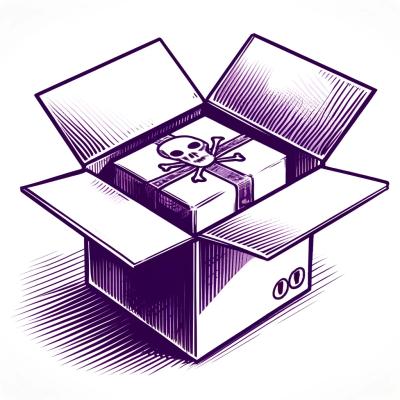
Security News
Research
Data Theft Repackaged: A Case Study in Malicious Wrapper Packages on npm
The Socket Research Team breaks down a malicious wrapper package that uses obfuscation to harvest credentials and exfiltrate sensitive data.
Documentation: https://supervised.mljar.com/
Source Code: https://github.com/mljar/mljar-supervised
Looking for commercial support: Please contact us by email for details
The mljar-supervised
is an Automated Machine Learning Python package that works with tabular data. It is designed to save time for a data scientist. It abstracts the common way to preprocess the data, construct the machine learning models, and perform hyper-parameters tuning to find the best model :trophy:. It is no black box, as you can see exactly how the ML pipeline is constructed (with a detailed Markdown report for each ML model).
The mljar-supervised
will help you with:
It has four built-in modes of work:
Explain
mode, which is ideal for explaining and understanding the data, with many data explanations, like decision trees visualization, linear models coefficients display, permutation importance, and SHAP explanations of data,Perform
for building ML pipelines to use in production,Compete
mode that trains highly-tuned ML models with ensembling and stacking, with the purpose to use in ML competitions.Optuna
mode can be used to search for highly-tuned ML models should be used when the performance is the most important, and computation time is not limited (it is available from version 0.10.0
)Of course, you can further customize the details of each mode
to meet the requirements.
Baseline
, Linear
, Random Forest
, Extra Trees
, LightGBM
, Xgboost
, CatBoost
, Neural Networks
, and Nearest Neighbors
.Compete
mode or after setting the stack_models
parameter).not-so-random-search
algorithm (random-search over a defined set of values) and hill climbing to fine-tune final models.Baseline
for your data so that you will know if you need Machine Learning or not!Decision Trees
with max_depth <= 5
, so you can easily visualize them with amazing dtreeviz to better understand your data.mljar-supervised
uses simple linear regression and includes its coefficients in the summary report, so you can check which features are used the most in the linear model.explain_level
parameter).mljar-supervised
creates markdown reports from AutoML training full of ML details, metrics, and charts.
We created a Web App with GUI, so you don't need to write any code 🐍. Just upload your data. Please check the Web App at github.com/mljar/automl-app. You can run this Web App locally on your computer, so your data is safe and secure :cat:
The report from running AutoML will contain the table with information about each model score and the time needed to train the model. There is a link for each model, which you can click to see the model's details. The performance of all ML models is presented as scatter and box plots so you can visually inspect which algorithms perform the best :trophy:.
Decision Tree
ReportThe example for Decision Tree
summary with trees visualization. For classification tasks, additional metrics are provided:
LightGBM
ReportThe example for LightGBM
summary:
In the docs you can find details about AutoML modes that are presented in the table.
automl = AutoML(mode="Explain")
It is aimed to be used when the user wants to explain and understand the data.
Baseline
, Linear
, Decision Tree
, Random Forest
, Xgboost
, `Neural Network' algorithms, and ensemble.automl = AutoML(mode="Perform")
It should be used when the user wants to train a model that will be used in real-life use cases.
Linear
, Random Forest
, LightGBM
, Xgboost
, CatBoost
, and Neural Network
. It uses ensembling.automl = AutoML(mode="Compete")
It should be used for machine learning competitions.
total_time_limit
. It can be: a train/test split (80/20), 5-fold CV or 10-fold CV.Linear
, Decision Tree
, Random Forest
, Extra Trees
, LightGBM
, Xgboost
, CatBoost
, Neural Network
, and Nearest Neighbors
. It uses ensemble and stacking.automl = AutoML(mode="Optuna", optuna_time_budget=3600)
It should be used when the performance is the most important and time is not limited.
Random Forest
, Extra Trees
, LightGBM
, Xgboost
, and CatBoost
. Those algorithms are tuned by Optuna
framework for optuna_time_budget
seconds, each. Algorithms are tuned with original data, without advanced feature engineering.All models in the AutoML are saved and loaded automatically. No need to call save()
or load()
.
automl = AutoML(results_path="AutoML_classifier")
automl.fit(X, y)
You will have all models saved in the AutoML_classifier
directory. Each model will have a separate directory with the README.md
file with all details from the training.
automl = AutoML(results_path="AutoML_classifier")
automl.predict(X)
The AutoML automatically loads models from the results_path
directory. If you will call fit()
on already trained AutoML then you will get a warning message that AutoML is already fitted.
All models are automatically saved to be able to restore the training after interruption. For example, you are training AutoML for 48 hours, and after 47 hours, there is some unexpected interruption. In MLJAR AutoML you just call the same training code after the interruption and AutoML reloads already trained models and finishes the training.
eval_metric
argument in AutoML()
)logloss
, auc
, f1
, average_precision
, accuracy
- default is logloss
logloss
, f1
, accuracy
- default is logloss
rmse
, mse
, mae
, r2
, mape
, spearman
, pearson
- default is rmse
If you don't find the eval_metric
that you need, please add a new issue. We will add it.
Starting from version 1.0.0
AutoML can optimize the Machine Learning pipeline with sensitive features. There are the following fairness related arguments in the AutoML constructor:
fairness_metric
- metric which will be used to decide if the model is fair,fairness_threshold
- threshold used in decision about model fairness,privileged_groups
- privileged groups used in fairness metrics computation,underprivileged_groups
- underprivileged groups used in fairness metrics computation.The fit()
method accepts sensitive_features
. When sensitive features are passed to AutoML, the best model will be selected among fair models only. In the AutoML reports, additional information about fairness metrics will be added. The MLJAR AutoML supports two methods for bias mitigation:
The fair ML building can be used with all algorithms, including Ensemble
and Stacked Ensemble
. We support three Machine Learning tasks:
Example code:
from sklearn.model_selection import train_test_split
from sklearn.datasets import fetch_openml
from supervised.automl import AutoML
data = fetch_openml(data_id=1590, as_frame=True)
X = data.data
y = (data.target == ">50K") * 1
sensitive_features = X[["sex"]]
X_train, X_test, y_train, y_test, S_train, S_test = train_test_split(
X, y, sensitive_features, stratify=y, test_size=0.75, random_state=42
)
automl = AutoML(
algorithms=[
"Xgboost"
],
train_ensemble=False,
fairness_metric="demographic_parity_ratio",
fairness_threshold=0.8,
privileged_groups = [{"sex": "Male"}],
underprivileged_groups = [{"sex": "Female"}],
)
automl.fit(X_train, y_train, sensitive_features=S_train)
You can read more about fairness aware AutoML training in our article https://mljar.com/blog/fairness-machine-learning/
There is a simple interface available with fit
and predict
methods.
import pandas as pd
from sklearn.model_selection import train_test_split
from supervised.automl import AutoML
df = pd.read_csv(
"https://raw.githubusercontent.com/pplonski/datasets-for-start/master/adult/data.csv",
skipinitialspace=True,
)
X_train, X_test, y_train, y_test = train_test_split(
df[df.columns[:-1]], df["income"], test_size=0.25
)
automl = AutoML()
automl.fit(X_train, y_train)
predictions = automl.predict(X_test)
AutoML fit
will print:
Create directory AutoML_1
AutoML task to be solved: binary_classification
AutoML will use algorithms: ['Baseline', 'Linear', 'Decision Tree', 'Random Forest', 'Xgboost', 'Neural Network']
AutoML will optimize for metric: logloss
1_Baseline final logloss 0.5519845471086654 time 0.08 seconds
2_DecisionTree final logloss 0.3655910192804364 time 10.28 seconds
3_Linear final logloss 0.38139916864708445 time 3.19 seconds
4_Default_RandomForest final logloss 0.2975204390214936 time 79.19 seconds
5_Default_Xgboost final logloss 0.2731086827200411 time 5.17 seconds
6_Default_NeuralNetwork final logloss 0.319812276905242 time 21.19 seconds
Ensemble final logloss 0.2731086821194617 time 1.43 seconds
The example code for classification of the optical recognition of handwritten digits dataset. Running this code in less than 30 minutes will result in test accuracy ~98%.
import pandas as pd
# scikit learn utilites
from sklearn.datasets import load_digits
from sklearn.metrics import accuracy_score
from sklearn.model_selection import train_test_split
# mljar-supervised package
from supervised.automl import AutoML
# load the data
digits = load_digits()
X_train, X_test, y_train, y_test = train_test_split(
pd.DataFrame(digits.data), digits.target, stratify=digits.target, test_size=0.25,
random_state=123
)
# train models with AutoML
automl = AutoML(mode="Perform")
automl.fit(X_train, y_train)
# compute the accuracy on test data
predictions = automl.predict_all(X_test)
print(predictions.head())
print("Test accuracy:", accuracy_score(y_test, predictions["label"].astype(int)))
Regression example on California Housing
house prices data.
import numpy as np
import pandas as pd
from sklearn.datasets import fetch_california_housing
from sklearn.model_selection import train_test_split
from sklearn.metrics import mean_squared_error
from supervised.automl import AutoML # mljar-supervised
# Load the data
housing = fetch_california_housing()
X_train, X_test, y_train, y_test = train_test_split(
pd.DataFrame(housing.data, columns=housing.feature_names),
housing.target,
test_size=0.25,
random_state=123,
)
# train models with AutoML
automl = AutoML(mode="Explain")
automl.fit(X_train, y_train)
# compute the MSE on test data
predictions = automl.predict(X_test)
print("Test MSE:", mean_squared_error(y_test, predictions))
The save and load of AutoML models is automatic. All models created during AutoML training are saved in the directory set in results_path
(argument of AutoML()
constructor). If there is no results_path
set, then the directory is created based on following name convention: AutoML_{number}
the number
will be number from 1 to 1000 (depends which directory name will be free).
Example save and load:
automl = AutoML(results_path='AutoML_1')
automl.fit(X, y)
The all models from AutoML are saved in AutoML_1
directory.
To load models:
automl = AutoML(results_path='AutoML_1')
automl.predict(X)
The MLJAR AutoML can work with:
The ML task detection is automatic based on target values. There can be situation if you want to manually force AutoML to select the ML task, then you need to set ml_task
parameter. It can be set to 'binary_classification'
, 'multiclass_classification'
, 'regression'
.
Example:
automl = AutoML(ml_task='regression')
automl.fit(X, y)
In the above example the regression model will be fitted.
You can reuse Optuna hyperparameters that were found in other AutoML training. You need to pass them in optuna_init_params
argument. All hyperparameters found during Optuna tuning are saved in the optuna/optuna.json
file (inside results_path
directory).
Example:
optuna_init = json.loads(open('previous_AutoML_training/optuna/optuna.json').read())
automl = AutoML(
mode='Optuna',
optuna_init_params=optuna_init
)
automl.fit(X, y)
When reusing Optuna hyperparameters the Optuna tuning is simply skipped. The model will be trained with hyperparameters set in optuna_init_params
. Right now there is no option to continue Optuna tuning with seed parameters.
To get predicted probabilites with information about class label please use the predict_all()
method. It returns the pandas DataFrame with class names in the columns. The order of predicted columns is the same in the predict_proba()
and predict_all()
methods. The predict_all()
method will additionaly have the column with the predicted class label.
For details please check mljar-supervised docs.
From PyPi repository:
pip install mljar-supervised
To install this package with conda run:
conda install -c conda-forge mljar-supervised
From source code:
git clone https://github.com/mljar/mljar-supervised.git
cd mljar-supervised
python setup.py install
Installation for development
git clone https://github.com/mljar/mljar-supervised.git
virtualenv venv --python=python3.6
source venv/bin/activate
pip install -r requirements.txt
pip install -r requirements_dev.txt
Running in the docker:
FROM python:3.7-slim-buster
RUN apt-get update && apt-get -y update
RUN apt-get install -y build-essential python3-pip python3-dev
RUN pip3 -q install pip --upgrade
RUN pip3 install mljar-supervised jupyter
CMD ["jupyter", "notebook", "--port=8888", "--no-browser", "--ip=0.0.0.0", "--allow-root"]
Install from GitHub with pip:
pip install -q -U git+https://github.com/mljar/mljar-supervised.git@master
In the below demo GIF you will see:
To get started take a look at our Contribution Guide for information about our process and where you can fit in!
Would you like to cite MLJAR? Great! :)
You can cite MLJAR as follows:
@misc{mljar,
author = {Aleksandra P\l{}o\'{n}ska and Piotr P\l{}o\'{n}ski},
year = {2021},
publisher = {MLJAR},
address = {\L{}apy, Poland},
title = {MLJAR: State-of-the-art Automated Machine Learning Framework for Tabular Data. Version 0.10.3},
url = {https://github.com/mljar/mljar-supervised}
}
Would love to hear from you about how have you used MLJAR AutoML in your project.
Please feel free to let us know at
The mljar-supervised
is provided with MIT license.
Looking for commercial support? Do you need new feature implementation? Please contact us by email for details.
The mljar-supervised
is an open-source project created by MLJAR. We care about ease of use in Machine Learning.
The mljar.com provides a beautiful and simple user interface for building machine learning models.
FAQs
Automated Machine Learning for Humans
We found that mljar-supervised demonstrated a healthy version release cadence and project activity because the last version was released less than a year ago. It has 1 open source maintainer collaborating on the project.
Did you know?
Socket for GitHub automatically highlights issues in each pull request and monitors the health of all your open source dependencies. Discover the contents of your packages and block harmful activity before you install or update your dependencies.
Security News
Research
The Socket Research Team breaks down a malicious wrapper package that uses obfuscation to harvest credentials and exfiltrate sensitive data.
Research
Security News
Attackers used a malicious npm package typosquatting a popular ESLint plugin to steal sensitive data, execute commands, and exploit developer systems.
Security News
The Ultralytics' PyPI Package was compromised four times in one weekend through GitHub Actions cache poisoning and failure to rotate previously compromised API tokens.