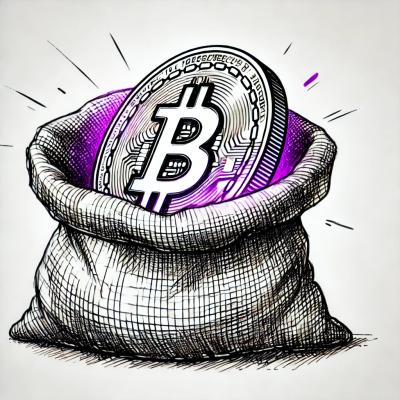
Research
Security News
Malicious npm Packages Use Telegram to Exfiltrate BullX Credentials
Socket uncovers an npm Trojan stealing crypto wallets and BullX credentials via obfuscated code and Telegram exfiltration.
Chatformers is a Python package that simplifies chatbot development by automatically managing chat history using local vector databases like Chroma DB.
⚡ Chatformers is a Python package designed to simplify the development of chatbot applications that use Large Language Models (LLMs). It offers automatic chat history management using a local vector database (ChromaDB, Qdrant or Pgvector), ensuring efficient context retrieval for ongoing conversations.
pip install chatformers
https://chatformers.mintlify.app/introduction
Read Documentation for advanced usage and understanding: https://chatformers.mintlify.app/development
from chatformers.chatbot import Chatbot
import os
from openai import OpenAI
system_prompt = None # use the default
metadata = None # use the default metadata
user_id = "Sam-Julia"
chat_model_name = "llama-3.1-70b-versatile"
memory_model_name = "llama-3.1-70b-versatile"
max_tokens = 150 # len of tokens to generate from LLM
limit = 4 # maximum number of memory to added during LLM chat
debug = True # enable to print debug messages
os.environ["GROQ_API_KEY"] = ""
llm_client = OpenAI(base_url="https://api.groq.com/openai/v1",
api_key="",
) # Any OpenAI Compatible LLM Client
config = {
"vector_store": {
"provider": "chroma",
"config": {
"collection_name": "test",
"path": "db",
}
},
"embedder": {
"provider": "ollama",
"config": {
"model": "nomic-embed-text:latest"
}
},
"llm": {
"provider": "groq",
"config": {
"model": memory_model_name,
"temperature": 0.1,
"max_tokens": 1000,
}
},
}
chatbot = Chatbot(config=config, llm_client=llm_client, metadata=None, system_prompt=system_prompt,
chat_model_name=chat_model_name, memory_model_name=memory_model_name,
max_tokens=max_tokens, limit=limit, debug=debug)
# Example to add buffer memory
memory_messages = [
{"role": "user", "content": "My name is Sam, what about you?"},
{"role": "assistant", "content": "Hello Sam! I'm Julia."},
{"role": "user", "content": "What do you like to eat?"},
{"role": "assistant", "content": "I like pizza"}
]
chatbot.add_memories(memory_messages, user_id=user_id)
# Buffer window memory, this will be acts as sliding window memory for LLM
message_history = [{"role": "user", "content": "where r u from?"},
{"role": "assistant", "content": "I am from CA, USA"},
{"role": "user", "content": "ok"},
{"role": "assistant", "content": "hmm"},
{"role": "user", "content": "What are u doing on next Sunday?"},
{"role": "assistant", "content": "I am all available"}
]
# Example to chat with the bot, send latest / current query here
query = "Could you remind me what do you like to eat?"
response = chatbot.chat(query=query, message_history=message_history, user_id=user_id, print_stream=True)
print("Assistant: ", response)
# # Example to check memories in bot based on user_id
# memories = chatbot.get_memories(user_id=user_id)
# for m in memories:
# print(m)
# print("================================================================")
# related_memories = chatbot.related_memory(user_id=user_id,
# query="yes i am sam? what us your name")
# print(related_memories)
Can I customize LLM endpoints / Groq or other models?
Can I use custom hosted chromadb, or any other vector db.
Need help or have suggestions?
FAQs
Chatformers is a Python package that simplifies chatbot development by automatically managing chat history using local vector databases like Chroma DB.
We found that chatformers demonstrated a healthy version release cadence and project activity because the last version was released less than a year ago. It has 1 open source maintainer collaborating on the project.
Did you know?
Socket for GitHub automatically highlights issues in each pull request and monitors the health of all your open source dependencies. Discover the contents of your packages and block harmful activity before you install or update your dependencies.
Research
Security News
Socket uncovers an npm Trojan stealing crypto wallets and BullX credentials via obfuscated code and Telegram exfiltration.
Research
Security News
Malicious npm packages posing as developer tools target macOS Cursor IDE users, stealing credentials and modifying files to gain persistent backdoor access.
Security News
AI-generated slop reports are making bug bounty triage harder, wasting maintainer time, and straining trust in vulnerability disclosure programs.