffle in your Java code::
import org.xerial.snappy.BitShuffle;
Then, you use them like this::
int[] data = new int[] {1, 3, 34, 43, 34};
byte[] shuffledData = BitShuffle.bitShuffle(data);
int[] result = BitShuffle.bitUnShuffleIntArray(shuffledData);
.. _snappy-java
: https://github.com/xerial/snappy-java
If you wish to open HDF5 files compressed with bitshuffle in your Rust program, there is a Rust binding
_ for it.
In your Cargo.toml::
[dependencies]
...
hdf5-bitshuffle = "0.9"
...
To register the plugin in your code::
use hdf5_bitshuffle::register_bitshuffle_plugin;
fn main() {
register_bitshuffle_plugin();
}
.. _Rust binding
: https://docs.rs/hdf5-bitshuffle/latest/hdf5_bitshuffle/
The conda package can be build via::
conda build conda-recipe
Here are a few tips to help you get the most out of Bitshuffle:
Bitshuffle was initially described in https://doi.org/10.1016/j.ascom.2015.07.002, pre-print available at https://arxiv.org/abs/1503.00638.
FAQs
Bitshuffle filter for improving typed data compression.
We found that bitshuffle demonstrated a healthy version release cadence and project activity because the last version was released less than a year ago. It has 1 open source maintainer collaborating on the project.
Did you know?
Socket for GitHub automatically highlights issues in each pull request and monitors the health of all your open source dependencies. Discover the contents of your packages and block harmful activity before you install or update your dependencies.
Product
Ensure open-source compliance with Socket’s License Enforcement Beta. Set up your License Policy and secure your software!
Product
We're launching a new set of license analysis and compliance features for analyzing, managing, and complying with licenses across a range of supported languages and ecosystems.
Product
We're excited to introduce Socket Optimize, a powerful CLI command to secure open source dependencies with tested, optimized package overrides.