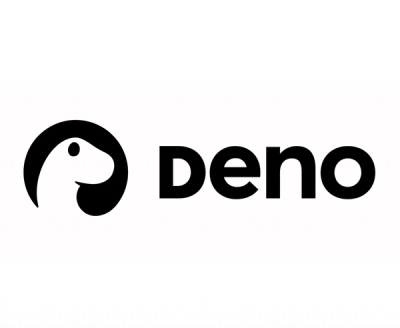
Security News
Deno 2.4 Brings Back deno bundle, Improves Dependency Management and Observability
Deno 2.4 brings back bundling, improves dependency updates and telemetry, and makes the runtime more practical for real-world JavaScript projects.
duplicate-recognition
Advanced tools
This Project uses the calculation of similarities scores of a set of entities in an edge list. To allow for versatile usage, it uses dependency injection to implement it into any application.
This Project uses the calculation of similarities scores of a set of entities in an edge list. To allow for versatile usage, it uses dependency injection to implement it into any application.
You need to implement all the read/write methods, to keep the project versatile.
For an example on how to use it, see the example, or the following code block:
"""
This is an example implementation of the DuplicateRecognition class.
It won't work. It's just to show, how it could be used.
"""
import logging
import os
from collections import defaultdict
from typing import Dict, Set
from typing import Generator, Tuple, Any
from itertools import chain, islice
from mysql.connector import connect
from duplicate_recognition import DuplicateRecognition, Algorithm, Comparison
logging.basicConfig(level=logging.DEBUG)
def chunks(iterable, size=1000):
# https://stackoverflow.com/a/24527424
iterator = iter(iterable)
for first in iterator:
yield chain([first], islice(iterator, size - 1))
class Entity(DuplicateRecognition):
ID_COLUMN: str = "id"
F_SCORES: Dict[str, float] = defaultdict(lambda: 0, {
"id": DuplicateRecognition.F_SCORE_FOR_EXACT_MATCH,
"company": 1,
"postal_code": 1,
"country": 0.5,
})
MATCHING_ALGORITHM: Dict[str, Algorithm] = defaultdict(lambda: Algorithm.EQUALITY, {
"id": Algorithm.EQUALITY,
"company": Algorithm.PHONETIC_DISTANCE,
"postal_code": Algorithm.EQUALITY,
"country": Algorithm.COUNTRY,
})
THRESHOLDS: Dict[str, float] = defaultdict(lambda: 0, {
"country": 1,
})
NEGATIVE_FIELDS: Set[str] = {"country"}
def __init__(self):
self.connection = connect(
host=os.getenv("MYSQL_HOST"),
port=os.getenv("MYSQL_PORT"),
user=os.getenv("MYSQL_USER"),
password=os.getenv("MYSQL_PASSWORD"),
database="foo",
)
super().__init__()
def get_relevant_entities(self) -> Generator[Dict[str, Any], None, None]:
cursor = self.connection.cursor(dictionary=True)
cursor.execute("""
SELECT DISTINCT * FROM entity
ORDER BY entity.id ASC
""")
return cursor
def get_refresh_pairs(self) -> Generator[Tuple[int, int], None, None]:
cursor = self.connection.cursor(buffered=True)
cursor.execute("""
SELECT entity_edge_list.a, entity_edge_list.b
FROM entity_edge_list
INNER JOIN entity
ON entity.id = entity_edge_list.a OR entity.id = entity_edge_list.b
WHERE entity.change_date > entity_edge_list.change_date
ORDER BY entity_edge_list.a, entity_edge_list.b ASC
""")
return cursor
def get_compared(self) -> Generator[int, None, None]:
cursor = self.connection.cursor(buffered=True)
cursor.execute("SELECT DISTINCT a FROM entity_edge_list")
for row in cursor:
yield row[0]
def get_uncompared(self) -> Generator[int, None, None]:
cursor = self.connection.cursor(buffered=True)
cursor.execute("""
SELECT DISTINCT entity.id
FROM entity
LEFT JOIN entity_edge_list
ON entity.id = entity_edge_list.a
WHERE entity_edge_list.a IS NULL
ORDER BY entity.id ASC
""")
for row in cursor:
yield row[0]
def write_comparisons(self, comparisons: Generator[Comparison, None, None]):
cursor = self.connection.cursor()
query = f"""
INSERT INTO entity_edge_list (a, b, score, count, f_score_sum, change_date) VALUE (%s, %s, %s, %s, %s, NOW())
ON DUPLICATE KEY UPDATE score=VALUES(score), count=VALUES(count), f_score_sum=VALUES(f_score_sum), change_date=NOW();
"""
# execute in batches of 1000
for chunk in chunks(comparisons, size=1000):
cursor.executemany(query, [
(c.entity[self.ID_COLUMN], c.other_entity[self.ID_COLUMN], c.score, c.count, c.f_score_sum)
for c in chunk
])
self.connection.commit()
if __name__ == "__main__":
Entity().execute(limit=None)
FAQs
This Project uses the calculation of similarities scores of a set of entities in an edge list. To allow for versatile usage, it uses dependency injection to implement it into any application.
We found that duplicate-recognition demonstrated a healthy version release cadence and project activity because the last version was released less than a year ago. It has 1 open source maintainer collaborating on the project.
Did you know?
Socket for GitHub automatically highlights issues in each pull request and monitors the health of all your open source dependencies. Discover the contents of your packages and block harmful activity before you install or update your dependencies.
Security News
Deno 2.4 brings back bundling, improves dependency updates and telemetry, and makes the runtime more practical for real-world JavaScript projects.
Security News
CVEForecast.org uses machine learning to project a record-breaking surge in vulnerability disclosures in 2025.
Security News
Browserslist-rs now uses static data to reduce binary size by over 1MB, improving memory use and performance for Rust-based frontend tools.