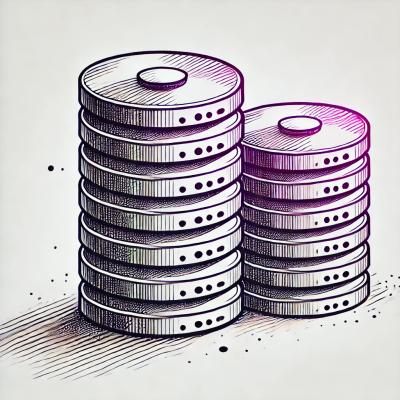
Security News
MCP Community Begins Work on Official MCP Metaregistry
The MCP community is launching an official registry to standardize AI tool discovery and let agents dynamically find and install MCP servers.
GAPandas4 is a Python package for accessing the Google Analytics Data API for GA4 using Pandas
GAPandas4 is a Python package for querying the Google Analytics Data API for GA4 and displaying the results in a Pandas dataframe. It is the successor to the GAPandas package, which did the same thing for GA3 or Universal Analytics. GAPandas4 is a wrapper around the official Google Analytics Data API package and simplifies imports and queries, requiring far less code.
In order to use GAPandas4 you will first need to create a Google Service Account with access to the Google Analytics Data API and export a client secrets JSON keyfile to use for authentication. You'll also need to add the service account email address as a user on the Google Analytics 4 property you wish to access, and you'll need to note the property ID to use in your queries.
As this is currently in alpha, there's currently no Pip package, however, you can install the code into your Python environment directly from GitHub using the command below. It will run fine in a Jupyter notebook, a Python IDE, or a Python script.
pip3 install git+https://github.com/practical-data-science/gapandas4.git
GAPandas4 has been written to allow you to use as little code as possible. Unlike the previous version of GAPandas for Universal Analytics, which used a payload based on a Python dictionary, GAPandas4 now uses a Protobuf (Protocol Buffer) payload as used in the API itself.
The query()
function is used to send a protobug API payload to the API. The function supports various report types
via the report_type
argument. Standard reports are handled using report_type="report"
, but this is also the
default. Data are returned as a Pandas dataframe.
import gapandas4 as gp
service_account = 'client_secrets.json'
property_id = 'xxxxxxxxx'
report_request = gp.RunReportRequest(
property=f"properties/{property_id}",
dimensions=[
gp.Dimension(name="country"),
gp.Dimension(name="city")
],
metrics=[
gp.Metric(name="activeUsers")
],
date_ranges=[gp.DateRange(start_date="2022-06-01", end_date="2022-06-01")],
)
df = gp.query(service_account, report_request, report_type="report")
print(df.head())
If you construct a protobuf payload using BatchRunReportsRequest()
you can pass up to five requests at once. These
are returned as a list of Pandas dataframes, so will need to access them using their index.
import gapandas4 as gp
service_account = 'client_secrets.json'
property_id = 'xxxxxxxxx'
batch_report_request = gp.BatchRunReportsRequest(
property=f"properties/{property_id}",
requests=[
gp.RunReportRequest(
dimensions=[
gp.Dimension(name="country"),
gp.Dimension(name="city")
],
metrics=[
gp.Metric(name="activeUsers")
],
date_ranges=[gp.DateRange(start_date="2022-06-01", end_date="2022-06-01")]
),
gp.RunReportRequest(
dimensions=[
gp.Dimension(name="country"),
gp.Dimension(name="city")
],
metrics=[
gp.Metric(name="activeUsers")
],
date_ranges=[gp.DateRange(start_date="2022-06-02", end_date="2022-06-02")]
)
]
)
df = gp.query(service_account, batch_report_request, report_type="batch_report")
print(df[0].head())
print(df[1].head())
Constructing a report using RunPivotReportRequest()
will return pivoted data in a single Pandas dataframe.
import gapandas4 as gp
service_account = 'client_secrets.json'
property_id = 'xxxxxxxxx'
pivot_request = gp.RunPivotReportRequest(
property=f"properties/{property_id}",
dimensions=[gp.Dimension(name="country"),
gp.Dimension(name="browser")],
metrics=[gp.Metric(name="sessions")],
date_ranges=[gp.DateRange(start_date="2022-05-30", end_date="today")],
pivots=[
gp.Pivot(
field_names=["country"],
limit=5,
order_bys=[
gp.OrderBy(
dimension=gp.OrderBy.DimensionOrderBy(dimension_name="country")
)
],
),
gp.Pivot(
field_names=["browser"],
offset=0,
limit=5,
order_bys=[
gp.OrderBy(
metric=gp.OrderBy.MetricOrderBy(metric_name="sessions"), desc=True
)
],
),
],
)
df = gp.query(service_account, pivot_request, report_type="pivot")
print(df.head())
Constructing a payload using BatchRunPivotReportsRequest()
will allow you to run up to five pivot reports. These
are returned as a list of Pandas dataframes.
import gapandas4 as gp
service_account = 'client_secrets.json'
property_id = 'xxxxxxxxx'
batch_pivot_request = gp.BatchRunPivotReportsRequest(
property=f"properties/{property_id}",
requests=[
gp.RunPivotReportRequest(
dimensions=[gp.Dimension(name="country"),
gp.Dimension(name="browser")],
metrics=[gp.Metric(name="sessions")],
date_ranges=[gp.DateRange(start_date="2022-05-30", end_date="today")],
pivots=[
gp.Pivot(
field_names=["country"],
limit=5,
order_bys=[
gp.OrderBy(
dimension=gp.OrderBy.DimensionOrderBy(dimension_name="country")
)
],
),
gp.Pivot(
field_names=["browser"],
offset=0,
limit=5,
order_bys=[
gp.OrderBy(
metric=gp.OrderBy.MetricOrderBy(metric_name="sessions"), desc=True
)
],
),
],
),
gp.RunPivotReportRequest(
dimensions=[gp.Dimension(name="country"),
gp.Dimension(name="browser")],
metrics=[gp.Metric(name="sessions")],
date_ranges=[gp.DateRange(start_date="2022-05-30", end_date="today")],
pivots=[
gp.Pivot(
field_names=["country"],
limit=5,
order_bys=[
gp.OrderBy(
dimension=gp.OrderBy.DimensionOrderBy(dimension_name="country")
)
],
),
gp.Pivot(
field_names=["browser"],
offset=0,
limit=5,
order_bys=[
gp.OrderBy(
metric=gp.OrderBy.MetricOrderBy(metric_name="sessions"), desc=True
)
],
),
],
)
]
)
df = gp.query(service_account, batch_pivot_request, report_type="batch_pivot")
print(df[0].head())
print(df[1].head())
The get_metadata()
function will return all metadata on dimensions and metrics within the Google Analytics 4 property.
metadata = gp.get_metadata(service_account, property_id)
print(metadata)
RunReportRequest
, BatchRunReportsRequest
,
RunPivotReportRequest
, BatchRunPivotReportsRequest
, RunRealtimeReportRequest
, and GetMetadataRequest
.FAQs
GAPandas4 is a Python package for accessing the Google Analytics Data API for GA4 using Pandas
We found that gapandas4 demonstrated a healthy version release cadence and project activity because the last version was released less than a year ago. It has 1 open source maintainer collaborating on the project.
Did you know?
Socket for GitHub automatically highlights issues in each pull request and monitors the health of all your open source dependencies. Discover the contents of your packages and block harmful activity before you install or update your dependencies.
Security News
The MCP community is launching an official registry to standardize AI tool discovery and let agents dynamically find and install MCP servers.
Research
Security News
Socket uncovers an npm Trojan stealing crypto wallets and BullX credentials via obfuscated code and Telegram exfiltration.
Research
Security News
Malicious npm packages posing as developer tools target macOS Cursor IDE users, stealing credentials and modifying files to gain persistent backdoor access.