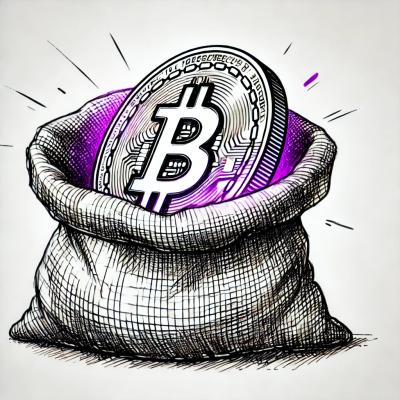
Research
Security News
Malicious npm Packages Use Telegram to Exfiltrate BullX Credentials
Socket uncovers an npm Trojan stealing crypto wallets and BullX credentials via obfuscated code and Telegram exfiltration.
.. image:: https://api.travis-ci.org/vi3k6i5/flashtext.svg?branch=master :target: https://travis-ci.org/vi3k6i5/flashtext :alt: Build Status
.. image:: https://readthedocs.org/projects/flashtext/badge/?version=latest :target: http://flashtext.readthedocs.io/en/latest/?badge=latest :alt: Documentation Status
.. image:: https://badge.fury.io/py/flashtext.svg :target: https://badge.fury.io/py/flashtext :alt: Version
.. image:: https://coveralls.io/repos/github/vi3k6i5/flashtext/badge.svg?branch=master :target: https://coveralls.io/github/vi3k6i5/flashtext?branch=master :alt: Test coverage
.. image:: https://img.shields.io/github/license/mashape/apistatus.svg?maxAge=2592000 :target: https://github.com/vi3k6i5/flashtext/blob/master/LICENSE :alt: license
This module can be used to replace keywords in sentences or extract keywords from sentences. It is based on the FlashText algorithm <https://arxiv.org/abs/1711.00046>
_.
::
$ pip install flashtext
Documentation can be found at FlashText Read the Docs <http://flashtext.readthedocs.io/>
_.
Extract keywords >>> from flashtext import KeywordProcessor >>> keyword_processor = KeywordProcessor() >>> # keyword_processor.add_keyword(, ) >>> keyword_processor.add_keyword('Big Apple', 'New York') >>> keyword_processor.add_keyword('Bay Area') >>> keywords_found = keyword_processor.extract_keywords('I love Big Apple and Bay Area.') >>> keywords_found >>> # ['New York', 'Bay Area']
Replace keywords >>> keyword_processor.add_keyword('New Delhi', 'NCR region') >>> new_sentence = keyword_processor.replace_keywords('I love Big Apple and new delhi.') >>> new_sentence >>> # 'I love New York and NCR region.'
Case Sensitive example >>> from flashtext import KeywordProcessor >>> keyword_processor = KeywordProcessor(case_sensitive=True) >>> keyword_processor.add_keyword('Big Apple', 'New York') >>> keyword_processor.add_keyword('Bay Area') >>> keywords_found = keyword_processor.extract_keywords('I love big Apple and Bay Area.') >>> keywords_found >>> # ['Bay Area']
Span of keywords extracted >>> from flashtext import KeywordProcessor >>> keyword_processor = KeywordProcessor() >>> keyword_processor.add_keyword('Big Apple', 'New York') >>> keyword_processor.add_keyword('Bay Area') >>> keywords_found = keyword_processor.extract_keywords('I love big Apple and Bay Area.', span_info=True) >>> keywords_found >>> # [('New York', 7, 16), ('Bay Area', 21, 29)]
Get Extra information with keywords extracted >>> from flashtext import KeywordProcessor >>> kp = KeywordProcessor() >>> kp.add_keyword('Taj Mahal', ('Monument', 'Taj Mahal')) >>> kp.add_keyword('Delhi', ('Location', 'Delhi')) >>> kp.extract_keywords('Taj Mahal is in Delhi.') >>> # [('Monument', 'Taj Mahal'), ('Location', 'Delhi')] >>> # NOTE: replace_keywords feature won't work with this.
No clean name for Keywords >>> from flashtext import KeywordProcessor >>> keyword_processor = KeywordProcessor() >>> keyword_processor.add_keyword('Big Apple') >>> keyword_processor.add_keyword('Bay Area') >>> keywords_found = keyword_processor.extract_keywords('I love big Apple and Bay Area.') >>> keywords_found >>> # ['Big Apple', 'Bay Area']
Add Multiple Keywords simultaneously >>> from flashtext import KeywordProcessor >>> keyword_processor = KeywordProcessor() >>> keyword_dict = { >>> "java": ["java_2e", "java programing"], >>> "product management": ["PM", "product manager"] >>> } >>> # {'clean_name': ['list of unclean names']} >>> keyword_processor.add_keywords_from_dict(keyword_dict) >>> # Or add keywords from a list: >>> keyword_processor.add_keywords_from_list(["java", "python"]) >>> keyword_processor.extract_keywords('I am a product manager for a java_2e platform') >>> # output ['product management', 'java']
To Remove keywords >>> from flashtext import KeywordProcessor >>> keyword_processor = KeywordProcessor() >>> keyword_dict = { >>> "java": ["java_2e", "java programing"], >>> "product management": ["PM", "product manager"] >>> } >>> keyword_processor.add_keywords_from_dict(keyword_dict) >>> print(keyword_processor.extract_keywords('I am a product manager for a java_2e platform')) >>> # output ['product management', 'java'] >>> keyword_processor.remove_keyword('java_2e') >>> # you can also remove keywords from a list/ dictionary >>> keyword_processor.remove_keywords_from_dict({"product management": ["PM"]}) >>> keyword_processor.remove_keywords_from_list(["java programing"]) >>> keyword_processor.extract_keywords('I am a product manager for a java_2e platform') >>> # output ['product management']
To check Number of terms in KeywordProcessor >>> from flashtext import KeywordProcessor >>> keyword_processor = KeywordProcessor() >>> keyword_dict = { >>> "java": ["java_2e", "java programing"], >>> "product management": ["PM", "product manager"] >>> } >>> keyword_processor.add_keywords_from_dict(keyword_dict) >>> print(len(keyword_processor)) >>> # output 4
To check if term is present in KeywordProcessor >>> from flashtext import KeywordProcessor >>> keyword_processor = KeywordProcessor() >>> keyword_processor.add_keyword('j2ee', 'Java') >>> 'j2ee' in keyword_processor >>> # output: True >>> keyword_processor.get_keyword('j2ee') >>> # output: Java >>> keyword_processor['colour'] = 'color' >>> keyword_processor['colour'] >>> # output: color
Get all keywords in dictionary >>> from flashtext import KeywordProcessor >>> keyword_processor = KeywordProcessor() >>> keyword_processor.add_keyword('j2ee', 'Java') >>> keyword_processor.add_keyword('colour', 'color') >>> keyword_processor.get_all_keywords() >>> # output: {'colour': 'color', 'j2ee': 'Java'}
For detecting Word Boundary currently any character other than this \\w
[A-Za-z0-9_]
is considered a word boundary.
To set or add characters as part of word characters >>> from flashtext import KeywordProcessor >>> keyword_processor = KeywordProcessor() >>> keyword_processor.add_keyword('Big Apple') >>> print(keyword_processor.extract_keywords('I love Big Apple/Bay Area.')) >>> # ['Big Apple'] >>> keyword_processor.add_non_word_boundary('/') >>> print(keyword_processor.extract_keywords('I love Big Apple/Bay Area.')) >>> # []
::
$ git clone https://github.com/vi3k6i5/flashtext
$ cd flashtext
$ pip install pytest
$ python setup.py test
::
$ git clone https://github.com/vi3k6i5/flashtext
$ cd flashtext/docs
$ pip install sphinx
$ make html
$ # open _build/html/index.html in browser to view it locally
It's a custom algorithm based on Aho-Corasick algorithm <https://en.wikipedia.org/wiki/Aho%E2%80%93Corasick_algorithm>
_ and Trie Dictionary <https://en.wikipedia.org/wiki/Trie Dictionary>
_.
.. image:: https://github.com/vi3k6i5/flashtext/raw/master/benchmark.png :target: https://twitter.com/RadimRehurek/status/904989624589803520 :alt: Benchmark
Time taken by FlashText to find terms in comparison to Regex.
.. image:: https://thepracticaldev.s3.amazonaws.com/i/xruf50n6z1r37ti8rd89.png
Time taken by FlashText to replace terms in comparison to Regex.
.. image:: https://thepracticaldev.s3.amazonaws.com/i/k44ghwp8o712dm58debj.png
Link to code for benchmarking the Find Feature <https://gist.github.com/vi3k6i5/604eefd92866d081cfa19f862224e4a0>
_ and Replace Feature <https://gist.github.com/vi3k6i5/dc3335ee46ab9f650b19885e8ade6c7a>
_.
The idea for this library came from the following StackOverflow question <https://stackoverflow.com/questions/44178449/regex-replace-is-taking-time-for-millions-of-documents-how-to-make-it-faster>
_.
The original paper published on FlashText algorithm <https://arxiv.org/abs/1711.00046>
_.
::
@ARTICLE{2017arXiv171100046S,
author = {{Singh}, V.},
title = "{Replace or Retrieve Keywords In Documents at Scale}",
journal = {ArXiv e-prints},
archivePrefix = "arXiv",
eprint = {1711.00046},
primaryClass = "cs.DS",
keywords = {Computer Science - Data Structures and Algorithms},
year = 2017,
month = oct,
adsurl = {http://adsabs.harvard.edu/abs/2017arXiv171100046S},
adsnote = {Provided by the SAO/NASA Astrophysics Data System}
}
The article published on Medium freeCodeCamp <https://medium.freecodecamp.org/regex-was-taking-5-days-flashtext-does-it-in-15-minutes-55f04411025f>
_.
The project is licensed under the MIT license.
FAQs
Extract/Replaces keywords in sentences.
We found that flashtext demonstrated a healthy version release cadence and project activity because the last version was released less than a year ago. It has 1 open source maintainer collaborating on the project.
Did you know?
Socket for GitHub automatically highlights issues in each pull request and monitors the health of all your open source dependencies. Discover the contents of your packages and block harmful activity before you install or update your dependencies.
Research
Security News
Socket uncovers an npm Trojan stealing crypto wallets and BullX credentials via obfuscated code and Telegram exfiltration.
Research
Security News
Malicious npm packages posing as developer tools target macOS Cursor IDE users, stealing credentials and modifying files to gain persistent backdoor access.
Security News
AI-generated slop reports are making bug bounty triage harder, wasting maintainer time, and straining trust in vulnerability disclosure programs.