Jiancheng Yang, Rui Shi, Donglai Wei, Zequan Liu, Lin Zhao, Bilian Ke, Hanspeter Pfister, Bingbing Ni
18x Standardized Datasets for 2D and 3D Biomedical Image Classification
Multiple Size Options: 28 (MNIST-Like), 64, 128, and 224

We introduce MedMNIST, a large-scale MNIST-like collection of standardized biomedical images, including 12 datasets for 2D and 6 datasets for 3D. All images are pre-processed into 28x28 (2D) or 28x28x28 (3D) with the corresponding classification labels, so that no background knowledge is required for users. Covering primary data modalities in biomedical images, MedMNIST is designed to perform classification on lightweight 2D and 3D images with various data scales (from 100 to 100,000) and diverse tasks (binary/multi-class, ordinal regression and multi-label). The resulting dataset, consisting of approximately 708K 2D images and 10K 3D images in total, could support numerous research and educational purposes in biomedical image analysis, computer vision and machine learning. We benchmark several baseline methods on MedMNIST, including 2D / 3D neural networks and open-source / commercial AutoML tools.
Update: We are thrilled to release MedMNIST+ with larger sizes: 64x64, 128x128, and 224x224 for 2D, and 64x64x64 for 3D. As a complement to the previous 28-size MedMNIST, the large-size version could serve as a standardized benchmark for medical foundation models. Install the latest API to try it out!
Third-Party Update: @sdoerrich97 released a comprehensive evaluation for MedMNIST+ covering 10 different deep learning models trained via 3 distinct training schemes across all 12 2D datasets and available image resolutions (28x28, 64x64, 128x128, and 224x224), which may be interesting for the MedMNIST community. Check the issue here.
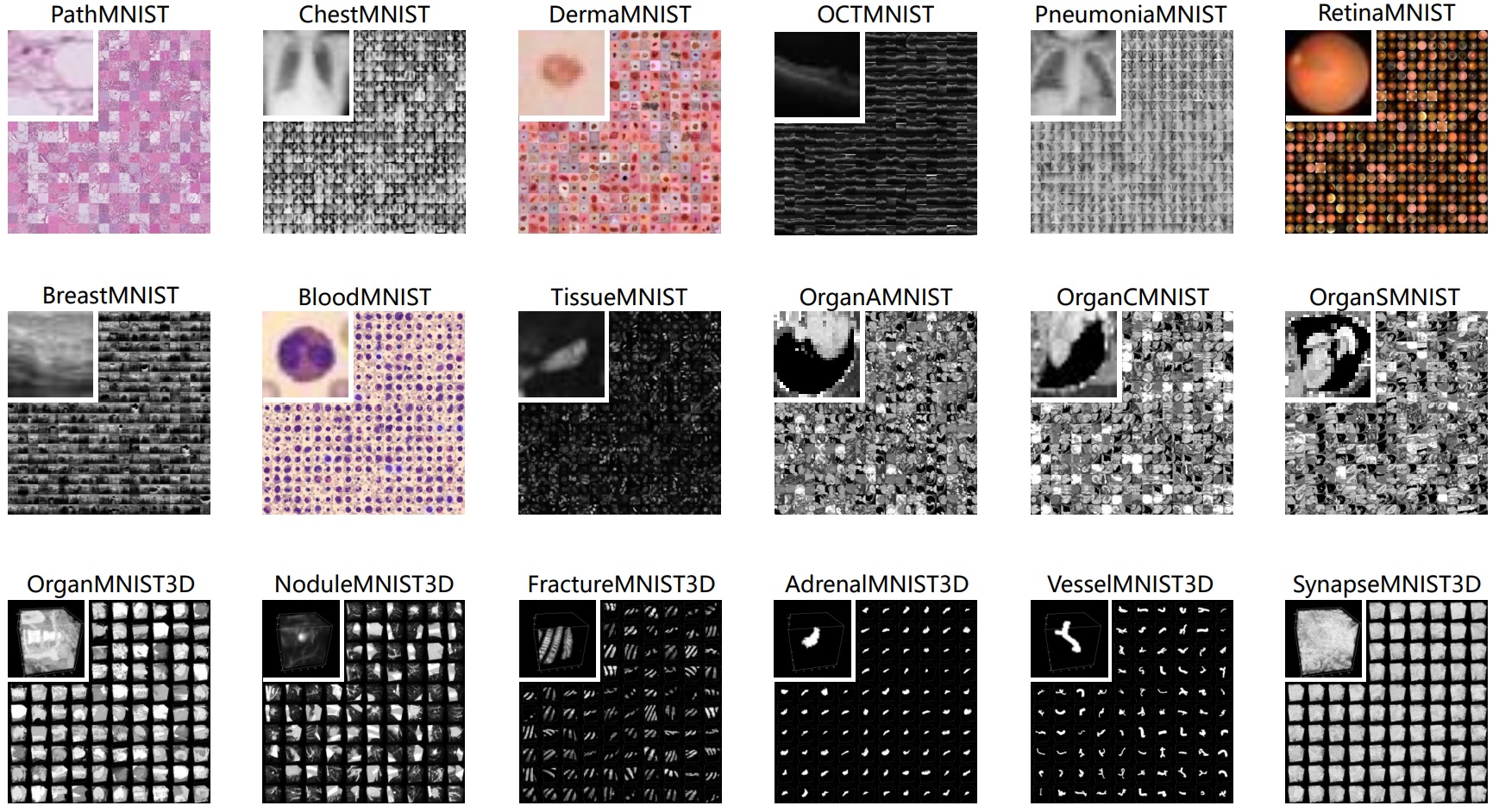
For more details, please refer to our paper:
MedMNIST v2: A Large-Scale Lightweight Benchmark for 2D and 3D Biomedical Image Classification (Nature Scientific Data'23)
or its conference version:
MedMNIST Classification Decathlon: A Lightweight AutoML Benchmark for Medical Image Analysis (ISBI'21)
Key Features
- Diverse: It covers diverse data modalities, dataset scales (from 100 to 100,000), and tasks (binary/multi-class, multi-label, and ordinal regression). It is as diverse as the VDD and MSD to fairly evaluate the generalizable performance of machine learning algorithms in different settings, but both 2D and 3D biomedical images are provided.
- Standardized: Each sub-dataset is pre-processed into the same format, which requires no background knowledge for users. As an MNIST-like dataset collection to perform classification tasks on small images, it primarily focuses on the machine learning part rather than the end-to-end system. Furthermore, we provide standard train-validation-test splits for all datasets in MedMNIST, therefore algorithms could be easily compared.
- User-Friendly: The small size of 28x28 (2D) or 28x28x28 (3D) is lightweight and ideal for evaluating machine learning algorithms. We also offer a larger-size version, MedMNIST+: 64x64 (2D), 128x128 (2D), 224x224 (2D), and 64x64x64 (3D). Serving as a complement to the 28-size MedMNIST, this could be a standardized resource for developing medical foundation models. All these datasets are accessible via the same API.
- Educational: As an interdisciplinary research area, biomedical image analysis is difficult to hand on for researchers from other communities, as it requires background knowledge from computer vision, machine learning, biomedical imaging, and clinical science. Our data with the Creative Commons (CC) License is easy to use for educational purposes.
Please note that this dataset is NOT intended for clinical use.
Code Structure
medmnist/
:
dataset.py
: PyTorch datasets and dataloaders of MedMNIST.evaluator.py
: Standardized evaluation functions.info.py
: Dataset information dict
for each subset of MedMNIST.
examples/
:
getting_started.ipynb
: To explore the MedMNIST dataset with jupyter notebook. It is ONLY intended for a quick exploration, i.e., it does not provide full training and evaluation functionalities.getting_started_without_PyTorch.ipynb
: This notebook provides snippets about how to use MedMNIST data (the .npz
files) without PyTorch.
setup.py
: To install medmnist
as a module.- [EXTERNAL]
MedMNIST/experiments
: training and evaluation scripts to reproduce both 2D and 3D experiments in our paper, including PyTorch, auto-sklearn, AutoKeras and Google AutoML Vision together with their weights ;)
Installation and Requirements
Setup the required environments and install medmnist
as a standard Python package from PyPI:
pip install medmnist
Or install from source:
pip install --upgrade git+https://github.com/MedMNIST/MedMNIST.git
Check whether you have installed the latest code version:
>>> import medmnist
>>> print(medmnist.__version__)
The code requires only common Python environments for machine learning. Basically, it was tested with
- Python 3 (>=3.6)
- PyTorch==1.3.1
- numpy==1.18.5, pandas==0.25.3, scikit-learn==0.22.2, Pillow==8.0.1
- fire, scikit-image
Higher (or lower) versions should also work (perhaps with minor modifications).
Quick Start
To use the standard 28-size (MNIST-like) version utilizing the downloaded files:
>>> from medmnist import PathMNIST
>>> train_dataset = PathMNIST(split="train")
To enable automatic downloading by setting download=True
:
>>> from medmnist import NoduleMNIST3D
>>> val_dataset = NoduleMNIST3D(split="val", download=True)
Alternatively, you can access MedMNIST+ with larger image sizes by specifying the size
parameter:
>>> from medmnist import ChestMNIST
>>> test_dataset = ChestMNIST(split="test", download=True, size=224)
If you use PyTorch...
-
Great! Our code is designed to work with PyTorch.
-
Explore the MedMNIST dataset with jupyter notebook (getting_started.ipynb
), and train basic neural networks in PyTorch.
If you do not use PyTorch...
- Although our code is tested with PyTorch, you are free to parse them with your own code (without PyTorch or even without Python!), as they are only standard NumPy serialization files. It is simple to create a dataset without PyTorch.
- Go to
getting_started_without_PyTorch.ipynb
, which provides snippets about how to use MedMNIST data (the .npz
files) without PyTorch. - Simply change the super class of
MedMNIST
from torch.utils.data.Dataset
to collections.Sequence
, you will get a standard dataset without PyTorch. Check dataset_without_pytorch.py
for more details. - You still have most functionality of our MedMNIST code ;)
Dataset
Please download the dataset(s) via Zenodo. You could also use our code to download automatically by setting download=True
in dataset.py
.
The MedMNIST dataset contains several subsets. Each subset (e.g., pathmnist.npz
) is comprised of 6 keys: train_images
, train_labels
, val_images
, val_labels
, test_images
and test_labels
.
train_images
/ val_images
/ test_images
: N
× 28 × 28 for 2D gray-scale datasets, N
× 28 × 28 × 3 for 2D RGB datasets, N
× 28 × 28 × 28 for 3D datasets. N
denotes the number of samples.train_labels
/ val_labels
/ test_labels
: N
× L
. N
denotes the number of samples. L
denotes the number of task labels; for single-label (binary/multi-class) classification, L=1
, and {0,1,2,3,..,C}
denotes the category labels (C=1
for binary); for multi-label classification L!=1
, e.g., L=14
for chestmnist.npz
.
Additionally, we provide a CSV file for each MedMNIST subset here, which maps the "image_id" in the subset to the corresponding image in the source dataset. For each entry, it details the specific "split" and "index" within the MedMNIST subset, along with the corresponding image name from the official source dataset.
Command Line Tools
-
List all available datasets:
python -m medmnist available
-
Download available datasets of a specific size (size=None
(28) by default):
python -m medmnist download --size=28
To download all available sizes:
python -m medmnist download --size=all
-
Delete all downloaded npz from root:
python -m medmnist clean
-
Print the dataset details given a subset flag:
python -m medmnist info --flag=xxxmnist
-
Save the dataset as standard figure and csv files, which could be used for AutoML tools, e.g., Google AutoML Vision:
for 2D datasets:
python -m medmnist save --flag=xxxmnist --folder=tmp/ --postfix=png --download=True --size=28
for 3D datasets:
python -m medmnist save --flag=xxxmnist3d --folder=tmp/ --postfix=gif --download=True --size=28
By default, download=False
and size=None
(28).
-
Parse and evaluate a standard result file, refer to Evaluator.parse_and_evaluate
for details.
python -m medmnist evaluate --path=folder/{flag}{size_flag}_{split}@{run}.csv
Here, size_flag
is blank for 28 images, and _size
for larger images, e.g., "_64", e.g.,
python -m medmnist evaluate --path=bloodmnist_64_val_[AUC]0.486_[ACC]0.114@dummy.csv
or
python -m medmnist evaluate --path=chestmnist_test_[AUC]0.500_[ACC]0.499@dummy.csv
License and Citation
The code is under Apache-2.0 License.
The MedMNIST dataset is licensed under Creative Commons Attribution 4.0 International (CC BY 4.0), except DermaMNIST under
Creative Commons Attribution-NonCommercial 4.0 International (CC BY-NC 4.0).
If you find this project useful in your research, please cite the following papers:
Jiancheng Yang, Rui Shi, Donglai Wei, Zequan Liu, Lin Zhao, Bilian Ke, Hanspeter Pfister, Bingbing Ni. Yang, Jiancheng, et al. "MedMNIST v2-A large-scale lightweight benchmark for 2D and 3D biomedical image classification." Scientific Data, 2023.
Jiancheng Yang, Rui Shi, Bingbing Ni. "MedMNIST Classification Decathlon: A Lightweight AutoML Benchmark for Medical Image Analysis". IEEE 18th International Symposium on Biomedical Imaging (ISBI), 2021.
or using the bibtex:
@article{medmnistv2,
title={MedMNIST v2-A large-scale lightweight benchmark for 2D and 3D biomedical image classification},
author={Yang, Jiancheng and Shi, Rui and Wei, Donglai and Liu, Zequan and Zhao, Lin and Ke, Bilian and Pfister, Hanspeter and Ni, Bingbing},
journal={Scientific Data},
volume={10},
number={1},
pages={41},
year={2023},
publisher={Nature Publishing Group UK London}
}
@inproceedings{medmnistv1,
title={MedMNIST Classification Decathlon: A Lightweight AutoML Benchmark for Medical Image Analysis},
author={Yang, Jiancheng and Shi, Rui and Ni, Bingbing},
booktitle={IEEE 18th International Symposium on Biomedical Imaging (ISBI)},
pages={191--195},
year={2021}
}
Please also cite source data paper(s) of the MedMNIST subset(s) as per the description on the project page.
Release Notes
v3.0.2
: Bug fixed in Evaluator (size_flag
) and PyPI updated.v3.0.1
: Updated the downloading error message to make it more instructive.v3.0.0
: MedMNIST+ featuring larger sizes: 64x64, 128x128, and 224x224 for 2D, and 64x64x64 for 3D.v2.2.4
: Removed a small number of blank samples in OrganAMNIST, OrganCMNIST, OrganSMNIST, OrganMNIST3D, and VesselMNIST3D.v2.2.3
: DermaMNIST license to CC BY-NC 4.0v2.2.2
: Python 3.11 Sequence
from collections.abc supportedv2.2.1
: PyPI info updatedv2.2.0
: montage
method supported for scikit-image>=0.20.0v2.1.0
: NoduleMNIST3D
data error fixedv2.0.0
: MedMNIST v2 release (on PyPI)v1.0.0
: MedMNIST v1 releasev0.2.0
: MedMNIST beta release