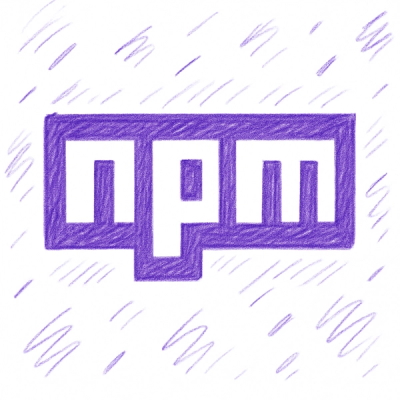
Security News
npm Adopts OIDC for Trusted Publishing in CI/CD Workflows
npm now supports Trusted Publishing with OIDC, enabling secure package publishing directly from CI/CD workflows without relying on long-lived tokens.
EchoSwift is a powerful and flexible tool designed for benchmarking Large Language Model (LLM) inference. It allows users to measure and analyze the performance of LLM endpoints across various metrics, including token latency, throughput, and time to first token (TTFT).
The performance metrics captured for varying input and output tokens and parallel users while running the benchmark includes
You can install EchoSwift using pip:
pip install echoswift
Alternatively, you can install from source:
git clone https://github.com/Infobellit-Solutions-Pvt-Ltd/EchoSwift.git
cd EchoSwift
pip install -e .
EchoSwift provides a simple CLI interface for running LLM Inference benchmarks.
Below are the steps to run a sample test, assuming the generation endpoint is active.
config.json
Before running a benchmark, you need to download and filter the dataset:
echoswift dataprep
This command will download the filtered ShareGPT dataset from Huggingface and creates a sample config.json
Modify the config.json
file in the project root directory. Here's an example configuration:
{
"_comment": "EchoSwift Configuration",
"out_dir": "test_results",
"base_url": "http://10.216.178.15:8000/v1/completions",
"provider": "vLLM",
"model": "meta-llama/Meta-Llama-3-8B",
"max_requests": 5,
"user_counts": [3],
"input_tokens": [32],
"output_tokens": [256]
}
Adjust these parameters according to your LLM endpoint you're benchmarking.
To start the benchmark using the configuration from config.json
:
echoswift start --config path/to/your/config.json
echoswift plot --results-dir path/to/your/results_dir
EchoSwift will create a results
directory (or the directory specified in out_dir
) containing:
After the benchmark completes, you can find CSV files in the output directory. These files contain information about latency, throughput, and TTFT for each test configuration.
If you find our resource useful, please cite our paper:
@inproceedings{Krishna2024,
series = {ICPE '24},
title = {EchoSwift: An Inference Benchmarking and Configuration Discovery Tool for Large Language Models (LLMs)},
url = {https://dl.acm.org/doi/10.1145/3629527.3652273},
DOI = {10.1145/3629527.3652273},
booktitle = {Companion of the 15th ACM/SPEC International Conference on Performance Engineering},
publisher = {ACM},
author = {Krishna, Karthik and Bandili, Ramana},
year = {2024},
month = May,
collection = {ICPE '24}
}
FAQs
LLM Inference Benchmarking Tool
We found that echoswift demonstrated a healthy version release cadence and project activity because the last version was released less than a year ago. It has 1 open source maintainer collaborating on the project.
Did you know?
Socket for GitHub automatically highlights issues in each pull request and monitors the health of all your open source dependencies. Discover the contents of your packages and block harmful activity before you install or update your dependencies.
Security News
npm now supports Trusted Publishing with OIDC, enabling secure package publishing directly from CI/CD workflows without relying on long-lived tokens.
Research
/Security News
A RubyGems malware campaign used 60 malicious packages posing as automation tools to steal credentials from social media and marketing tool users.
Security News
The CNA Scorecard ranks CVE issuers by data completeness, revealing major gaps in patch info and software identifiers across thousands of vulnerabilities.