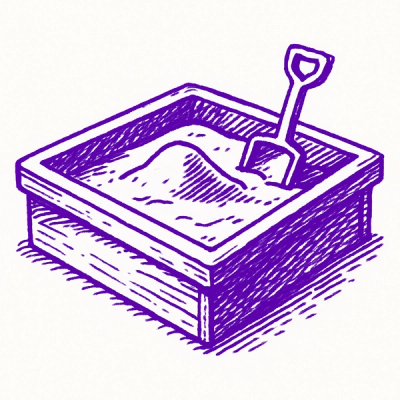
Research
/Security News
Critical Vulnerability in NestJS Devtools: Localhost RCE via Sandbox Escape
A flawed sandbox in @nestjs/devtools-integration lets attackers run code on your machine via CSRF, leading to full Remote Code Execution (RCE).
llama-index-readers-wordlift
Advanced tools
pip install llama-index-readers-wordlift
The WordLift GraphQL Reader is a connector to fetch and transform data from a WordLift Knowledge Graph using your the WordLift Key. The connector provides a convenient way to load data from WordLift using a GraphQL query and transform it into a list of documents for further processing.
To use the WordLift GraphQL Reader, follow the steps below:
WordLiftLoader
class, passing in the configuration options.load_data
method to fetch and transform the data.Here's an example of how to use the WordLift GraphQL Reader:
import json
from llama_index.core import VectorStoreIndex
from llama_index.core import Document
from langchain.llms import OpenAI
from llama_index.readers.wordlift import WordLiftLoader
# Set up the necessary configuration options
endpoint = "https://api.wordlift.io/graphql"
headers = {
"Authorization": "<YOUR_WORDLIFT_KEY>",
"Content-Type": "application/json",
}
query = """
# Your GraphQL query here
"""
fields = "<YOUR_FIELDS>"
config_options = {
"text_fields": ["<YOUR_TEXT_FIELDS>"],
"metadata_fields": ["<YOUR_METADATA_FIELDS>"],
}
# Create an instance of the WordLiftLoader
reader = WordLiftLoader(endpoint, headers, query, fields, config_options)
# Load the data
documents = reader.load_data()
# Convert the documents
converted_doc = []
for doc in documents:
converted_doc_id = json.dumps(doc.doc_id)
converted_doc.append(
Document(
text=doc.text,
doc_id=converted_doc_id,
embedding=doc.embedding,
doc_hash=doc.doc_hash,
extra_info=doc.extra_info,
)
)
# Create the index and query engine
index = VectorStoreIndex.from_documents(converted_doc)
query_engine = index.as_query_engine()
# Perform a query
result = query_engine.query("<YOUR_QUERY>")
# Process the result as needed
logging.info("Result: %s", result)
FAQs
llama-index readers wordlift integration
We found that llama-index-readers-wordlift demonstrated a healthy version release cadence and project activity because the last version was released less than a year ago. It has 1 open source maintainer collaborating on the project.
Did you know?
Socket for GitHub automatically highlights issues in each pull request and monitors the health of all your open source dependencies. Discover the contents of your packages and block harmful activity before you install or update your dependencies.
Research
/Security News
A flawed sandbox in @nestjs/devtools-integration lets attackers run code on your machine via CSRF, leading to full Remote Code Execution (RCE).
Product
Customize license detection with Socket’s new license overlays: gain control, reduce noise, and handle edge cases with precision.
Product
Socket now supports Rust and Cargo, offering package search for all users and experimental SBOM generation for enterprise projects.