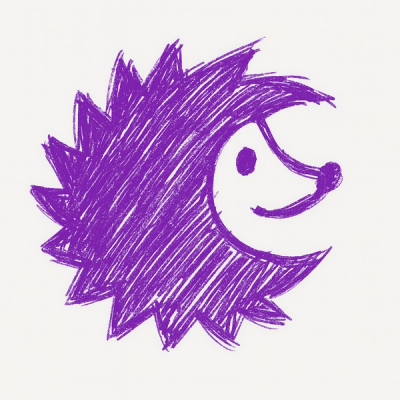
Security News
Browserslist-rs Gets Major Refactor, Cutting Binary Size by Over 1MB
Browserslist-rs now uses static data to reduce binary size by over 1MB, improving memory use and performance for Rust-based frontend tools.
.. image:: https://img.shields.io/pypi/v/npstructures.svg :target: https://pypi.python.org/pypi/npstructures
.. image:: https://github.com/knutdrand/npstructures/actions/workflows/python-install-and-test.yml/badge.svg :target: https://github.com/knutdrand/npstructures/actions/workflows/python-install-and-test.yml
.. image:: https://readthedocs.org/projects/npstructures/badge/?version=latest :target: https://npstructures.readthedocs.io/en/latest/?version=latest :alt: Documentation Status
Simple data structures that augments the numpy library
The main feature is the RaggedArray
class which enables numpy
-like behaviour and performance for arrays where
the length of the rows differ.
RaggedArray
is meant as a drop-in replacement for numpy
when you have arrays with differing row lengths.
As such, familiarity with numpy
is assumed. The simplest way to construct a RaggedArray
is from a list of lists::
>>> from npstructures import RaggedArray
>>> ra = RaggedArray([[1, 2], [4, 1, 3, 7], [9], [8, 7, 3, 4]])
A RaggedArray
can be indexed much like a numpy
array::
>>> ra[1]
array([4, 1, 3, 7])
>>> ra[1, 3]
7
>>> ra[1:3]
RaggedArray([[4, 1, 3, 7], [9]])
>>> ra[[0, 3]]
RaggedArray([[1, 2], [8, 7, 3, 4]])
>>> ra[0] = [0, 0]
>>> ra
RaggedArray([[0, 0], [4, 1, 3, 7], [9], [8, 7, 3, 4]])
>>> ra[1:3] = [[10], [20]]
>>> ra
RaggedArray([[0, 0], [10, 10, 10, 10], [20], [8, 7, 3, 4]])
>>> ra[[0, 2, 3]] = RaggedArray([[2, 2], [3], [5, 5, 5, 5]])
>>> ra
RaggedArray([[2, 2], [10, 10, 10, 10], [3], [5, 5, 5, 5]])
numpy ufuncs
can be applied to RaggedArray
objects::
>>> ra + 1
RaggedArray([[2, 3], [5, 2, 4, 8], [10], [9, 8, 4, 5]])
>>> ra*2
RaggedArray([[2, 4], [8, 2, 6, 14], [18], [16, 14, 6, 8]])
>>> ra + [[1], [10], [100], [1000]]
RaggedArray([[2, 3], [14, 11, 13, 17], [109], [1008, 1007, 1003, 1004]])
>>> ra - (ra*2)
RaggedArray([[-1, -2], [-4, -1, -3, -7], [-9], [-8, -7, -3, -4]])
Some numpy
functions can be applied to RaggedArray
objects::
>>> import numpy as np
>>> ra = RaggedArray([[1, 2], [4, 1, 3, 7], [9], [8, 7, 3, 4]])
>>> np.concatenate((ra, ra*10))
RaggedArray([[1, 2], [4, 1, 3, 7], [9], [8, 7, 3, 4], [10, 20], [40, 10, 30, 70], [90], [80, 70, 30, 40]])
>>> np.nonzero(ra>3)
(array([1, 1, 2, 3, 3, 3]), array([0, 3, 0, 0, 1, 3]))
>>> np.ones_like(ra)
RaggedArray([[1, 1], [1, 1, 1, 1], [1], [1, 1, 1, 1]])
In addition to this. HashTable
and Counter
provides simple dict
-like behaviour for numpy
arrays:
HashTable
can be used for dict
-like functionality of numpy
arrays. The simplest way to construct a HashTable
is from an array of keys and an array of values (note that the set of keys cannot be modified after the initialization of the object)::
>>> table = HashTable([11, 113, 1191, 11199], [2, 3, 5, 7])
>>> table[11]
array([2])
>>> table[[113, 11199]]
array([3, 7])
>>> table[11]=1000
>>> table
HashTable([ 113 1191 11 11199], [ 3 5 1000 7])
>>> table[[113, 1191]]=2000
>>> table
HashTable([ 113 1191 11 11199], [2000 2000 1000 7])
>>> table[[113, 1191, 11, 11191]] = [1, 2, 3, 4]
>>> table[[113, 1191, 11, 11199]] = [1, 2, 3, 4]
>>> table
HashTable([ 113 1191 11 11199], [1 2 3 4])
Counter
objects supports counting the occurances of a predefined set of keys in a set of samples. For instance, to count the occurances of 3
and 1
in the list [3, 2, 1, 3, 4, 1, 1]
::
>>> from npstructures import Counter
>>> counter = Counter([3, 1])
>>> counter.count([3, 2, 1, 3, 4, 1, 1])
>>> counter
Counter([3 1], [2 3])
This package was created with Cookiecutter_ and the audreyr/cookiecutter-pypackage
_ project template.
.. _Cookiecutter: https://github.com/audreyr/cookiecutter
.. _audreyr/cookiecutter-pypackage
: https://github.com/audreyr/cookiecutter-pypackage
FAQs
Simple data structures that augments the numpy library
We found that npstructures demonstrated a healthy version release cadence and project activity because the last version was released less than a year ago. It has 1 open source maintainer collaborating on the project.
Did you know?
Socket for GitHub automatically highlights issues in each pull request and monitors the health of all your open source dependencies. Discover the contents of your packages and block harmful activity before you install or update your dependencies.
Security News
Browserslist-rs now uses static data to reduce binary size by over 1MB, improving memory use and performance for Rust-based frontend tools.
Research
Security News
Eight new malicious Firefox extensions impersonate games, steal OAuth tokens, hijack sessions, and exploit browser permissions to spy on users.
Security News
The official Go SDK for the Model Context Protocol is in development, with a stable, production-ready release expected by August 2025.