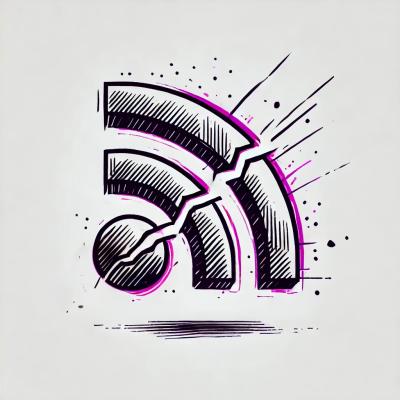
Security News
CISA Kills Off RSS Feeds for KEVs and Cyber Alerts
CISA is discontinuing official RSS support for KEV and cybersecurity alerts, shifting updates to email and social media, disrupting automation workflows.
This package contains the AI models used by the Docling PDF conversion package
AI modules to support the Docling PDF document conversion project.
To install poetry
locally, use either pip
or homebrew
.
To install poetry
on a docker container, do the following:
ENV POETRY_NO_INTERACTION=1 \
POETRY_VIRTUALENVS_CREATE=false
# Install poetry
RUN curl -sSL 'https://install.python-poetry.org' > install-poetry.py \
&& python install-poetry.py \
&& poetry --version \
&& rm install-poetry.py
To install and run the package, simply set up a poetry environment
poetry env use $(which python3.10)
poetry shell
and install all the dependencies,
poetry install # this will only install the deps from the poetry.lock
poetry install --no-dev # this will skip installing dev dependencies
To update or add new dependencies from pyproject.toml
, rebuild poetry.lock
poetry update
When in development mode on MacOS with Intel chips, one can use compatible dependencies with
poetry update --with mac_intel
Below we list datasets used with their description, source, and "TableFormer Format". The TableFormer Format is our processed version of the version of the original format to work with the dataloader out of the box, and to augment the dataset when necassary to add missing groundtruth (bounding boxes for empty cells).
Name | Description | URL |
---|---|---|
PubTabNet | PubTabNet contains heterogeneous tables in both image and HTML format, 516k+ tables in the PubMed Central Open Access Subset | PubTabNet |
FinTabNet | A dataset for Financial Report Tables with corresponding ground truth location and structure. 112k+ tables included. | FinTabNet |
TableBank | TableBank is a new image-based table detection and recognition dataset built with novel weak supervision from Word and Latex documents on the internet, contains 417K high-quality labeled tables. | TableBank |
TableModel04rs (OTSL) is our SOTA method that using transformers in order to predict table structure and bounding box.
Example configuration can be found inside test tests/test_tf_predictor.py
These are the main sections of the configuration file:
dataset
: The directory for prepared data and the parameters used during the data loading.model
: The type, name and hyperparameters of the model. Also the directory to save/load the
trained checkpoint files.train
: Parameters for the training of the model.predict
: Parameters for the evaluation of the model.dataset_wordmap
: Very important part that contains token maps.You can download the model weights and config files from the links:
You can run the inference tests for the models with:
python -m pytest tests/
This will also generate prediction and matching visualizations that can be found here:
tests\test_data\viz\
Visualization outlines:
Light Pink
: border of recognized tableGrey
: OCR cellsGreen
: prediction bboxesRed
: OCR cells matched with predictionBlue
: Post processed, matchBold Blue
: column headerBold Magenta
: row headerBold Brown
: section row (if table have one)A demo application allows to apply the LayoutPredictor
on a directory <input_dir>
that contains
png
images and visualize the predictions inside another directory <viz_dir>
.
First download the model weights (see above), then run:
python -m demo.demo_layout_predictor -i <input_dir> -v <viz_dir>
e.g.
python -m demo.demo_layout_predictor -i tests/test_data/samples -v viz/
FAQs
This package contains the AI models used by the Docling PDF conversion package
We found that docling-ibm-models demonstrated a healthy version release cadence and project activity because the last version was released less than a year ago. It has 1 open source maintainer collaborating on the project.
Did you know?
Socket for GitHub automatically highlights issues in each pull request and monitors the health of all your open source dependencies. Discover the contents of your packages and block harmful activity before you install or update your dependencies.
Security News
CISA is discontinuing official RSS support for KEV and cybersecurity alerts, shifting updates to email and social media, disrupting automation workflows.
Security News
The MCP community is launching an official registry to standardize AI tool discovery and let agents dynamically find and install MCP servers.
Research
Security News
Socket uncovers an npm Trojan stealing crypto wallets and BullX credentials via obfuscated code and Telegram exfiltration.