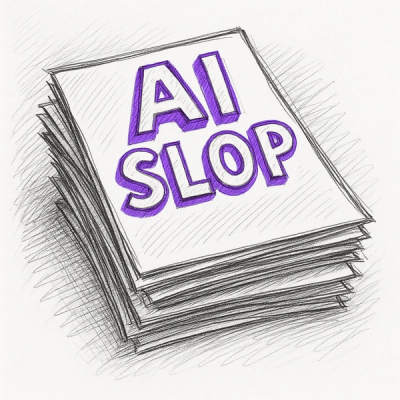
Security News
Django Joins curl in Pushing Back on AI Slop Security Reports
Django has updated its security policies to reject AI-generated vulnerability reports that include fabricated or unverifiable content.
LangAgent is a powerful multi-agent system designed to automate and streamline complex tasks, including research, automated code generation, logical reasoning, data analysis, business intelligence, trading insights, and dynamic reporting.
LangAgent is a versatile multi-agent system designed to automate and streamline a wide range of complex tasks, including research, code generation, logical reasoning, data analysis, dynamic reporting, trading, and business intelligence. Powered by advanced language models, LangAgent integrates seamlessly with APIs, databases, and various data formats, making it an essential tool for developers, data scientists, traders, and business professionals looking to optimize workflows and extract actionable insights.
You can install the langagent
package via pip
:
pip install langagent==3.3.9
To start using the langagent library, you need to import the agents from their respective teams. Below is a detailed guide explaining each agent, its arguments, and how to effectively use them in your projects.
The Research Team consists of three agents: Researcher, Coder, and Weather. Each agent is designed to assist with gathering research, generating code, or fetching weather data.
Researcher Agent
Arguments:
llm
: The language model to be used (e.g., ChatOpenAI
).messages
: A list of HumanMessage
objects representing the research query.Example:
from langagent.research_team.agents import researcher
from langchain_openai import ChatOpenAI
from langchain_core.messages import HumanMessage
import yaml
llm = ChatOpenAI()
os.environ['TAVILY_API_KEY'] = yaml.safe_load(open('credentials.yml'))['online']
search = {
'api_key': os.environ.get("TAVILY_API_KEY"),
'max_results': 5,
'search_depth': "advanced"
}
researcher_agent = researcher.researcher(llm=llm, tavily_search=search)
# Invoke the agent with a query
result = researcher_agent.invoke({"messages": [HumanMessage(content="Who is Messi?")]})
print(result['output'])
Example of Resarcher Workflow
Coder Agent
Arguments:
llm
: The language model you want to use.messages
: A list of HumanMessage
objects representing the code generation task.Example:
from langagent.research_team.agents import coder
from langchain_openai import ChatOpenAI
from langchain_core.messages import HumanMessage
llm = ChatOpenAI()
coder_agent = coder(llm)
# Ask the agent to generate code
result = coder_agent.invoke({"messages": [HumanMessage(content="Write a Python function to calculate the factorial of a number.")]} )
print(result['output'])
Example of Coder Workflow
Weather Agent
Arguments:
llm
: The language model to be used.OPENWEATHERMAP_API_KEY
: Your OpenWeatherMap API key.messages
: A list of HumanMessage
objects representing the weather query.Example:
from langagent.research_team.agents import weather
from langchain_openai import ChatOpenAI
from langchain_core.messages import HumanMessage
llm = ChatOpenAI()
weather_agent = weather(llm, OPENWEATHERMAP_API_KEY="your-api-key-here")
# Ask for weather details
result = weather_agent.invoke({"messages": [HumanMessage(content="What is the weather today in New York?")]})
print(result['output'])
Example of Weather Workflow
The Logic Team consists of two agents: Reasoner and Calculator, both designed to solve logical and mathematical problems efficiently.
Reasoner Agent
Arguments:
llm
: The language model to be used.messages
: A list of HumanMessage
objects describing the logic problem.Example:
from langagent.logic_team.agents import reasoner
from langchain_openai import ChatOpenAI
from langchain_core.messages import HumanMessage
llm = ChatOpenAI()
reasoner_agent = reasoner(llm)
# Solve a logic problem
result = reasoner_agent.invoke({"messages": [HumanMessage(content="I have a 7 in the tens place. I have an even number in the ones place. I am lower than 74. What number am I?")]})
print(result['output'])
Example of Reasoner Workflow
Calculator Agent
Arguments:
llm
: The language model to be used.messages
: A list of HumanMessage
objects containing the mathematical query.Example:
from langagent.logic_team.agents import calculator
from langchain_openai import ChatOpenAI
from langchain_core.messages import HumanMessage
llm = ChatOpenAI()
calculator_agent = calculator(llm)
# Solve a mathematical problem
result = calculator_agent.invoke({"messages": [HumanMessage(content="Calculate the square root of 16")]})
print(result['output'])
Example of Calculator Workflow
The Text Clustering Team consists of one agent: Topic Generator, designed to provide insights from data, either through text clustering.
Topic Generator Agent
Arguments:
llm
: The language model to be used.embedding_model
: The embedding model used for text vectorization. If None
, it defaults to SentenceTransformer('all-MiniLM-L6-v2')
.path
: The path to the CSV or Excel file containing text data.text_column
: The column in the file that contains the text data.user_topics
: (Optional) User-provided topics for clustering. If None
, topics will be automatically generated.summary_system_prompt
: (Optional) Custom system prompt for summarization. Defaults to:"You are an expert summarizer. Your task is to generate precise and concise summaries."
summary_user_prompt
: (Optional) Custom user prompt for summarization. Defaults to:"Generate a ten-word summary of the text below:\nText: {transcript}"
topic_system_prompt_with_user_topics
: (Optional) Custom system prompt for topic generation when user topics are provided. Defaults to:"You are an expert in {user_request}, tasked with analyzing and categorizing diverse user-generated content.
Your goal is to accurately assign each summary to one of the following topics: {topics_str}.
Ensure that your categorizations are precise and based on a deep understanding of the context."
topic_user_prompt_with_user_topics
: (Optional) Custom user prompt for topic generation when user topics are provided. Defaults to:"Based on the summaries provided, match each summary to the most relevant topic from the list below.
Summaries: {{{column_name}}}.\n\nPlease return your response in the format 'Summary: Topic'."
topic_system_prompt_no_user_topics
: (Optional) Custom system prompt for topic generation when user topics are not provided. Defaults to:"You are an expert in {user_request}, specialized in analyzing user-generated content such as reviews,
comments, feedback, and discussions. Your task is to generate a concise and informative topic title
that accurately summarizes a set of summaries in the given context."
topic_user_prompt_no_user_topics
: (Optional) Custom user prompt for topic generation when user topics are not provided. Defaults to:"Review the following summaries and generate a clear, concise topic title that encapsulates the main themes:\n\n
Summaries: {{{column_name}}}.\n\nTOPIC TITLE:"
Example:
from LangAgent.text_clustering_team.agents import topic_generator
from sentence_transformers import SentenceTransformer
from langchain_openai import ChatOpenAI
# Initialize the language model
llm = ChatOpenAI()
# Initialize the embedding model
embedding_model = None
# Create the topic generator agent with default prompts
topic_generator_agent = topic_generator(
llm,
embedding_model,
summary_system_prompt=None,
summary_user_prompt=None,
topic_system_prompt_with_user_topics=None,
topic_user_prompt_with_user_topics=None,
topic_system_prompt_no_user_topics=None,
topic_user_prompt_no_user_topics=None,
)
inputs = {
'path': '../Comments.csv',
'text_column': 'Comments',
'user_topics': None
}
result = topic_generator_agent.invoke(inputs)
result['summary_df'] # View the summarized data
result['fig'].show() # Show the visualization
Example of Topic Generator Workflow
The Business Intelligence Team consists of one agent: BI Analyst Agent, designed to provide insights from data through SQL-based analysis.
*BI Analyst Agent
Arguments:
db_path
: The path to your SQLite or SQL database.llm
: The language model.user_question
: The question that drives the SQL query and chart generation.Example:
from langagent.business_intelligence_team.agents import bi_analyst
from langchain_openai import ChatOpenAI
from langchain_experimental.utilities import PythonREPL
llm = ChatOpenAI()
PATH_DB = "sqlite:///database/leads_scored.db"
bi_analyst_agent = bi_analyst(db_path=PATH_DB, llm=llm)
question = """
What are the total sales by month-year?
Use suggested price as a proxy for revenue for each transaction and a quantity of 1.
Make a line chart of sales over time.
"""
initial_input = {
"user_question": question
}
# Invoke the agent with the input
result = bi_analyst_agent.invoke(initial_input)
print(result['sql_query']) # SQL Query
print(result['summary']) # Summary of results
# Execute the Python code for chart generation
repl = PythonREPL()
repl.run(result['chart_plotly_code'])
Example of BI Analyst Workflow
The Reporting Team consists of two agents: Interpreter and Summarizer, both designed to provide insights and summaries of outputs from various sources such as data, charts, or documents.
Interpreter Agent
Arguments:
llm
: The language model.code_output
: The result or output that needs to be interpreted.Example:
from langagent.reporting_team.agents import interpreter
from langchain_openai import ChatOpenAI
llm = ChatOpenAI()
interpreter_agent = interpreter(llm)
result = interpreter_agent.invoke({"code_output": "Bar chart showing sales data."})
print(result['output'])
Example of Interpreter Workflow
Summarizer Agent
Arguments:
llm
: The language model.input_data
: The path to the document or text file that needs to be summarized.Example:
from langagent.reporting_team.agents import summarizer
from langchain_openai import ChatOpenAI
llm = ChatOpenAI()
summarizer_agent = summarizer(llm)
inputs = {
'input_data': '../Comments.csv'
}
result = summarizer_agent.invoke(inputs)
print(result['summary'])
Example of Summarizer Workflow
The Data Science Team consists of specialized agents that can operate individually for specific tasks or collaborate together to handle complex data science workflows.
The Data Cleaning Agent applies a series of data preprocessing steps to clean raw datasets based on user instructions.
Arguments:
llm
: The language model to generate data cleaning instructions and code.log
: Whether to log the generated code and errors (default: False
).log_path
: Path for log storage (default: None
).overwrite
: Overwrite existing logs if True
(default: True
).human_in_the_loop
: Enables user review of cleaning instructions if True
(default: False
).Example:
from langagent.data_science_team.agents import data_cleaning_agent
from langchain_openai import ChatOpenAI
import pandas as pd
llm = ChatOpenAI()
cleaning_agent = data_cleaning_agent(llm)
inputs = {
'user_instructions': "Don't remove outliers when cleaning the data.",
'data_raw': pd.read_csv("../data/churn_data.csv").to_dict(),
'max_retries': 3,
'retry_count': 0
}
result = cleaning_agent.invoke(inputs)
pd.DataFrame(result['data_cleaned']) # View the cleaned data
Example of Data Cleaning Agent without Human Review Workflow
Example of Data Cleaning Agent with Human Review Workflow
The Data Wrangling Agent performs complex data transformation tasks such as merging datasets, reshaping data, and encoding features.
Arguments:
llm
: The language model to generate wrangling instructions and code.log
: Whether to log the generated code and errors (default: False
).log_path
: Path for log storage (default: None
).overwrite
: Overwrite existing logs if True
(default: True
).human_in_the_loop
: Enables user review of wrangling instructions if True
(default: False
).Example:
from langagent.data_science_team.agents import data_wrangling_agent
from langchain_openai import ChatOpenAI
import pandas as pd
llm = ChatOpenAI()
wrangling_agent = data_wrangling_agent(llm)
inputs = {
'user_instructions': "Aggregate 'sales' by 'region' and compute the average.",
'data_raw': pd.read_csv("../data/sales_data.csv").to_dict(),
'max_retries': 3,
'retry_count': 0
}
result = wrangling_agent.invoke(inputs)
pd.DataFrame(result['data_wrangled']) # View the transformed data
Example of Data Wrangling Agent without Human Review Workflow
Example of Data Wrangling Agent with Human Review Workflow
The Feature Engineering Agent automates feature extraction and transformation, such as encoding, scaling, and feature generation.
Arguments:
llm
: The language model to generate feature engineering instructions and code.log
: Whether to log the generated code and errors (default: False
).log_path
: Path for log storage (default: None
).overwrite
: Overwrite existing logs if True
(default: True
).human_in_the_loop
: Enables user review of feature engineering instructions if True
(default: False
).Example:
from langagent.data_science_team.agents import feature_engineering_agent
from langchain_openai import ChatOpenAI
import pandas as pd
llm = ChatOpenAI()
feature_engineering_agent = feature_engineering_agent(llm)
inputs = {
'user_instructions': "Create interaction terms for 'age' and 'income'.",
'data_raw': pd.read_csv("../data/customer_data.csv").to_dict(),
'target_variable': "Churn",
'max_retries': 3,
'retry_count': 0
}
result = feature_engineering_agent.invoke(inputs)
pd.DataFrame(result['data_engineered']) # View the engineered features
Example of Feature Engineering Agent without Human Review Workflow
Example of Feature Engineering Agent with Human Review Workflow
The SQL Database Agent generates SQL queries and executes them on a connected database based on user-provided instructions.
Arguments:
llm
: The language model to generate SQL instructions and code.connection
: SQLAlchemy engine or connection object for accessing the database.n_samples
: Number of sample rows to retrieve during metadata analysis (default: 10
).log
: Whether to log the generated code and errors (default: False
).log_path
: Path for log storage (default: None
).overwrite
: Overwrite existing logs if True
(default: True
).human_in_the_loop
: Enables user review of SQL instructions if True
(default: False
).Example:
from langagent.data_science_team.agents import sql_database_agent
from langchain_openai import ChatOpenAI
import sqlalchemy as sql
llm = ChatOpenAI()
sql_engine = sql.create_engine("sqlite:///data/database-sql-transactions/leads_scored.db")
conn = sql_engine.connect()
sql_agent = sql_database_agent(llm, connection=conn)
inputs = {
'user_instructions': "List the tables in the database.",
'max_retries': 3,
'retry_count': 0
}
result = sql_agent.invoke(inputs)
result['data_sql'] # View the query results
Example of SQL Database Agent without Human Review Workflow
Example of SQL Database Agent with Human Review Workflow
The Trading Team consists of specialized agents that can operate individually for specific trading tasks or collaborate together to handle complex trading workflows.
The Fundamentals Analyst Agent evaluates a company's financial metrics, compares them with industry benchmarks, and generates trading signals.
model
: The language model used for reasoning and explanations.industry_benchmarks
: (Optional) A dictionary of industry-specific benchmarks for financial metrics.api_key
: (Optional) API key for accessing financial datasets.from langagent.trading_team.agents import make_fundamentals_agent
from langchain_openai import ChatOpenAI
llm = ChatOpenAI()
fundamentals_agent = make_fundamentals_agent(llm)
# Set up the financial data API key
os.environ["api_key"] = "api_key"
inputs = {
"user_instructions": "Analyze my portfolio of $10,000 in cash, with $3,000 in Apple (AAPL) and $4,000 in Microsoft (MSFT), focusing on long-term investment goals. Using the last two statements, evaluate the portfolio's performance and conduct a TTM analysis of AAPL and MSFT from October 1, 2024, to December 31, 2024, to inform long-term strategies",
'financial_data_api_key': os.environ.get("api_key"),
}
result = fundamentals_agent.invoke(inputs)
print(result['report']) # View the fundamentals analysis report
Example of Fundamentals Analyst Agent Workflow
The Sentiments Analyst Agent analyzes insider trades and company news to determine the market sentiment for a stock, generating bullish or bearish signals.
model
: The language model used for reasoning and explanations.api_key
: (Optional) API key for accessing financial datasets.from langagent.trading_team.agents import make_sentiments_agent
from langchain_openai import ChatOpenAI
llm = ChatOpenAI()
sentiments_agent = make_sentiments_agent(llm)
# Set up the financial data API key
os.environ["api_key"] = "api_key"
inputs = {
"user_instructions": "Analyze my portfolio of $10,000 in cash, with $3,000 in Apple (AAPL) and $4,000 in Microsoft (MSFT), focusing on long-term investment goals. Using the last two statements, evaluate the portfolio's performance and conduct a TTM analysis of AAPL and MSFT from October 1, 2024, to December 31, 2024, to inform long-term strategies",
'financial_data_api_key': os.environ.get("api_key"),
}
result = sentiments_agent.invoke(inputs)
print(result['report']) # View the sentiment analysis report
Example of Sentiments Analyst Agent Workflow
The Technicals Analyst Agent applies advanced technical indicators, such as trend analysis, momentum, mean reversion, and volatility measures, to generate trading signals.
model
: The language model used for reasoning and explanations.api_key
: (Optional) API key for accessing historical price data.market_index_df
: (Optional) DataFrame containing market index data for relative strength analysis.related_securities_dfs
: (Optional) Dictionary of related securities for correlation analysis.strategy_weights
: (Optional) Dictionary specifying weight distribution among different technical strategies.from langagent.trading_team.agents import make_technicals_agent
from langchain_openai import ChatOpenAI
llm = ChatOpenAI()
technicals_agent = make_technicals_agent(llm)
# Set up the financial data API key
os.environ["api_key"] = "api_key"
inputs = {
"user_instructions": "Analyze my portfolio of $10,000 in cash, with $3,000 in Apple (AAPL) and $4,000 in Microsoft (MSFT), focusing on long-term investment goals. Using the last two statements, evaluate the portfolio's performance and conduct a TTM analysis of AAPL and MSFT from October 1, 2024, to December 31, 2024, to inform long-term strategies",
'financial_data_api_key': os.environ.get("api_key"),
}
result = technicals_agent.invoke(inputs)
print(result['report']) # View the technical analysis report
Example of Technicals Analyst Agent Workflow
The Valuations Analyst Agent performs discounted cash flow (DCF) and Owner Earnings valuation to assess whether a stock is undervalued or overvalued based on intrinsic value calculations.
model
: The language model used for reasoning and explanations.api_key
: (Optional) API key for accessing financial datasets.dcf_assumptions
: (Optional) Dictionary of assumptions for discounted cash flow valuation.owner_earnings_assumptions
: (Optional) Dictionary of assumptions for Owner Earnings valuation.sensitivity_ranges
: (Optional) Dictionary specifying ranges for sensitivity analysis.from langagent.trading_team.agents import make_valuations_agent
from langchain_openai import ChatOpenAI
llm = ChatOpenAI()
valuations_agent = make_valuations_agent(llm)
# Set up the financial data API key
os.environ["api_key"] = "api_key"
inputs = {
"user_instructions": "Analyze my portfolio of $10,000 in cash, with $3,000 in Apple (AAPL) and $4,000 in Microsoft (MSFT), focusing on long-term investment goals. Using the last two statements, evaluate the portfolio's performance and conduct a TTM analysis of AAPL and MSFT from October 1, 2024, to December 31, 2024, to inform long-term strategies",
'financial_data_api_key': os.environ.get("api_key"),
}
result = valuations_agent.invoke(inputs)
print(result['report']) # View the valuation analysis report
Example of Valuations Analyst Agent Workflow
The Risk Manager Agent evaluates portfolio risk, determines position limits, and recommends position sizes based on real-world risk factors.
model
: The language model used for risk management calculations.api_key
: (Optional) API key for accessing financial datasets.from langagent.trading_team.agents import make_risk_manager_agent
from langchain_openai import ChatOpenAI
llm = ChatOpenAI()
risk_manager_agent = make_risk_manager_agent(llm)
# Set up the financial data API key
os.environ["api_key"] = "api_key"
inputs = {
"user_instructions": "Analyze my portfolio of $10,000 in cash, with $3,000 in Apple (AAPL) and $4,000 in Microsoft (MSFT), focusing on long-term investment goals. Using the last two statements, evaluate the portfolio's performance and conduct a TTM analysis of AAPL and MSFT from October 1, 2024, to December 31, 2024, to inform long-term strategies",
'financial_data_api_key': os.environ.get("api_key"),
}
result = risk_manager_agent.invoke(inputs)
print(result['report']) # View the risk management analysis
Example of Risk Manager Agent Workflow
The Portfolio Manager Agent integrates insights from multiple analysts (Fundamentals, Sentiments, Valuations, Technicals, Risk Management) to generate comprehensive trading decisions.
model
: The language model used for reasoning and trading strategy evaluations.api_key
: (Optional) API key for accessing financial datasets.industry_benchmarks
: (Optional) Industry benchmark data for fundamentals analysis.strategy_weights
: (Optional) Weightings for different investment strategies.from langagent.trading_team.agents import make_portfolio_manager_agent
from langchain_openai import ChatOpenAI
llm = ChatOpenAI()
portfolio_manager_agent = make_portfolio_manager_agent(llm)
# Set up the financial data API key
os.environ["api_key"] = "api_key"
inputs = {
"user_instructions": "Analyze my portfolio of $10,000 in cash, with nothing invested in NVDA and in TSLA, focusing on long-term investment goals. Using the last two statements, evaluate the portfolio's performance and conduct a TTM analysis of NVDA and TSLA from November 1, 2024, to January 20, 2025, to inform balanced strategies",
'financial_data_api_key': os.environ["api_key"],
}
result = portfolio_manager_agent.invoke(inputs)
print(result['trading_table']) # View the final trading decisions
Example of *Portfolio Manager Agent Workflow
The Supervisor Chain manages the workflow between multiple agents. It selects the next agent based on predefined criteria or completion conditions. This is useful when you want to automate multi-step tasks and route tasks to different agents based on the flow of the conversation or task requirements.
Arguments:
subagent_names
: A list of subagents (workers) that will perform tasks.llm
: The language model that powers decision-making.subagent_descriptions
: A dictionary of subagent names with descriptions of their roles (optional).completion_criteria
: A string representing the criteria that signals completion of the workflow.history_depth
: The number of previous messages to consider when making routing decisions (optional).Example:
from langagent.supervisor import supervisor_chain
from langchain_openai import ChatOpenAI
llm = ChatOpenAI()
# Define the subagents and their descriptions
subagent_names = ["researcher", "coder", "calculator"]
subagent_descriptions = {
"researcher": "Finds relevant research and gathers data.",
"coder": "Generates, debugs, and optimizes code.",
"calculator": "Solves mathematical problems and queries."
}
# Create a supervisor chain to manage the workflow
supervisor = supervisor_chain(
subagent_names=subagent_names,
llm=llm,
subagent_descriptions=subagent_descriptions,
completion_criteria="FINISH"
)
Example of Supervision Workflow
To use the Team Agents, ensure that you have the following API keys configured:
For OpenAI models (GPT-4o, GPT-4o-mini, etc.):
OPENAI_API_KEY=your-openai-api-key
For Groq-hosted models (DeepSeek, LLaMA3, etc.):
GROQ_API_KEY=your-groq-api-key
For financial datasets powering the hedge fund:
FINANCIAL_DATASETS_API_KEY=your-financial-datasets-api-key
These API keys should be set in your environment variables for seamless agent execution.
LangAgent relies on several core libraries, including:
Install the required dependencies using:
pip install -r requirements.txt
We welcome contributions to LangAgent! If you'd like to contribute:
git checkout -b feature/new-feature
).git commit -m "Add new feature"
).git push origin feature/new-feature
).This project is licensed under the MIT License. See the LICENSE file for details.
For any inquiries or issues, please contact:
FAQs
LangAgent is a powerful multi-agent system designed to automate and streamline complex tasks, including research, automated code generation, logical reasoning, data analysis, business intelligence, trading insights, and dynamic reporting.
We found that langagent demonstrated a healthy version release cadence and project activity because the last version was released less than a year ago. It has 1 open source maintainer collaborating on the project.
Did you know?
Socket for GitHub automatically highlights issues in each pull request and monitors the health of all your open source dependencies. Discover the contents of your packages and block harmful activity before you install or update your dependencies.
Security News
Django has updated its security policies to reject AI-generated vulnerability reports that include fabricated or unverifiable content.
Security News
ECMAScript 2025 introduces Iterator Helpers, Set methods, JSON modules, and more in its latest spec update approved by Ecma in June 2025.
Security News
A new Node.js homepage button linking to paid support for EOL versions has sparked a heated discussion among contributors and the wider community.