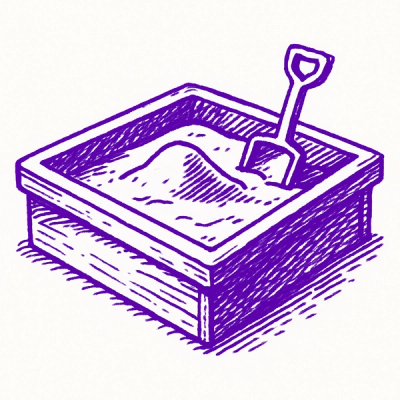
Research
/Security News
Critical Vulnerability in NestJS Devtools: Localhost RCE via Sandbox Escape
A flawed sandbox in @nestjs/devtools-integration lets attackers run code on your machine via CSRF, leading to full Remote Code Execution (RCE).
The Fixess Predictive Database returns an AI prediction instead of raising a KeyError for missing keys. This is a Python library that interacts with the fixess cloud database to provide cloud GPU accelerated predictions for missing data.
Get an X-RapidAPI-Key from https://rapidapi.com/fixessgithub/api/fixess
To start using this package, you need to pass in your Rapid API headers (API Key) to create a fixess
dictionary client:
import fixess
client = fixess.Fixess(api_key=YOUR_API_KEY)
Replace YOUR_API_KEY
with your actual Rapid API Key.
Once you have created a client object, you can use it like a Python dictionary. For example:
value = client['missing-key']
print(value)
# Or with a more complex key
value = client[{'key' : 'value'}]
print(value)
In the above code snippet, missing-key
and {'key' : 'value'}
are keys absent in the database. Still, instead of raising a KeyError, the Fixess Predictive Database provides a sophisticated AI prediction.
The fixess API will automatically serialize (pickle) your dictionary keys, allowing you to use complex types as keys.
Please make sure to use the client object like a python dictionary, as shown in the example above.
Enjoy using Fixess Predictive Database!
For more information, visit our official documentation or submit an issue on our Github page.
An important note for users considering storing keys from multiple users in a single Fixess predictive database:
Each Fixess predictive database is backed by a single AI model. This has implications for the privacy and isolation of data.
When storing sensitive data from multiple users in a single database, it's possible for the AI model to share information inferred from this data between users during the prediction process. This is a byproduct of how the AI model learns patterns across all the data it has been given access to.
Please note, this does not imply that data will be explicitly shared between users nor does it mean that data will be shared between distinct databases or between distinct AI models.
While the raw data itself always remains strictly isolated (the database will never show one user's data to another user), the AI model's predictions can inevitably be influenced by the data from all users.
Therefore, if stringent data isolation is a requirement, we recommend against storing sensitive data from different users in the same predictive database. Consider creating separate databases for each user or thoroughly anonymizing data prior to insertion.
Always be attentive towards user privacy and respectful of all relevant data protection laws and best practices when using Fixess predictive databases.
FAQs
Fixess cloud AI database client for Python
We found that fixess demonstrated a healthy version release cadence and project activity because the last version was released less than a year ago. It has 1 open source maintainer collaborating on the project.
Did you know?
Socket for GitHub automatically highlights issues in each pull request and monitors the health of all your open source dependencies. Discover the contents of your packages and block harmful activity before you install or update your dependencies.
Research
/Security News
A flawed sandbox in @nestjs/devtools-integration lets attackers run code on your machine via CSRF, leading to full Remote Code Execution (RCE).
Product
Customize license detection with Socket’s new license overlays: gain control, reduce noise, and handle edge cases with precision.
Product
Socket now supports Rust and Cargo, offering package search for all users and experimental SBOM generation for enterprise projects.