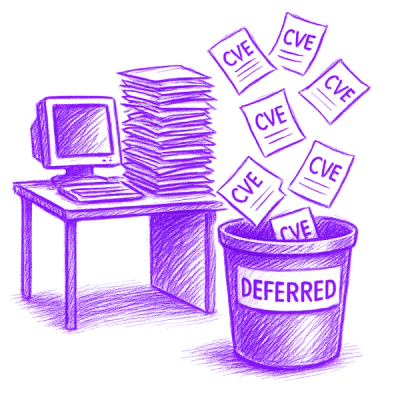
Security News
NVD Quietly Sweeps 100K+ CVEs Into a “Deferred” Black Hole
NVD now marks all pre-2018 CVEs as "Deferred," signaling it will no longer enrich older vulnerabilities, further eroding trust in its data.
|Build Status| |Coverage Status| |Python 3| |PyPI| |License: MIT|
featureflow is a python library that allows users to build feature extraction pipelines in a declarative way, and control how and where those features are persisted.
The following example will compute word frequency in individual text documents, and then over the entire corpus of documents, but featureflow isn't limited to text data. It's designed to work well with sequential/streaming data (e.g. audio or video) that is often processed iteratively, in small chunks.
You can see all the code in this example in one place here <https://github.com/JohnVinyard/featureflow/tree/master/examples/wordcount.py>
__.
We can define a graph of processing nodes like this:
.. code:: python
import featureflow as ff
@ff.simple_in_memory_settings
class Document(ff.BaseModel):
"""
Define the processing graph needed to extract document-level features,
whether, and how those features should be persisted.
"""
raw = ff.ByteStreamFeature(
ff.ByteStream,
chunksize=128,
store=True)
checksum = ff.JSONFeature(
CheckSum,
needs=raw,
store=True)
tokens = ff.Feature(
Tokenizer,
needs=raw,
store=False)
counts = ff.JSONFeature(
WordCount,
needs=tokens,
store=True)
We can define the individual processing "nodes" referenced in the graph above like this:
.. code:: python
import featureflow as ff
from collections import Counter
import re
import hashlib
class Tokenizer(ff.Node):
"""
Tokenize a stream of text into individual, normalized (lowercase)
words/tokens
"""
def __init__(self, needs=None):
super(Tokenizer, self).__init__(needs=needs)
self._cache = ''
self._pattern = re.compile('(?P<word>[a-zA-Z]+)\W+')
def _enqueue(self, data, pusher):
self._cache += data.decode()
def _dequeue(self):
matches = list(self._pattern.finditer(self._cache))
if not matches:
raise ff.NotEnoughData()
last_boundary = matches[-1].end()
self._cache = self._cache[last_boundary:]
return matches
def _process(self, data):
yield map(lambda x: x.groupdict()['word'].lower(), data)
class WordCount(ff.Aggregator, ff.Node):
"""
Keep track of token frequency
"""
def __init__(self, needs=None):
super(WordCount, self).__init__(needs=needs)
self._cache = Counter()
def _enqueue(self, data, pusher):
self._cache.update(data)
class CheckSum(ff.Aggregator, ff.Node):
"""
Compute the checksum of a text stream
"""
def __init__(self, needs=None):
super(CheckSum, self).__init__(needs=needs)
self._cache = hashlib.sha256()
def _enqueue(self, data, pusher):
self._cache.update(data)
def _process(self, data):
yield data.hexdigest()
We can also define a graph that will process an entire corpus of stored document features:
.. code:: python
import featureflow as ff
@ff.simple_in_memory_settings
class Corpus(ff.BaseModel):
"""
Define the processing graph needed to extract corpus-level features,
whether, and how those features should be persisted.
"""
docs = ff.Feature(
lambda doc_cls: (doc.counts for doc in doc_cls),
store=False)
total_counts = ff.JSONFeature(
WordCount,
needs=docs,
store=True)
Finally, we can execute these processing graphs and access the stored features like this:
.. code:: python
from __future__ import print_function
import argparse
def process_urls(urls):
for url in urls:
Document.process(raw=url)
def summarize_document(doc):
return 'doc {_id} with checksum {cs} contains "the" {n} times'.format(
_id=doc._id,
cs=doc.checksum,
n=doc.counts.get('the', 0))
def process_corpus(document_cls):
corpus_id = Corpus.process(docs=document_cls)
return Corpus(corpus_id)
def summarize_corpus(corpus):
return 'The entire text corpus contains "the" {n} times'.format(
n=corpus.total_counts.get("the", 0))
if __name__ == '__main__':
parser = argparse.ArgumentParser()
parser.add_argument(
'--url',
help='specify one or more urls of text files to ingest',
required=True,
action='append')
args = parser.parse_args()
process_urls(args.url)
for doc in Document:
print(summarize_document(doc))
corpus = process_corpus(Document)
print(summarize_corpus(corpus))
To see this in action we can:
.. code:: bash
python wordcount.py \
--url http://textfiles.com/food/1st_aid.txt \
--url http://textfiles.com/food/antibiot.txt \
...
Python headers are required. You can install by running:
.. code:: bash
apt-get install python-dev
Numpy is optional. If you'd like to use it, the
Anaconda <https://www.continuum.io/downloads>
__ distribution is highly
recommended.
Finally, just
.. code:: bash
pip install featureflow
.. |Build Status| image:: https://travis-ci.org/JohnVinyard/featureflow.svg?branch=master :target: https://travis-ci.org/JohnVinyard/featureflow .. |Coverage Status| image:: https://coveralls.io/repos/github/JohnVinyard/featureflow/badge.svg?branch=master :target: https://coveralls.io/github/JohnVinyard/featureflow?branch=master .. |Python 3| image:: https://img.shields.io/pypi/pyversions/featureflow.svg .. |PyPI| image:: https://img.shields.io/pypi/v/featureflow.svg :target: https://pypi.python.org/pypi/featureflow .. |License: MIT| image:: https://img.shields.io/badge/License-MIT-yellow.svg :target: https://opensource.org/licenses/MIT
FAQs
Unknown package
We found that featureflow demonstrated a healthy version release cadence and project activity because the last version was released less than a year ago. It has 1 open source maintainer collaborating on the project.
Did you know?
Socket for GitHub automatically highlights issues in each pull request and monitors the health of all your open source dependencies. Discover the contents of your packages and block harmful activity before you install or update your dependencies.
Security News
NVD now marks all pre-2018 CVEs as "Deferred," signaling it will no longer enrich older vulnerabilities, further eroding trust in its data.
Research
Security News
Lazarus-linked threat actors expand their npm malware campaign with new RAT loaders, hex obfuscation, and over 5,600 downloads across 11 packages.
Security News
Safari 18.4 adds support for Iterator Helpers and two other TC39 JavaScript features, bringing full cross-browser coverage to key parts of the ECMAScript spec.