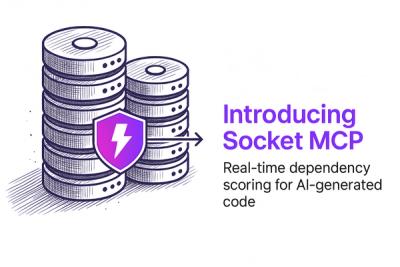
Product
Secure Your AI-Generated Code with Socket MCP
Socket MCP brings real-time security checks to AI-generated code, helping developers catch risky dependencies before they enter the codebase.
RPyC implementation for DCC software integration with Model Context Protocol (MCP). This package provides a framework for exposing DCC functionality via RPYC, allowing for remote control of DCC applications.
RPyC (Remote Python Call) offers significant advantages for DCC software integration:
cmds
/pymel
, Houdini's hou
, Blender's bpy
, and Nuke's Python API without translation layers.By leveraging RPyC, DCC-MCP-RPYC provides a unified framework that preserves the native feel of each DCC's API while enabling remote control capabilities.
The architecture of DCC-MCP-RPYC is designed to provide a unified interface for controlling various DCC applications:
graph TD
A[Client App<br>AI Assistant] --> B[MCP Server<br>Coordinator]
B --> C[DCC Software<br>Maya/Houdini]
A --> D[DCC-MCP<br>Core API]
D --> E[DCC-MCP-RPYC<br>Transport]
E --> C
F[Action System] --> E
G[Mock DCC Services] -.-> E
Key components:
pip install dcc-mcp-rpyc
Or with Poetry:
poetry add dcc-mcp-rpyc
# Create and start a DCC server in Maya
from dcc_mcp_rpyc.server import create_dcc_server, DCCRPyCService
# Create a custom service class
class MayaService(DCCRPyCService):
def get_scene_info(self):
# Implement Maya-specific scene info retrieval
return {"scene": "Maya scene info"}
def exposed_execute_cmd(self, cmd_name, *args, **kwargs):
# Implement Maya command execution
pass
# Create and start the server
server = create_dcc_server(
dcc_name="maya",
service_class=MayaService,
port=18812 # Optional, will use random port if not specified
)
# Start the server (threaded=True to avoid blocking Maya's main thread)
server.start(threaded=True)
from dcc_mcp_rpyc.server import create_service_factory, create_shared_service_instance, create_raw_threaded_server
# Create a shared state manager
class SceneManager:
def __init__(self):
self.scenes = {}
def add_scene(self, name, data):
self.scenes[name] = data
# Method 1: Create a service factory (new instance per connection)
scene_manager = SceneManager()
service_factory = create_service_factory(MayaService, scene_manager)
# Method 2: Create a shared service instance (single instance for all connections)
shared_service = create_shared_service_instance(MayaService, scene_manager)
# Create a server with the service factory
server = create_raw_threaded_server(service_factory, port=18812)
server.start()
from dcc_mcp_rpyc.parameters import process_rpyc_parameters, execute_remote_command
# Process parameters for RPyC calls
params = {"radius": 5.0, "create": True, "name": "mySphere"}
processed = process_rpyc_parameters(params)
# Execute a command on a remote connection with proper parameter handling
result = execute_remote_command(connection, "create_sphere", radius=5.0, create=True)
from dcc_mcp_rpyc.client import BaseDCCClient
# Connect to a DCC server
client = BaseDCCClient(
dcc_name="maya",
host="localhost",
port=18812 # Optional, will discover automatically if not specified
)
# Connect to the server
client.connect()
# Execute Python code in the DCC
result = client.execute_python("import maya.cmds as cmds; _result = cmds.ls()")
print(result)
# Execute DCC-specific command
result = client.execute_dcc_command("sphere -name test_sphere;")
print(result)
# Get scene information
scene_info = client.get_scene_info()
print(scene_info)
# Get DCC application information
dcc_info = client.get_dcc_info()
print(dcc_info)
# Disconnect when done
client.disconnect()
from dcc_mcp_rpyc.client import ConnectionPool
# Create a connection pool
pool = ConnectionPool()
# Get a client from the pool (creates a new connection if needed)
with pool.get_client("maya", host="localhost") as client:
# Call methods on the client
result = client.execute_python("import maya.cmds as cmds; _result = cmds.sphere()")
print(result)
# Connection is automatically returned to the pool
from dcc_mcp_rpyc.action_adapter import ActionAdapter, get_action_adapter
from dcc_mcp_core.actions.base import Action
from dcc_mcp_core.models import ActionResultModel
from pydantic import BaseModel, Field
# Define an Action input model
class CreateSphereInput(BaseModel):
radius: float = Field(default=1.0, description="Sphere radius")
name: str = Field(default="sphere1", description="Sphere name")
# Define an Action
class CreateSphereAction(Action):
name = "create_sphere"
input_model = CreateSphereInput
def execute(self, input_data: CreateSphereInput) -> ActionResultModel:
# Implementation would use DCC-specific API
return ActionResultModel(
success=True,
message=f"Created sphere {input_data.name} with radius {input_data.radius}",
context={"name": input_data.name, "radius": input_data.radius}
)
# Get or create an action adapter
adapter = get_action_adapter("maya")
# Register the action
adapter.register_action(CreateSphereAction)
# Call the action
result = adapter.call_action("create_sphere", radius=2.0, name="mySphere")
print(result.message) # "Created sphere mySphere with radius 2.0"
import threading
import rpyc
from rpyc.utils.server import ThreadedServer
from dcc_mcp_rpyc.client import BaseDCCClient
from dcc_mcp_rpyc.utils.discovery import register_service
# Create a mock DCC service
class MockDCCService(rpyc.Service):
def exposed_get_dcc_info(self, conn=None):
return {
"name": "mock_dcc",
"version": "1.0.0",
"platform": "windows",
}
def exposed_execute_python(self, code, conn=None):
# Safe execution of Python code in a controlled environment
local_vars = {}
exec(code, {}, local_vars)
if "_result" in local_vars:
return local_vars["_result"]
return None
# Start the mock service
server = ThreadedServer(
MockDCCService,
port=18812,
protocol_config={"allow_public_attrs": True}
)
# Register the service for discovery
register_service("mock_dcc", "localhost", 18812)
# Start in a separate thread
thread = threading.Thread(target=server.start, daemon=True)
thread.start()
# Connect a client to the mock service
client = BaseDCCClient("mock_dcc", host="localhost", port=18812)
client.connect()
# Use the client as if it were connected to a real DCC
dcc_info = client.get_dcc_info()
print(dcc_info) # {"name": "mock_dcc", "version": "1.0.0", "platform": "windows"}
from dcc_mcp_rpyc.dcc_adapter import DCCAdapter
from dcc_mcp_rpyc.client import BaseDCCClient
class MayaAdapter(DCCAdapter):
def _create_client(self) -> BaseDCCClient:
return BaseDCCClient(
dcc_name="maya",
host=self.host,
port=self.port,
timeout=self.timeout
)
def create_sphere(self, radius=1.0):
self.ensure_connected()
return self.dcc_client.execute_dcc_command(f"sphere -r {radius};")
# Clone the repository
git clone https://github.com/loonghao/dcc-mcp-rpyc.git
cd dcc-mcp-rpyc
# Install dependencies with Poetry
poetry install
# Run tests with nox
nox -s pytest
# Run linting
nox -s lint
# Fix linting issues
nox -s lint-fix
MIT
FAQs
RPYC implementation for DCC software integration with Model Context Protocol
We found that dcc-mcp-rpyc demonstrated a healthy version release cadence and project activity because the last version was released less than a year ago. It has 1 open source maintainer collaborating on the project.
Did you know?
Socket for GitHub automatically highlights issues in each pull request and monitors the health of all your open source dependencies. Discover the contents of your packages and block harmful activity before you install or update your dependencies.
Product
Socket MCP brings real-time security checks to AI-generated code, helping developers catch risky dependencies before they enter the codebase.
Security News
As vulnerability data bottlenecks grow, the federal government is formally investigating NIST’s handling of the National Vulnerability Database.
Research
Security News
Socket’s Threat Research Team has uncovered 60 npm packages using post-install scripts to silently exfiltrate hostnames, IP addresses, DNS servers, and user directories to a Discord-controlled endpoint.