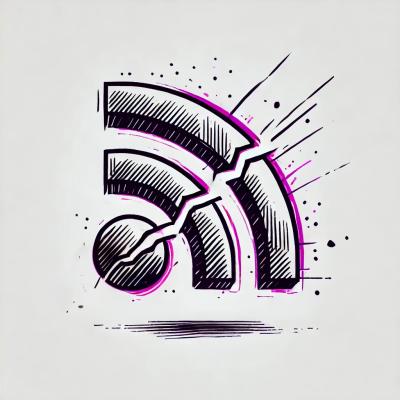
Security News
CISA Kills Off RSS Feeds for KEVs and Cyber Alerts
CISA is discontinuing official RSS support for KEV and cybersecurity alerts, shifting updates to email and social media, disrupting automation workflows.
Ancestral sequence reconstruction using a tree structured Ornstein Uhlenbeck variational autoencoder
DRAUPNIR: "Beta library version for performing ASR using a tree-structured Variational Autoencoder"
Extra requirements for tree inference:
#These are NOT necessary if you have your own tree file or for using the default datasets
IQ-Tree: http://www.iqtree.org/doc/Quickstart
conda install -c bioconda iqtree
RapidNJ: https://birc.au.dk/software/rapidnj
conda config --add channels bioconda
conda install rapidnj
Extra requirements for fast patristic matrix construction
#Recommended if you have more than 200 sequences. The patristic matrix is constructed only once
Install R (R version 4.1.2 (2021-11-01) -- "Bird Hippie" )
sudo apt update & sudo apt upgrade
sudo apt -y install r-base
together with ape 5.5 and TreeDist 2.3 libraries
install.packages(c("ape","TreeDist"))
Draupnir python environment .yaml
https://github.com/LysSanzMoreta/DRAUPNIR_ASR/tree/main/draupnir/env/Python3_9
Draupnir pip install
pip install draupnir
Example
See Draupnir_example.py
Which guide to use?
By experience, use delta_map, since the marginal results (Test folder) are the most stable. It is recommended to run the model both with the variational and the delta_map guides and compare outputs using the mutual information. If necessary, run the variational guide longer than the delta_map, since it has more parameters to optimize and takes longer.
How long should I run my model?
Observe the latent space:
Observe the sampled training (leaves) sequences and test (internal) sequences: Navigate to the Train_argmax and Test_argmax folders and look for the .fasta files
Calculate mutual information:
Datasets #They are recommended to use with the pipeline, look into datasets.py for more details
dict_urls = {
"aminopeptidase":"https://drive.google.com/drive/folders/1fLsOJbD1hczX15NW0clCgL6Yf4mnx_yl?usp=sharing",
"benchmark_randall_original_naming":"https://drive.google.com/drive/folders/1oE5-22lqcobZMIguatOU_Ki3N2Fl9b4e?usp=sharing",
"Coral_all":"https://drive.google.com/drive/folders/1IbfiM2ww5PDcDSpTjrWklRnugP8RdUTu?usp=sharing",
"Coral_Faviina":"https://drive.google.com/drive/folders/1Ehn5xNNYHRu1iaf7vS66sbAESB-dPJRx?usp=sharing",
"PDB_files_Draupnir_PF00018_116":"https://drive.google.com/drive/folders/1YJDS_oHHq-5qh2qszwk-CucaYWa9YDOD?usp=sharing",
"PDB_files_Draupnir_PF00400_185": "https://drive.google.com/drive/folders/1LTOt-dhksW1ZsBjb2uzi2NB_333hLeu2?usp=sharing",
"PF00096":"https://drive.google.com/drive/folders/103itCfxiH8jIjKYY9Cvy7pRGyDl9cnej?usp=sharing",
"PF00400":"https://drive.google.com/drive/folders/1Ql10yTItcdX93Xpz3Oh-sl9Md6pyJSZ3?usp=sharing",
"SH3_pf00018_larger_than_30aa":"https://drive.google.com/drive/folders/1Mww3uvF_WonpMXhESBl9Jjes6vAKPj5f?usp=sharing",
"simulations_blactamase_1":"https://drive.google.com/drive/folders/1ecHyqnimdnsbeoIh54g2Wi6NdGE8tjP4?usp=sharing",
"simulations_calcitonin_1":"https://drive.google.com/drive/folders/1jJ5RCfLnJyAq0ApGIPrXROErcJK3COvK?usp=sharing",
"simulations_insulin_2":"https://drive.google.com/drive/folders/1xB03AF_DYv0EBTwzUD3pj03zBcQDDC67?usp=sharing",
"simulations_PIGBOS_1":"https://drive.google.com/drive/folders/1KTzfINBVo0MqztlHaiJFoNDt5gGsc0dK?usp=sharing",
"simulations_sirtuins_1":"https://drive.google.com/drive/folders/1llT_HvcuJQps0e0RhlfsI1OLq251_s5S?usp=sharing",
"simulations_src_sh3_1":"https://drive.google.com/drive/folders/1tZOn7PrCjprPYmyjqREbW9PFTsPb29YZ?usp=sharing",
"simulations_src_sh3_2":"https://drive.google.com/drive/folders/1ji4wyUU4aZQTaha-Uha1GBaYruVJWgdh?usp=sharing",
"simulations_src_sh3_3":"https://drive.google.com/drive/folders/13xLOqW2ldRNm8OeU-bnp9DPEqU1d31Wy?usp=sharing"
}
Dataset | Number of leaves | Alignment lenght | Name |
---|---|---|---|
Randall's Coral fluorescent proteins (CFP) | 19 | 225 | benchmark_randall_original_naming |
Coral fluorescent proteins (CFP) Faviina subclade | 35 | 361 | Coral_Faviina |
Coral fluorescent proteins (CFP) subclade | 71 | 272 | Coral_all |
Simulation $\beta$-Lactamase | 32 | 314 | simulations_blactamase_1 |
Simulation Calcitonin | 50 | 71 | simulations_calcitonin_1 |
Simulation SRC-Kinase SH3 domain | 100 | 63 | simulations_src_sh3_1 |
Simulation Sirtuin | 150 | 477 | simulations_sirtuins_1 |
Simulation SRC-kinase SH3 domain | 200 | 128 | simulations_src_sh3_3 |
Simulation PIGBOS | 300 | 77 | simulations_PIGBOS_1 |
Simulation Insulin | 400 | 558 | simulations_insulin_2 |
Simulation SRC-kinase SH3 domain | 800 | 99 | simulations_src_sh3_2 |
What do the results folders mean?
Where are my ancestral sequences?
In each of the folders there should be a fasta file _sampled_nodes_seq.fasta
Each of the sequences in the file should be identified as //_sample_
-Node-name-input-tree: Original name of the node in the given input tree
-Tree-level-order: Position of the node in tree-level order in the tree
Node_A1//1.0_sample_0
If this library is useful for your research please cite:
@inproceedings{moreta2021ancestral,
title={Ancestral protein sequence reconstruction using a tree-structured Ornstein-Uhlenbeck variational autoencoder},
author={Moreta, Lys Sanz and R{\o}nning, Ola and Al-Sibahi, Ahmad Salim and Hein, Jotun and Theobald, Douglas and Hamelryck, Thomas},
booktitle={International Conference on Learning Representations},
year={2021}
}
Do not hesitate to give input on how to improve the documentation of this library
**Leave like and subscribe ... wait that was somewhere else ... well, a star will do it ;) **
FAQs
Ancestral sequence reconstruction using a tree structured Ornstein Uhlenbeck variational autoencoder
We found that draupnir demonstrated a healthy version release cadence and project activity because the last version was released less than a year ago. It has 1 open source maintainer collaborating on the project.
Did you know?
Socket for GitHub automatically highlights issues in each pull request and monitors the health of all your open source dependencies. Discover the contents of your packages and block harmful activity before you install or update your dependencies.
Security News
CISA is discontinuing official RSS support for KEV and cybersecurity alerts, shifting updates to email and social media, disrupting automation workflows.
Security News
The MCP community is launching an official registry to standardize AI tool discovery and let agents dynamically find and install MCP servers.
Research
Security News
Socket uncovers an npm Trojan stealing crypto wallets and BullX credentials via obfuscated code and Telegram exfiltration.