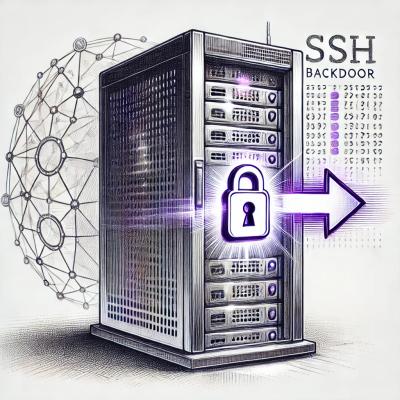
Research
Security News
Malicious npm Packages Inject SSH Backdoors via Typosquatted Libraries
Socket’s threat research team has detected six malicious npm packages typosquatting popular libraries to insert SSH backdoors.
Holistic AI is an open-source library dedicated to assessing and improving the trustworthiness of AI systems. We believe that responsible AI development requires a comprehensive evaluation across multiple dimensions, beyond just accuracy.
Holistic AI currently focuses on five verticals of AI trustworthiness:
pip install holisticai # Basic installation
pip install holisticai[bias] # Bias mitigation support
pip install holisticai[explainability] # For explainability metrics and plots
pip install holisticai[all] # Install all packages for bias and explainability
# imports
from holisticai.bias.metrics import classification_bias_metrics
from holisticai.datasets import load_dataset
from holisticai.bias.plots import bias_metrics_report
from sklearn.linear_model import LogisticRegression
from sklearn.preprocessing import StandardScaler
# load an example dataset and split
dataset = load_dataset('law_school', protected_attribute="race")
dataset_split = dataset.train_test_split(test_size=0.3)
# separate the data into train and test sets
train_data = dataset_split['train']
test_data = dataset_split['test']
# rescale the data
scaler = StandardScaler()
X_train_t = scaler.fit_transform(train_data['X'])
X_test_t = scaler.transform(test_data['X'])
# train a logistic regression model
model = LogisticRegression(random_state=42, max_iter=500)
model.fit(X_train_t, train_data['y'])
# make predictions
y_pred = model.predict(X_test_t)
# compute bias metrics
metrics = classification_bias_metrics(
group_a = test_data['group_a'],
group_b = test_data['group_b'],
y_true = test_data['y'],
y_pred = y_pred
)
# create a comprehensive report
bias_metrics_report(model_type='binary_classification', table_metrics=metrics)
Troubleshooting (macOS):
Before installing the library, you may need to install these packages:
brew install cbc pkg-config
python -m pip install cylp
brew install cmake
We welcome contributions from the community To learn more about contributing to Holistic AI, please refer to our Contributing Guide.
FAQs
Unknown package
We found that holisticai demonstrated a healthy version release cadence and project activity because the last version was released less than a year ago. It has 4 open source maintainers collaborating on the project.
Did you know?
Socket for GitHub automatically highlights issues in each pull request and monitors the health of all your open source dependencies. Discover the contents of your packages and block harmful activity before you install or update your dependencies.
Research
Security News
Socket’s threat research team has detected six malicious npm packages typosquatting popular libraries to insert SSH backdoors.
Security News
MITRE's 2024 CWE Top 25 highlights critical software vulnerabilities like XSS, SQL Injection, and CSRF, reflecting shifts due to a refined ranking methodology.
Security News
In this segment of the Risky Business podcast, Feross Aboukhadijeh and Patrick Gray discuss the challenges of tracking malware discovered in open source softare.