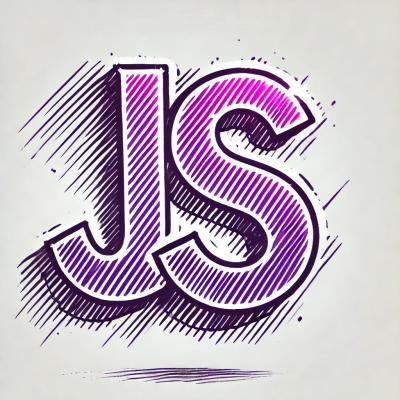
Security News
Oracle Drags Its Feet in the JavaScript Trademark Dispute
Oracle seeks to dismiss fraud claims in the JavaScript trademark dispute, delaying the case and avoiding questions about its right to the name.
MLflow is an open-source platform, purpose-built to assist machine learning practitioners and teams in handling the complexities of the machine learning process. MLflow focuses on the full lifecycle for machine learning projects, ensuring that each phase is manageable, traceable, and reproducible
The core components of MLflow are:
To install the MLflow Python package, run the following command:
pip install mlflow
Alternatively, you can install MLflow from on differnet package hosting platforms:
PyPI | |
conda-forge | |
CRAN | |
Maven Central |
Official documentation for MLflow can be found at here.
You can run MLflow on many different environments, including local development, Amazon SageMaker, AzureML, and Databricks. Please refer to this guidance for how to setup MLflow on your environment.
The following examples trains a simple regression model with scikit-learn, while enabling MLflow's autologging feature for experiment tracking.
import mlflow
from sklearn.model_selection import train_test_split
from sklearn.datasets import load_diabetes
from sklearn.ensemble import RandomForestRegressor
# Enable MLflow's automatic experiment tracking for scikit-learn
mlflow.sklearn.autolog()
# Load the training dataset
db = load_diabetes()
X_train, X_test, y_train, y_test = train_test_split(db.data, db.target)
rf = RandomForestRegressor(n_estimators=100, max_depth=6, max_features=3)
# MLflow triggers logging automatically upon model fitting
rf.fit(X_train, y_train)
Once the above code finishes, run the following command in a separate terminal and access the MLflow UI via the printed URL. An MLflow Run should be automatically created, which tracks the training dataset, hyper parameters, performance metrics, the trained model, dependencies, and even more.
mlflow ui
You can deploy the logged model to a local inference server by a one-line command using the MLflow CLI. Visit the documentation for how to deploy models to other hosting platforms.
mlflow models serve --model-uri runs:/<run-id>/model
The following example runs automatic evaluation for question-answering tasks with several built-in metrics.
import mlflow
import pandas as pd
# Evaluation set contains (1) input question (2) model outputs (3) ground truth
df = pd.DataFrame(
{
"inputs": ["What is MLflow?", "What is Spark?"],
"outputs": [
"MLflow is an innovative fully self-driving airship powered by AI.",
"Sparks is an American pop and rock duo formed in Los Angeles.",
],
"ground_truth": [
"MLflow is an open-source platform for managing the end-to-end machine learning (ML) "
"lifecycle.",
"Apache Spark is an open-source, distributed computing system designed for big data "
"processing and analytics.",
],
}
)
eval_dataset = mlflow.data.from_pandas(
df, predictions="outputs", targets="ground_truth"
)
# Start an MLflow Run to record the evaluation results to
with mlflow.start_run(run_name="evaluate_qa"):
# Run automatic evaluation with a set of built-in metrics for question-answering models
results = mlflow.evaluate(
data=eval_dataset,
model_type="question-answering",
)
print(results.tables["eval_results_table"])
MLflow Tracing provides LLM observability for various GenAI libraries such as OpenAI, LangChain, LlamaIndex, DSPy, AutoGen, and more. To enable auto-tracing, call mlflow.xyz.autolog()
before running your models. Refer to the documentation for customization and manual instrumentation.
import mlflow
from openai import OpenAI
# Enable tracing for OpenAI
mlflow.openai.autolog()
# Query OpenAI LLM normally
response = OpenAI().chat.completions.create(
model="gpt-4o-mini",
messages=[{"role": "user", "content": "Hi!"}],
temperature=0.1,
)
Then navigate to the "Traces" tab in the MLflow UI to find the trace records OpenAI query.
We happily welcome contributions to MLflow! We are also seeking contributions to items on the MLflow Roadmap. Please see our contribution guide to learn more about contributing to MLflow.
MLflow is currently maintained by the following core members with significant contributions from hundreds of exceptionally talented community members.
FAQs
MLflow is an open source platform for the complete machine learning lifecycle
We found that mlflow demonstrated a healthy version release cadence and project activity because the last version was released less than a year ago. It has 13 open source maintainers collaborating on the project.
Did you know?
Socket for GitHub automatically highlights issues in each pull request and monitors the health of all your open source dependencies. Discover the contents of your packages and block harmful activity before you install or update your dependencies.
Security News
Oracle seeks to dismiss fraud claims in the JavaScript trademark dispute, delaying the case and avoiding questions about its right to the name.
Security News
The Linux Foundation is warning open source developers that compliance with global sanctions is mandatory, highlighting legal risks and restrictions on contributions.
Security News
Maven Central now validates Sigstore signatures, making it easier for developers to verify the provenance of Java packages.