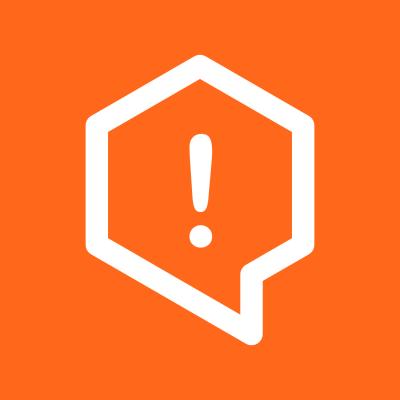
Security News
Fluent Assertions Faces Backlash After Abandoning Open Source Licensing
Fluent Assertions is facing backlash after dropping the Apache license for a commercial model, leaving users blindsided and questioning contributor rights.
Normalized Cut, aka. spectral clustering, is a graphical method to analyze data grouping in the affinity eigenvector space. It has been widely used for unsupervised segmentation in the 2000s.
Nyström Normalized Cut, is a new approximation algorithm developed for large-scale graph cuts, a large-graph of million nodes can be processed in under 10s (cpu) or 2s (gpu).
conda install pytorch torchvision torchaudio pytorch-cuda=11.8 -c pytorch -c nvidia
ncut-pytorch
pip install ncut-pytorch
In case of pip
install failed, please try install the build dependencies
Option A:
sudo apt-get update && sudo apt-get install build-essential cargo rustc -y
Option B:
conda install rust -c conda-forge
Option C:
curl --proto '=https' --tlsv1.2 -sSf https://sh.rustup.rs | sh && . "$HOME/.cargo/env"
Minimal example on how to run NCUT:
import torch
from ncut_pytorch import NCUT, rgb_from_tsne_3d
model_features = torch.rand(20, 64, 64, 768) # (B, H, W, C)
inp = model_features.reshape(-1, 768) # flatten
eigvectors, eigvalues = NCUT(num_eig=100, device='cuda:0').fit_transform(inp)
tsne_x3d, tsne_rgb = rgb_from_tsne_3d(eigvectors, device='cuda:0')
eigvectors = eigvectors.reshape(20, 64, 64, 100) # (B, H, W, num_eig)
tsne_rgb = tsne_rgb.reshape(20, 64, 64, 3) # (B, H, W, 3)
Any backbone model works as plug-in feature extractor. We have implemented some backbone models, here is a list of available models:
from ncut_pytorch.backbone import list_models
print(list_models())
[
'SAM2(sam2_hiera_t)', 'SAM2(sam2_hiera_s)', 'SAM2(sam2_hiera_b+)', 'SAM2(sam2_hiera_l)',
'SAM(sam_vit_b)', 'SAM(sam_vit_l)', 'SAM(sam_vit_h)', 'MobileSAM(TinyViT)',
'DiNOv2reg(dinov2_vits14_reg)', 'DiNOv2reg(dinov2_vitb14_reg)', 'DiNOv2reg(dinov2_vitl14_reg)', 'DiNOv2reg(dinov2_vitg14_reg)',
'DiNOv2(dinov2_vits14)', 'DiNOv2(dinov2_vitb14)', 'DiNOv2(dinov2_vitl14)', 'DiNOv2(dinov2_vitg14)',
'DiNO(dino_vits8_896)', 'DiNO(dino_vitb8_896)', 'DiNO(dino_vits8_672)', 'DiNO(dino_vitb8_672)', 'DiNO(dino_vits8_448)', 'DiNO(dino_vitb8_448)', 'DiNO(dino_vits16_448)', 'DiNO(dino_vitb16_448)',
'Diffusion(stabilityai/stable-diffusion-2)', 'Diffusion(CompVis/stable-diffusion-v1-4)', 'Diffusion(stabilityai/stable-diffusion-3-medium-diffusers)',
'CLIP(ViT-B-16/openai)', 'CLIP(ViT-L-14/openai)', 'CLIP(ViT-H-14/openai)', 'CLIP(ViT-B-16/laion2b_s34b_b88k)',
'CLIP(convnext_base_w_320/laion_aesthetic_s13b_b82k)', 'CLIP(convnext_large_d_320/laion2b_s29b_b131k_ft_soup)', 'CLIP(convnext_xxlarge/laion2b_s34b_b82k_augreg_soup)',
'CLIP(eva02_base_patch14_448/mim_in22k_ft_in1k)', "CLIP(eva02_large_patch14_448/mim_m38m_ft_in22k_in1k)",
'MAE(vit_base)', 'MAE(vit_large)', 'MAE(vit_huge)',
'ImageNet(vit_base)'
]
import torch
from ncut_pytorch import NCUT, rgb_from_tsne_3d
from ncut_pytorch.backbone import load_model, extract_features
model = load_model(model_name="SAM(sam_vit_b)")
images = torch.rand(20, 3, 1024, 1024)
model_features = extract_features(images, model, node_type='attn', layer=6)
# model_features = model(images)['attn'][6] # this also works
inp = model_features.reshape(-1, 768) # flatten
eigvectors, eigvalues = NCUT(num_eig=100, device='cuda:0').fit_transform(inp)
tsne_x3d, tsne_rgb = rgb_from_tsne_3d(eigvectors, device='cuda:0')
eigvectors = eigvectors.reshape(20, 64, 64, 100) # (B, H, W, num_eig)
tsne_rgb = tsne_rgb.reshape(20, 64, 64, 3) # (B, H, W, 3)
import os
from ncut_pytorch import NCUT, rgb_from_tsne_3d
from ncut_pytorch.backbone_text import load_text_model
os.environ['HF_ACCESS_TOKEN'] = "your_huggingface_token"
llama = load_text_model("meta-llama/Meta-Llama-3.1-8B").cuda()
output_dict = llama("The quick white fox jumps over the lazy cat.")
model_features = output_dict['block'][31].squeeze(0) # 32nd block output
token_texts = output_dict['token_texts']
eigvectors, eigvalues = NCUT(num_eig=5, device='cuda:0').fit_transform(model_features)
tsne_x3d, tsne_rgb = rgb_from_tsne_3d(eigvectors, device='cuda:0')
# eigvectors.shape[0] == tsne_rgb.shape[0] == len(token_texts)
paper in prep, Yang 2024
AlignedCut: Visual Concepts Discovery on Brain-Guided Universal Feature Space, Huzheng Yang, James Gee*, Jianbo Shi*,2024
Normalized Cuts and Image Segmentation, Jianbo Shi and Jitendra Malik, 2000
FAQs
Unknown package
We found that ncut-pytorch demonstrated a healthy version release cadence and project activity because the last version was released less than a year ago. It has 1 open source maintainer collaborating on the project.
Did you know?
Socket for GitHub automatically highlights issues in each pull request and monitors the health of all your open source dependencies. Discover the contents of your packages and block harmful activity before you install or update your dependencies.
Security News
Fluent Assertions is facing backlash after dropping the Apache license for a commercial model, leaving users blindsided and questioning contributor rights.
Research
Security News
Socket researchers uncover the risks of a malicious Python package targeting Discord developers.
Security News
The UK is proposing a bold ban on ransomware payments by public entities to disrupt cybercrime, protect critical services, and lead global cybersecurity efforts.