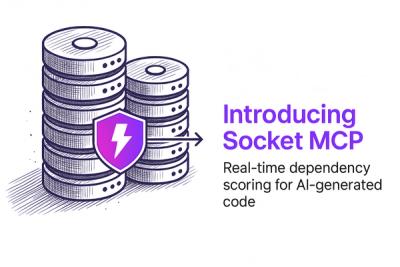
Product
Secure Your AI-Generated Code with Socket MCP
Socket MCP brings real-time security checks to AI-generated code, helping developers catch risky dependencies before they enter the codebase.
A lightweight multi-stage grid search AutoML framework for classifiers. GridMaster v0.2.0: Log-scale search + plot customization features
GridMaster is a Python module built for data scientists who want flexible and interpretable model selection and tuning on structured classification datasets. It automates the hyperparameter tuning process using coarse-to-fine grid search and supports model evaluation, visualization, export, and reproducibility.
⚠️ GridMaster is currently designed for classification tasks only, and does not yet support regressors.
Model Name | Backend Module | Notes |
---|---|---|
Logistic Regression | sklearn.linear_model | Great interpretability via coefficients |
Random Forest | sklearn.ensemble | Robust baseline, provides feature importance |
XGBoost | xgboost | Highly accurate, supports regularization |
LightGBM | lightgbm | Fast, efficient gradient boosting |
CatBoost | catboost | Handles categorical features natively |
❌
DecisionTreeClassifier
is intentionally excluded due to its high variance and limited generalization ability. Use ensemble variants instead.
pip install scikit-learn xgboost lightgbm catboost pandas numpy matplotlib joblib
Then clone the repository:
git clone https://github.com/wins-wang/GridMaster.git
from gridmaster import GridMaster
gm = GridMaster(models=[...], X_train=..., y_train=...)
You can then call:
gm.coarse_search(...)
gm.fine_search(...)
gm.multi_stage_search(...)
gm.compare_best_models(...)
All with built-in output redirection for noisy models.
gm.multi_stage_search("xgboost", scoring="roc_auc")
gm.compare_best_models(X_test, y_test, metrics=["f1", "roc_auc"])
gm.plot_cv_score_curve("xgboost")
gm.export_model_package("xgboost")
Use suppress_output=False
if you want to see model logs.
# demo_usage.ipynb
from gridmaster import GridMaster
from sklearn.datasets import load_breast_cancer
from sklearn.model_selection import train_test_split
from sklearn.metrics import classification_report
import pandas as pd
import os
import warnings
warnings.filterwarnings("ignore")
X, y = load_breast_cancer(return_X_y=True)
X_train, X_test, y_train, y_test = train_test_split(X, y, test_size=0.2, random_state=42)
gm = GridMaster(
models=["logistic", "random_forest", "xgboost", "lightgbm", "catboost"],
X_train=X_train,
y_train=y_train
)
for model in ["logistic", "random_forest", "xgboost", "lightgbm", "catboost"]:
gm.multi_stage_search(model, scoring="f1")
best_model, scores = gm.compare_best_models(X_test, y_test, metrics=["accuracy", "f1", "roc_auc"])
print("Best model:", best_model)
print(scores)
# Visualize all
for model in ["logistic", "random_forest", "xgboost", "lightgbm", "catboost"]:
gm.plot_cv_score_curve(model)
gm.plot_confusion_matrix(model, X_test, y_test)
if model == "logistic":
gm.plot_model_coefficients(model)
else:
gm.plot_feature_importance(model)
# Export and reload
os.makedirs("outputs", exist_ok=True)
gm.export_all_models(folder_path="outputs")
gm.import_all_models(folder_path="outputs")
# Final report
final_model = gm.results[best_model]["best_model"]
X_test_df = pd.DataFrame(X_test, columns=X_train.columns)
y_pred = final_model.predict(X_test_df)
print(classification_report(y_test, y_pred))
MIT License. See LICENSE
.
FAQs
A lightweight multi-stage grid search AutoML framework for classifiers. GridMaster v0.2.0: Log-scale search + plot customization features
We found that gridmaster demonstrated a healthy version release cadence and project activity because the last version was released less than a year ago. It has 1 open source maintainer collaborating on the project.
Did you know?
Socket for GitHub automatically highlights issues in each pull request and monitors the health of all your open source dependencies. Discover the contents of your packages and block harmful activity before you install or update your dependencies.
Product
Socket MCP brings real-time security checks to AI-generated code, helping developers catch risky dependencies before they enter the codebase.
Security News
As vulnerability data bottlenecks grow, the federal government is formally investigating NIST’s handling of the National Vulnerability Database.
Research
Security News
Socket’s Threat Research Team has uncovered 60 npm packages using post-install scripts to silently exfiltrate hostnames, IP addresses, DNS servers, and user directories to a Discord-controlled endpoint.