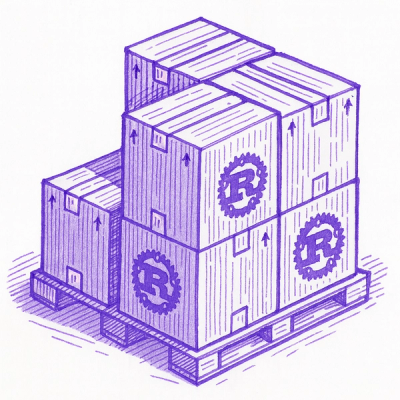
Security News
Crates.io Implements Trusted Publishing Support
Crates.io adds Trusted Publishing support, enabling secure GitHub Actions-based crate releases without long-lived API tokens.
Remap, mask, renumber, unique, and in-place transposition of 3D labeled images. Point cloud too.
Renumber and relabel Numpy arrays at C++ speed and physically convert rectangular Numpy arrays between C and Fortran order using an in-place transposition.
import fastremap
uniq, cts = fastremap.unique(labels, return_counts=True) # may be much faster than np.unique
idxs = fastremap.indices(labels, 1231) # important for huge arrays
labels, remapping = fastremap.renumber(labels, in_place=True) # relabel values from 1 and refit data type
ptc = fastremap.point_cloud(labels) # dict of coordinates by label
labels = fastremap.refit(labels) # resize the data type of the array to fit extrema
labels = fastremap.refit(labels, value=-35) # resize the data type to fit the value provided
wider_dtype = fastremap.widen_dtype(np.uint32) # np.uint64
narrower_dtype = fastremap.narrow_dtype(np.uint32) # np.uint16
# remap all occurances of 1 -> 2
labels = fastremap.remap(labels, { 1: 2 }, preserve_missing_labels=True, in_place=True)
labels = fastremap.mask(labels, [1,5,13]) # set all occurances of 1,5,13 to 0
labels = fastremap.mask_except(labels, [1,5,13]) # set all labels except 1,5,13 to 0
mapping = fastremap.component_map([ 1, 2, 3, 4 ], [ 5, 5, 6, 7 ]) # { 1: 5, 2: 5, 3: 6, 4: 7 }
mapping = fastremap.inverse_component_map([ 1, 2, 1, 3 ], [ 4, 4, 5, 6 ]) # { 1: [ 4, 5 ], 2: [ 4 ], 3: [ 6 ] }
fastremap.transpose(labels) # physically transpose labels in-place
fastremap.ascontiguousarray(labels) # try to perform a physical in-place transposition to C order
fastremap.asfortranarray(labels) # try to perform a physical in-place transposition to F order
minval, maxval = fastremap.minmax(labels) # faster version of (np.min(labels), np.max(labels))
# computes number of matching adjacent pixel pairs in an image
num_pairs = fastremap.pixel_pairs(labels)
n_foreground = fastremap.foreground(labels) # number of nonzero voxels
# computes the cutout.tobytes(order) of each chunk and returns
# the binaries indexed by fortran order in the order specified (C or F)
# If the input image is F contiguous and F is requested, or C and C order,
# and the image is larger than a single chunk, this will be significantly
# faster than iterating and using tobytes.
binaries = fastremap.tobytes(labels, (64,64,64), order="F")
np.unique
.np.transpose
function otherwise.np.asfortranarray
function otherwise.np.ascontiguousarray
function otherwise.pip
Installationpip install fastremap
If not, a C++ compiler is required.
pip install numpy
pip install fastremap --no-binary :all:
A C++ compiler is required.
sudo apt-get install g++ python3-dev
mkvirtualenv -p python3 fastremap
pip install numpy
# Choose one:
python setup.py develop
python setup.py install
Python loops are slow, so Numpy is often used to perform remapping on large arrays (hundreds of megabytes or gigabytes). In order to efficiently remap an array in Numpy you need a key-value array where the index is the key and the value is the contents of that index.
import numpy as np
original = np.array([ 1, 3, 5, 5, 10 ])
remap = np.array([ 0, -5, 0, 6, 0, 0, 2, 0, 0, 0, -100 ])
# Keys: 0 1 2 3 4 5 6 7 8 9 10
remapped = remap[ original ]
>>> [ -5, 6, 2, 2, -100 ]
If there are 32 or 64 bit labels in the array, this becomes impractical as the size of the array can grow larger than RAM. Therefore, it would be helpful to be able to perform this mapping using a C speed loop. Numba can be used for this in some circumstances. However, this library provides an alternative.
import numpy as np
import fastremap
mappings = {
1: 100,
2: 200,
-3: 7,
}
arr = np.array([5, 1, 2, -5, -3, 10, 6])
# Custom remapping of -3, 5, and 6 leaving the rest alone
arr = fastremap.remap(arr, mappings, preserve_missing_labels=True)
# result: [ 5, 100, 200, -5, 7, 10, 6 ]
Sometimes a 64-bit array contains values that could be represented by an 8-bit array. However, similarly to the remapping problem, Python loops can be too slow to do this. Numpy doesn't provide a convenient way to do it either. Therefore this library provides an alternative solution.
import fastremap
import numpy as np
arr = np.array([ 283732875, 439238823, 283732875, 182812404, 0 ], dtype=np.int64)
arr, remapping = fastremap.renumber(arr, preserve_zero=True) # Returns uint8 array
>>> arr = [ 1, 2, 1, 3, 0 ]
>>> remapping = { 0: 0, 283732875: 1, 439238823: 2, 182812404: 3 }
arr, remapping = fastremap.renumber(arr, preserve_zero=False) # Returns uint8 array
>>> arr = [ 1, 2, 1, 3, 4 ]
>>> remapping = { 0: 4, 283732875: 1, 439238823: 2, 182812404: 3 }
arr, remapping = fastremap.renumber(arr, preserve_zero=False, in_place=True) # Mutate arr to use less memory
>>> arr = [ 1, 2, 1, 3, 4 ]
>>> remapping = { 0: 4, 283732875: 1, 439238823: 2, 182812404: 3 }
When transitioning between different media, e.g. CPU to GPU, CPU to Network, CPU to disk, it's often necessary to physically transpose multi-dimensional arrays to reformat as C or Fortran order. Tranposing matrices is also a common action in linear algebra, but often you can get away with just changing the strides.
An out-of-place transposition is easy to write, and often faster, but it will spike peak memory consumption. This library grants the user the option of performing an in-place transposition which trades CPU time for peak memory usage. In the special case of square or cubic arrays, the in-place transpisition is both lower memory and faster.
import fastremap
import numpy as np
arr = np.ones((512,512,512), dtype=np.float32)
arr = fastremap.asfortranarray(x)
arr = np.ones((512,512,512), dtype=np.float32, order='F')
arr = fastremap.ascontiguousarray(x)
The in-place matrix transposition is implemented in ipt.hpp. If you're working in C++, you can also use it directly like so:
#include "ipt.hpp"
int main() {
int sx = 128;
int sy = 124;
int sz = 103;
int sw = 3;
auto* arr = ....;
// All primitive number types supported
// The array will be modified in place,
// so these functions are void type.
ipt::ipt<int>(arr, sx, sy); // 2D
ipt::ipt<float>(arr, sx, sy, sz); // 3D
ipt::ipt<double>(arr, sx, sy, sz, sw); // 4D
return 0;
}
--
Made with <3
FAQs
Remap, mask, renumber, unique, and in-place transposition of 3D labeled images. Point cloud too.
We found that fastremap demonstrated a healthy version release cadence and project activity because the last version was released less than a year ago. It has 1 open source maintainer collaborating on the project.
Did you know?
Socket for GitHub automatically highlights issues in each pull request and monitors the health of all your open source dependencies. Discover the contents of your packages and block harmful activity before you install or update your dependencies.
Security News
Crates.io adds Trusted Publishing support, enabling secure GitHub Actions-based crate releases without long-lived API tokens.
Research
/Security News
Undocumented protestware found in 28 npm packages disrupts UI for Russian-language users visiting Russian and Belarusian domains.
Research
/Security News
North Korean threat actors deploy 67 malicious npm packages using the newly discovered XORIndex malware loader.