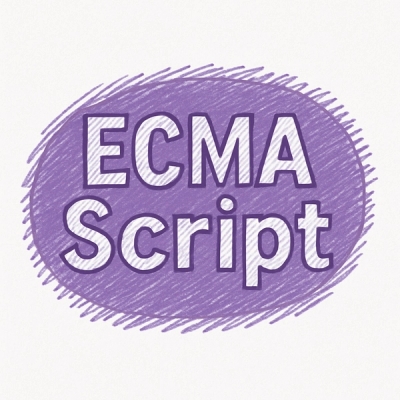
Security News
ECMAScript 2025 Finalized with Iterator Helpers, Set Methods, RegExp.escape, and More
ECMAScript 2025 introduces Iterator Helpers, Set methods, JSON modules, and more in its latest spec update approved by Ecma in June 2025.
A self-generalizing gradient boosting machine that doesn't need hyperparameter optimization
PerpetualBooster is a gradient boosting machine (GBM) algorithm that doesn't need hyperparameter optimization unlike other GBM algorithms. Similar to AutoML libraries, it has a budget
parameter. Increasing the budget
parameter increases the predictive power of the algorithm and gives better results on unseen data. Start with a small budget (e.g. 0.5) and increase it (e.g. 1.0) once you are confident with your features. If you don't see any improvement with further increasing the budget
, it means that you are already extracting the most predictive power out of your data.
You can use the algorithm like in the example below. Check examples folders for both Rust and Python.
from perpetual import PerpetualBooster
model = PerpetualBooster(objective="SquaredLoss", budget=0.5)
model.fit(X, y)
Documentation for the Python API can be found here and for the Rust API here.
Hyperparameter optimization usually takes 100 iterations with plain GBM algorithms. PerpetualBooster achieves the same accuracy in a single run. Thus, it achieves up to 100x speed-up at the same accuracy with different budget
levels and with different datasets.
The following table summarizes the results for the California Housing dataset (regression):
Perpetual budget | LightGBM n_estimators | Perpetual mse | LightGBM mse | Speed-up wall time | Speed-up cpu time |
---|---|---|---|---|---|
1.0 | 100 | 0.192 | 0.192 | 54x | 56x |
1.5 | 300 | 0.188 | 0.188 | 59x | 58x |
2.1 | 1000 | 0.185 | 0.186 | 42x | 41x |
The following table summarizes the results for the Cover Types dataset (classification):
Perpetual budget | LightGBM n_estimators | Perpetual log loss | LightGBM log loss | Speed-up wall time | Speed-up cpu time |
---|---|---|---|---|---|
0.9 | 100 | 0.091 | 0.084 | 72x | 78x |
The results can be reproduced using the scripts in the examples folder.
PerpetualBooster is a GBM but behaves like AutoML so it is benchmarked also against AutoGluon (v1.2, best quality preset), the current leader in AutoML benchmark. Top 10 datasets with the most number of rows are selected from OpenML datasets for both regression and classification tasks.
The results are summarized in the following table for regression tasks:
OpenML Task | Perpetual Training Duration | Perpetual Inference Duration | Perpetual RMSE | AutoGluon Training Duration | AutoGluon Inference Duration | AutoGluon RMSE |
---|---|---|---|---|---|---|
Airlines_DepDelay_10M | 518 | 11.3 | 29.0 | 520 | 30.9 | 28.8 |
bates_regr_100 | 3421 | 15.1 | 1.084 | OOM | OOM | OOM |
BNG(libras_move) | 1956 | 4.2 | 2.51 | 1922 | 97.6 | 2.53 |
BNG(satellite_image) | 334 | 1.6 | 0.731 | 337 | 10.0 | 0.721 |
COMET_MC | 44 | 1.0 | 0.0615 | 47 | 5.0 | 0.0662 |
friedman1 | 275 | 4.2 | 1.047 | 278 | 5.1 | 1.487 |
poker | 38 | 0.6 | 0.256 | 41 | 1.2 | 0.722 |
subset_higgs | 868 | 10.6 | 0.420 | 870 | 24.5 | 0.421 |
BNG(autoHorse) | 107 | 1.1 | 19.0 | 107 | 3.2 | 20.5 |
BNG(pbc) | 48 | 0.6 | 836.5 | 51 | 0.2 | 957.1 |
average | 465 | 3.9 | - | 464 | 19.7 | - |
PerpetualBooster outperformed AutoGluon on 8 out of 10 regression tasks, training equally fast and inferring 5.1x faster.
The results are summarized in the following table for classification tasks:
OpenML Task | Perpetual Training Duration | Perpetual Inference Duration | Perpetual AUC | AutoGluon Training Duration | AutoGluon Inference Duration | AutoGluon AUC |
---|---|---|---|---|---|---|
BNG(spambase) | 70.1 | 2.1 | 0.671 | 73.1 | 3.7 | 0.669 |
BNG(trains) | 89.5 | 1.7 | 0.996 | 106.4 | 2.4 | 0.994 |
breast | 13699.3 | 97.7 | 0.991 | 13330.7 | 79.7 | 0.949 |
Click_prediction_small | 89.1 | 1.0 | 0.749 | 101.0 | 2.8 | 0.703 |
colon | 12435.2 | 126.7 | 0.997 | 12356.2 | 152.3 | 0.997 |
Higgs | 3485.3 | 40.9 | 0.843 | 3501.4 | 67.9 | 0.816 |
SEA(50000) | 21.9 | 0.2 | 0.936 | 25.6 | 0.5 | 0.935 |
sf-police-incidents | 85.8 | 1.5 | 0.687 | 99.4 | 2.8 | 0.659 |
bates_classif_100 | 11152.8 | 50.0 | 0.864 | OOM | OOM | OOM |
prostate | 13699.9 | 79.8 | 0.987 | OOM | OOM | OOM |
average | 3747.0 | 34.0 | - | 3699.2 | 39.0 | - |
PerpetualBooster outperformed AutoGluon on 10 out of 10 classification tasks, training equally fast and inferring 1.1x faster.
PerpetualBooster demonstrates greater robustness compared to AutoGluon, successfully training on all 20 tasks, whereas AutoGluon encountered out-of-memory errors on 3 of those tasks.
The results can be reproduced using the automlbenchmark fork here.
The package can be installed directly from pypi:
pip install perpetual
Using conda-forge:
conda install conda-forge::perpetual
To use in a Rust project and to get the package from crates.io:
cargo add perpetual
Contributions are welcome. Check CONTRIBUTING.md for the guideline.
PerpetualBooster prevents overfitting with a generalization algorithm. The paper is work-in-progress to explain how the algorithm works. Check our blog post for a high level introduction to the algorithm.
FAQs
A self-generalizing gradient boosting machine that doesn't need hyperparameter optimization
We found that perpetual demonstrated a healthy version release cadence and project activity because the last version was released less than a year ago. It has 1 open source maintainer collaborating on the project.
Did you know?
Socket for GitHub automatically highlights issues in each pull request and monitors the health of all your open source dependencies. Discover the contents of your packages and block harmful activity before you install or update your dependencies.
Security News
ECMAScript 2025 introduces Iterator Helpers, Set methods, JSON modules, and more in its latest spec update approved by Ecma in June 2025.
Security News
A new Node.js homepage button linking to paid support for EOL versions has sparked a heated discussion among contributors and the wider community.
Research
North Korean threat actors linked to the Contagious Interview campaign return with 35 new malicious npm packages using a stealthy multi-stage malware loader.