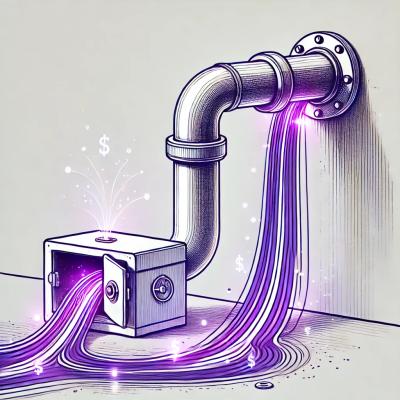
Research
Security News
Malicious npm Package Targets Solana Developers and Hijacks Funds
A malicious npm package targets Solana developers, rerouting funds in 2% of transactions to a hardcoded address.
Multimodal AI services & pipelines with cloud-native stack: gRPC, Kubernetes, Docker, OpenTelemetry, Prometheus, Jaeger, etc.
Jina-serve is a framework for building and deploying AI services that communicate via gRPC, HTTP and WebSockets. Scale your services from local development to production while focusing on your core logic.
Key advantages over FastAPI:
pip install jina
See guides for Apple Silicon and Windows.
Three main layers:
Let's create a gRPC-based AI service using StableLM:
from jina import Executor, requests
from docarray import DocList, BaseDoc
from transformers import pipeline
class Prompt(BaseDoc):
text: str
class Generation(BaseDoc):
prompt: str
text: str
class StableLM(Executor):
def __init__(self, **kwargs):
super().__init__(**kwargs)
self.generator = pipeline(
'text-generation', model='stabilityai/stablelm-base-alpha-3b'
)
@requests
def generate(self, docs: DocList[Prompt], **kwargs) -> DocList[Generation]:
generations = DocList[Generation]()
prompts = docs.text
llm_outputs = self.generator(prompts)
for prompt, output in zip(prompts, llm_outputs):
generations.append(Generation(prompt=prompt, text=output))
return generations
Deploy with Python or YAML:
from jina import Deployment
from executor import StableLM
dep = Deployment(uses=StableLM, timeout_ready=-1, port=12345)
with dep:
dep.block()
jtype: Deployment
with:
uses: StableLM
py_modules:
- executor.py
timeout_ready: -1
port: 12345
Use the client:
from jina import Client
from docarray import DocList
from executor import Prompt, Generation
prompt = Prompt(text='suggest an interesting image generation prompt')
client = Client(port=12345)
response = client.post('/', inputs=[prompt], return_type=DocList[Generation])
Chain services into a Flow:
from jina import Flow
flow = Flow(port=12345).add(uses=StableLM).add(uses=TextToImage)
with flow:
flow.block()
Boost throughput with built-in features:
Example scaling a Stable Diffusion deployment:
jtype: Deployment
with:
uses: TextToImage
timeout_ready: -1
py_modules:
- text_to_image.py
env:
CUDA_VISIBLE_DEVICES: RR
replicas: 2
uses_dynamic_batching:
/default:
preferred_batch_size: 10
timeout: 200
TextToImage/
├── executor.py
├── config.yml
├── requirements.txt
# config.yml
jtype: TextToImage
py_modules:
- executor.py
metas:
name: TextToImage
description: Text to Image generation Executor
jina hub push TextToImage
jina export kubernetes flow.yml ./my-k8s
kubectl apply -R -f my-k8s
jina export docker-compose flow.yml docker-compose.yml
docker-compose up
Deploy with a single command:
jina cloud deploy jcloud-flow.yml
Enable token-by-token streaming for responsive LLM applications:
from docarray import BaseDoc
class PromptDocument(BaseDoc):
prompt: str
max_tokens: int
class ModelOutputDocument(BaseDoc):
token_id: int
generated_text: str
from transformers import GPT2Tokenizer, GPT2LMHeadModel
class TokenStreamingExecutor(Executor):
def __init__(self, **kwargs):
super().__init__(**kwargs)
self.model = GPT2LMHeadModel.from_pretrained('gpt2')
@requests(on='/stream')
async def task(self, doc: PromptDocument, **kwargs) -> ModelOutputDocument:
input = tokenizer(doc.prompt, return_tensors='pt')
input_len = input['input_ids'].shape[1]
for _ in range(doc.max_tokens):
output = self.model.generate(**input, max_new_tokens=1)
if output[0][-1] == tokenizer.eos_token_id:
break
yield ModelOutputDocument(
token_id=output[0][-1],
generated_text=tokenizer.decode(
output[0][input_len:], skip_special_tokens=True
),
)
input = {
'input_ids': output,
'attention_mask': torch.ones(1, len(output[0])),
}
# Server
with Deployment(uses=TokenStreamingExecutor, port=12345, protocol='grpc') as dep:
dep.block()
# Client
async def main():
client = Client(port=12345, protocol='grpc', asyncio=True)
async for doc in client.stream_doc(
on='/stream',
inputs=PromptDocument(prompt='what is the capital of France ?', max_tokens=10),
return_type=ModelOutputDocument,
):
print(doc.generated_text)
Jina-serve is backed by Jina AI and licensed under Apache-2.0.
FAQs
Multimodal AI services & pipelines with cloud-native stack: gRPC, Kubernetes, Docker, OpenTelemetry, Prometheus, Jaeger, etc.
We found that jina demonstrated a healthy version release cadence and project activity because the last version was released less than a year ago. It has 1 open source maintainer collaborating on the project.
Did you know?
Socket for GitHub automatically highlights issues in each pull request and monitors the health of all your open source dependencies. Discover the contents of your packages and block harmful activity before you install or update your dependencies.
Research
Security News
A malicious npm package targets Solana developers, rerouting funds in 2% of transactions to a hardcoded address.
Security News
Research
Socket researchers have discovered malicious npm packages targeting crypto developers, stealing credentials and wallet data using spyware delivered through typosquats of popular cryptographic libraries.
Security News
Socket's package search now displays weekly downloads for npm packages, helping developers quickly assess popularity and make more informed decisions.