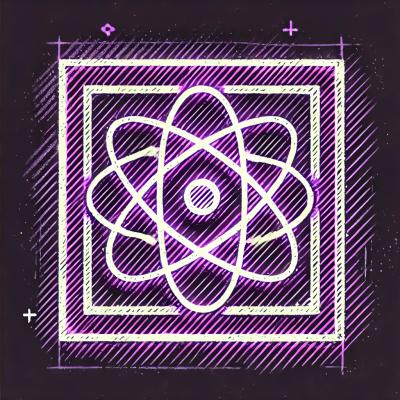
Security News
Create React App Officially Deprecated Amid React 19 Compatibility Issues
Create React App is officially deprecated due to React 19 issues and lack of maintenance—developers should switch to Vite or other modern alternatives.
Step-by-step tutorial of Conjugate Gradient SENSE (CG-SENSE) reconstruction by Hung P. Do.
This tutorial was written by Hung Do based on the RRSG_Challenge_01 github repository.
Original python code:
Hung Do’s modifications:
cgsense2023 PyPI Package re-written: Details can be seen in the P4_HPD_cgsense section.
Contributions to the below tasks are highly appreciated!
Document the standardization of the raw h5-file, otherwise
read_data()
has to be modified every time a deviation in h5-file is
encountered.
Refactor, simplify, modularize the gridding reconstructions. Currently, it is difficult to understand, especially for new learner.
Add dstore
flag in gradient descent or conjugate gradient
optimization functions to store intermediate reconstruction in each
iteration.
Add theoretical descriptions on MRI, MRI recons, and gradient Descent, and gonjugate gradient algorithms.
etc.
APA style:
Do, H. (2024). CG-SENSE Tutorial version 0.0.3 (0.0.3). Zenodo. https://doi.org/10.5281/zenodo.10547817
IEEE style:
H. Do, “CG-SENSE Tutorial version 0.0.3”. Zenodo, Jan. 21, 2024. doi: 10.5281/zenodo.10547817.
The cgsense2023 package was uploaded to PyPI and can be easily installed using the below command.
pip install cgsense2023
from cgsense2023.plot import *
import numpy as np
# Sequential - full - spoke radial trajectory
traj_full = gen_radial_traj(golden=False, full_spoke=True)
show_trajectory(traj_full, golden=True, figsize=(10,8))
# golden - full - spoke radial trajectory
traj_full_golden = gen_radial_traj(golden=True, full_spoke=True)
show_trajectory(traj_full_golden, golden=True, figsize=(10,8))
Nim, Nx, Ny = 12, 128, 256
x1 = np.random.randn(Nim, Nx, Ny)
x2 = np.random.randn(1, Nx, Ny)
show_image_grid(x1)
Warning: number of images (12) is larger than number of panels (2x5)!
show_image_grid(x2)
from cgsense2023.metrics import *
import numpy as np
# same dimensions
Nim, Nx, Ny = 11, 128, 256
x1 = np.random.randn(1, Nx, Ny)
x2 = np.random.randn(1, Nx, Ny)
print_metrics(x1, x2)
+---------+-----------+
| Metrics | Values |
+---------+-----------+
| MSE | 1.999e+00 |
| NMSE | 2.000e+00 |
| RMSE | 1.414e+00 |
| NRMSE | 1.414e+00 |
| PSNR | 14.981 |
| SSIM | 0.0092604 |
+---------+-----------+
from cgsense2023.math import *
from cgsense2023.io import *
import cgsense2023 as cgs2003
from cgsense2023.mri import *
from cgsense2023.optimizer import *
import h5py
import numpy as np
import matplotlib.pyplot as plt
# path to the data file
fpath = '../testdata/rawdata_brain.h5'
# path to the reference results
rpath = '../testdata/CG_reco_inscale_True_denscor_True_reduction_1.h5'
with h5py.File(rpath, 'r') as rf:
print(list(rf.keys()))
ref_cg = np.squeeze(rf['CG_reco'][()][-1])
ref_grid = np.squeeze(rf['Coil_images'][()])
['CG_reco', 'Coil_images']
ref_cg.shape, ref_grid.shape
((300, 300), (12, 300, 300))
# one stop shop for all Parameters and Data
params = setup_params(fpath, R=1)
['Coils', 'InScale', 'rawdata', 'trajectory']
# Set up MRI operator
mrimodel = MriImagingModel(params)
mriop = MriOperator(data_par=params["Data"],optimizer_par=params["Optimizer"])
mriop.set_operator(mrimodel)
# Single Coil images after FFT
my_grid = mriop.operator.NuFFT.adjoint(params['Data']['rawdata_density_cor'])
my_grid.shape, ref_grid.shape
((12, 300, 300), (12, 300, 300))
# test gridding recon results
np.allclose(ref_grid, my_grid)
True
# test gridding recon results
np.array_equal(ref_grid, my_grid)
False
print_metrics(np.abs(ref_grid[0]), np.abs(my_grid[0]))
+---------+-----------+
| Metrics | Values |
+---------+-----------+
| MSE | 1.472e-24 |
| NMSE | 5.905e-15 |
| RMSE | 1.213e-12 |
| NRMSE | 7.684e-08 |
| PSNR | 154.87 |
| SSIM | 1.0 |
+---------+-----------+
show_image_grid(my_grid, figsize=(10,10), rows=3, cols=4)
show_image_grid(rss_rec(my_grid), figsize=(10,10))
guess = np.zeros((params['Data']['image_dim'],params['Data']['image_dim']))
SD_result, SD_residuals, SD_ref_res = steepest_descent(mriop, guess,
params['Data']['rawdata_density_cor'],
iters=50,
ref=ref_cg)
Residuum at iter 50 : 6.553379e-06
show_compared_images(np.abs(ref_cg), np.abs(SD_result), diff_fac=10,
labels=['Reference', 'Steepest Descent', 'diff'])
np.allclose(ref_cg, SD_result)
False
print_metrics(np.abs(ref_cg), np.abs(SD_result))
+---------+-----------+
| Metrics | Values |
+---------+-----------+
| MSE | 5.633e-14 |
| NMSE | 7.888e-05 |
| RMSE | 2.373e-07 |
| NRMSE | 8.882e-03 |
| PSNR | 55.242 |
| SSIM | 0.9988 |
+---------+-----------+
CG_result, CG_residuals, CG_ref_res = conjugate_gradient(mriop, guess,
params['Data']['rawdata_density_cor'],
iters=50,
ref=ref_cg)
Residuum at iter 50 : 2.993229e-06
plt.plot(np.log10(SD_ref_res),'*--', label='SD reference_norms');
plt.plot(np.log10(SD_residuals),'*--', label='SD residual_norms');
plt.plot(np.log10(CG_ref_res),'*--', label='CG reference_norms');
plt.plot(np.log10(CG_residuals),'*--', label='CG residual_norms');
plt.grid();
plt.xlabel("# iteration")
plt.ylabel("residuals (log10)")
plt.legend();
Based on the “semi-convergence” plot above, the optimal number of iterations for CG and SD are around 10 and 28, respectively.
CG_result_vs_REF, _, _ = conjugate_gradient(mriop, guess,
params['Data']['rawdata_density_cor'],
iters=10,
ref=ref_cg)
Residuum at iter 10 : 1.826174e-05
show_compared_images(np.abs(ref_cg), np.abs(CG_result_vs_REF), diff_fac=200000,
labels=['Reference', 'Conjugate Gradient', 'diff'])
np.allclose(ref_cg, CG_result_vs_REF)
True
print_metrics(np.abs(ref_cg), np.abs(CG_result_vs_REF))
+---------+-----------+
| Metrics | Values |
+---------+-----------+
| MSE | 1.196e-22 |
| NMSE | 1.675e-13 |
| RMSE | 1.094e-11 |
| NRMSE | 4.093e-07 |
| PSNR | 141.97 |
| SSIM | 1.0 |
+---------+-----------+
If you want to develop cgsense2023
yourself, please use an editable
installation of cgsense2023
.
git clone https://github.com/hdocmsu/cgsense2023.git
pip install -e "cgsense2023[dev]"
You also need to use an editable installation of nbdev, fastcore, and execnb.
Happy Coding!!!
This template was created based on the below references. The list is not exhaustive so if you notice any missing references, please report an issue. I will promptly correct it.
RRSG_Challenge_01 github repository
fastMRI github repository
CG-SENSE revisited: Results from the first ISMRM reproducibility challenge
Prof. James V. Burke’s Lecture on “The Conjugate Gradient Algorithm”
Magnetic Resonance Image Reconstruction: Theory, Methods, and Applications, (2022)
If you would like to contribute to improve this repository please feel free to propose here.
MIT License as seen from the Original Repo’s License
MIT License
Copyright (c) 2020 ISMRM Reproducible Research Study Group
Permission is hereby granted, free of charge, to any person obtaining a copy of this software and associated documentation files (the “Software”), to deal in the Software without restriction, including without limitation the rights to use, copy, modify, merge, publish, distribute, sublicense, and/or sell copies of the Software, and to permit persons to whom the Software is furnished to do so, subject to the following conditions:
The above copyright notice and this permission notice shall be included in all copies or substantial portions of the Software.
THE SOFTWARE IS PROVIDED “AS IS”, WITHOUT WARRANTY OF ANY KIND, EXPRESS OR IMPLIED, INCLUDING BUT NOT LIMITED TO THE WARRANTIES OF MERCHANTABILITY, FITNESS FOR A PARTICULAR PURPOSE AND NONINFRINGEMENT. IN NO EVENT SHALL THE AUTHORS OR COPYRIGHT HOLDERS BE LIABLE FOR ANY CLAIM, DAMAGES OR OTHER LIABILITY, WHETHER IN AN ACTION OF CONTRACT, TORT OR OTHERWISE, ARISING FROM, OUT OF OR IN CONNECTION WITH THE SOFTWARE OR THE USE OR OTHER DEALINGS IN THE SOFTWARE.
FAQs
Conjugate Gradient SENSE (CG-SENSE) MRI Reconstruction
We found that cgsense2023 demonstrated a healthy version release cadence and project activity because the last version was released less than a year ago. It has 1 open source maintainer collaborating on the project.
Did you know?
Socket for GitHub automatically highlights issues in each pull request and monitors the health of all your open source dependencies. Discover the contents of your packages and block harmful activity before you install or update your dependencies.
Security News
Create React App is officially deprecated due to React 19 issues and lack of maintenance—developers should switch to Vite or other modern alternatives.
Security News
Oracle seeks to dismiss fraud claims in the JavaScript trademark dispute, delaying the case and avoiding questions about its right to the name.
Security News
The Linux Foundation is warning open source developers that compliance with global sanctions is mandatory, highlighting legal risks and restrictions on contributions.