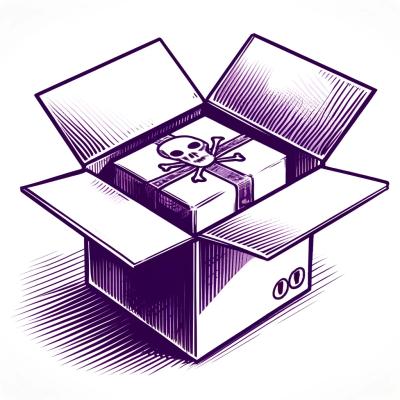
Security News
Research
Data Theft Repackaged: A Case Study in Malicious Wrapper Packages on npm
The Socket Research Team breaks down a malicious wrapper package that uses obfuscation to harvest credentials and exfiltrate sensitive data.
.. figure:: https://img.shields.io/pypi/dm/cynet.svg :alt: cynet PyPI Downloads .. figure:: https://img.shields.io/pypi/v/cynet.svg :alt: cynet version
.. image:: http://zed.uchicago.edu/logo/logozed1.png :height: 400px :scale: 50 % :alt: alternate text :align: center
.. class:: no-web no-pdf
:Info: See https://arxiv.org/abs/1406.6651 for theoretical background :Author: ZeD@UChicago <zed.uchicago.edu> :Description: Implementation of the Deep Granger net inference algorithm, described in https://arxiv.org/abs/1406.6651, for learning spatio-temporal stochastic processes (point processes). cynet learns a network of generative local models, without assuming any specific model structure.
.. NOTE:: If issues arise with dependencies in python3, be sure that tkinter is installed
.. code-block::
sudo apt-get install python3-tk
Usage:
.. code-block::
from cynet import cynet
from cynet.cynet import uNetworkModels as models
from viscynet import viscynet as vcn
cynet module includes:
cynet library classes:
* spatioTemporal
* uNetworkModels
* simulateModels
* xgModels
Examples of Pipeline:
You may find two examples of this pipeline in your enviroment's bin folder
after installing the cynet package. There will also be a pdf walking through
another extremely detailed example.
Produces detailed timeseries predictions using Deep Granger Nets.
.. image:: https://zed.uchicago.edu/img/cynetpred.png
:align: center
:scale: 50 %
Description of Pipeline:
You may find two examples of this pipeline in your enviroment's bin folder
after installing the cynet package.
Step 1:
Use the spatioTemporal class and its utility functions to fit and
manipulate your data into a timeseries grid. The end outputs will be triplets:
files that contain the rows (coordinates), the columns (dates), and the timeseries.
The splitTS function will help generate rows of the timeseries. Generally, we
use this to create timeseries beyond the length of the data in the triplets.
We use the triplets to generate predictive models and then split, which have
the longer timeseries to evaluate those models.
Step 2:
Run xGenESeSS on the triplets to generate predictive models. The
xgModels class can be used to assist in this step. If running on a cluster,
set run local to false and calling xgModels.run() will generate the shell
commands to run xGenESeSS in a text file. Otherwise, xgModels will run
locally using the binary installed with the package. The end result are predictive
models. Note that example 1 starts at this point. Thus there are sample models
provided.
Step 3:
To evaluate the models afterwards, use the run_pipeline utility function.
This calls uNetworkModels and simulateModels in parallel to evaluate each model.
simulateModels calls the cynet and flexroc binaries. Outputs will be auc, tpr,
and fpr statistics.
See example 2 for an example of the entire pipeline.
FAQs
Learning Point Processes Using Deep Granger Nets
We found that cynet demonstrated a healthy version release cadence and project activity because the last version was released less than a year ago. It has 4 open source maintainers collaborating on the project.
Did you know?
Socket for GitHub automatically highlights issues in each pull request and monitors the health of all your open source dependencies. Discover the contents of your packages and block harmful activity before you install or update your dependencies.
Security News
Research
The Socket Research Team breaks down a malicious wrapper package that uses obfuscation to harvest credentials and exfiltrate sensitive data.
Research
Security News
Attackers used a malicious npm package typosquatting a popular ESLint plugin to steal sensitive data, execute commands, and exploit developer systems.
Security News
The Ultralytics' PyPI Package was compromised four times in one weekend through GitHub Actions cache poisoning and failure to rotate previously compromised API tokens.