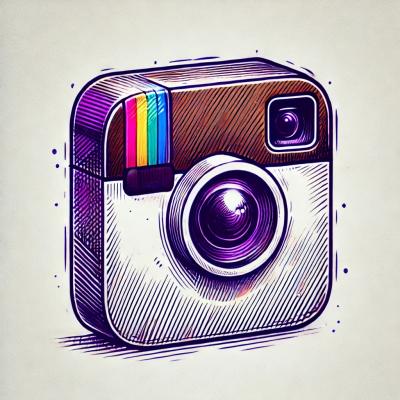
Research
PyPI Package Disguised as Instagram Growth Tool Harvests User Credentials
A deceptive PyPI package posing as an Instagram growth tool collects user credentials and sends them to third-party bot services.
HydraFlow seamlessly integrates Hydra and MLflow to streamline ML experiment management, combining Hydra's configuration management with MLflow's tracking capabilities.
HydraFlow seamlessly integrates Hydra and MLflow to streamline machine learning experiment workflows. By combining Hydra's powerful configuration management with MLflow's robust experiment tracking, HydraFlow provides a comprehensive solution for defining, executing, and analyzing machine learning experiments.
HydraFlow is built on the following design principles:
pip install hydraflow
Requirements: Python 3.13+
from dataclasses import dataclass
from mlflow.entities import Run
import hydraflow
@dataclass
class Config:
width: int = 1024
height: int = 768
@hydraflow.main(Config)
def app(run: Run, cfg: Config) -> None:
# Your experiment code here
print(f"Running with width={cfg.width}, height={cfg.height}")
if __name__ == "__main__":
app()
Execute a parameter sweep with:
python app.py -m width=800,1200 height=600,900
HydraFlow consists of the following key components:
Define type-safe configurations using Python dataclasses:
@dataclass
class Config:
learning_rate: float = 0.001
batch_size: int = 32
epochs: int = 10
The @hydraflow.main
decorator integrates Hydra and MLflow:
@hydraflow.main(Config)
def train(run: Run, cfg: Config) -> None:
# Your experiment code
Define reusable experiment workflows in YAML:
jobs:
train_models:
run: python train.py
sets:
- each: model=small,medium,large
all: learning_rate=0.001,0.01,0.1
Analyze experiment results with powerful APIs:
from hydraflow import Run, iter_run_dirs
# Load runs
runs = Run.load(iter_run_dirs("mlruns"))
# Filter and analyze
best_runs = runs.filter(model_type="transformer").to_frame("learning_rate", "accuracy")
For detailed documentation, visit our documentation site:
This project is licensed under the MIT License.
FAQs
HydraFlow seamlessly integrates Hydra and MLflow to streamline ML experiment management, combining Hydra's configuration management with MLflow's tracking capabilities.
We found that hydraflow demonstrated a healthy version release cadence and project activity because the last version was released less than a year ago. It has 1 open source maintainer collaborating on the project.
Did you know?
Socket for GitHub automatically highlights issues in each pull request and monitors the health of all your open source dependencies. Discover the contents of your packages and block harmful activity before you install or update your dependencies.
Research
A deceptive PyPI package posing as an Instagram growth tool collects user credentials and sends them to third-party bot services.
Product
Socket now supports pylock.toml, enabling secure, reproducible Python builds with advanced scanning and full alignment with PEP 751's new standard.
Security News
Research
Socket uncovered two npm packages that register hidden HTTP endpoints to delete all files on command.