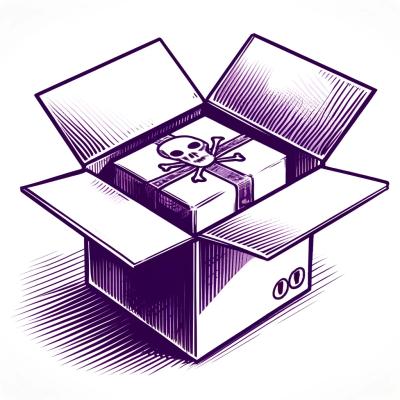
Security News
Research
Data Theft Repackaged: A Case Study in Malicious Wrapper Packages on npm
The Socket Research Team breaks down a malicious wrapper package that uses obfuscation to harvest credentials and exfiltrate sensitive data.
hyperparameter-tuning
Advanced tools
A minimal hyperparameter tuning framework to help you train hundreds of models.
It's essentially a set of helpful wrappers over optuna.
Consult the package documentation here!
Install package from PyPI:
pip install hyperparameter-tuning
from hpt.tuner import ObjectiveFunction, OptunaTuner
obj_func = ObjectiveFunction(
X_train, y_train, X_test, y_test,
hyperparameter_space=HYPERPARAM_SPACE_PATH, # path to YAML file
eval_metric="accuracy",
s_train=s_train,
s_val=s_test,
threshold=0.50,
)
tuner = OptunaTuner(
objective_function=obj_func,
direction="maximize", # NOTE: can pass other useful study kwargs here (e.g. storage)
)
# Then just run optimize as you would for an optuna.Study object
tuner.optimize(n_trials=20, n_jobs=4)
# Results are stored in tuner.results
tuner.results
# You can reconstruct the best predictor with:
clf = obj_func.reconstruct_model(obj_func.best_trial)
The hyperparameter space is provided either path to a YAML file, or as a dict
with the same structure.
Example hyperparameter spaces here.
The YAML file must follow this structure:
# One or more top-level algorithms
DT:
# Full classpath of algorithm's constructor
classpath: sklearn.tree.DecisionTreeClassifier
# One or more key-word arguments to be passed to the constructor
kwargs:
# Kwargs may be sampled from a distribution
max_depth:
type: int # either 'int' or 'float'
range: [ 10, 100 ] # minimum and maximum values
log: True # (optionally) whether to use logarithmic scale
# Kwargs may be sampled from a fixed set of categories
criterion:
- 'gini'
- 'entropy'
# Kwargs may be a pre-defined value
min_samples_split: 4
# You may explore multiple algorithms at once
LR:
classpath: sklearn.linear_model.LogisticRegression
kwargs:
# An example of a float hyperparameter
C:
type: float
range: [ 0.01, 1.0 ]
log: True
FAQs
A minimal framework for running hyperparameter tuning
We found that hyperparameter-tuning demonstrated a healthy version release cadence and project activity because the last version was released less than a year ago. It has 1 open source maintainer collaborating on the project.
Did you know?
Socket for GitHub automatically highlights issues in each pull request and monitors the health of all your open source dependencies. Discover the contents of your packages and block harmful activity before you install or update your dependencies.
Security News
Research
The Socket Research Team breaks down a malicious wrapper package that uses obfuscation to harvest credentials and exfiltrate sensitive data.
Research
Security News
Attackers used a malicious npm package typosquatting a popular ESLint plugin to steal sensitive data, execute commands, and exploit developer systems.
Security News
The Ultralytics' PyPI Package was compromised four times in one weekend through GitHub Actions cache poisoning and failure to rotate previously compromised API tokens.