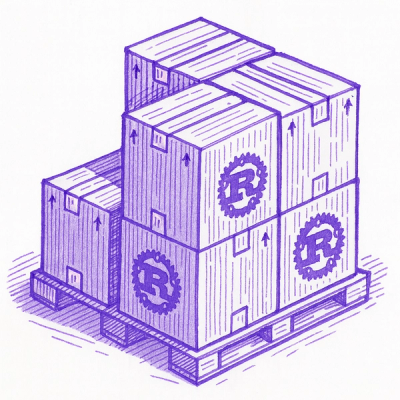
Security News
Crates.io Implements Trusted Publishing Support
Crates.io adds Trusted Publishing support, enabling secure GitHub Actions-based crate releases without long-lived API tokens.
python wrapper of pnnx, only support python 3.7+ now.
pnnx is available as wheel packages for macOS, Windows and Linux distributions, you can install with pip:
pip install pnnx
On Unix (Linux, OS X)
On Mac
On Windows
git clone https://github.com/Tencent/ncnn.git
install pytorch according to https://pytorch.org/ . Anaconda is strongly recommended for example:
conda install pytorch
cd /pathto/ncnntools/pnnx/python
python setup.py install
Note: If torchvision and pnnx2onnx are needed, you can set the following environment variables before 'python setup.py install' to enable them. e.g. on ubuntu:
export TORCHVISION_INSTALL_DIR="/project/torchvision" export PROTOBUF_INCLUDE_DIR="/project/protobuf/include" export PROTOBUF_LIBRARIES="/project/protobuf/lib64/libprotobuf.a" export PROTOBUF_PROTOC_EXECUTABLE="/project/protobuf/bin/protoc"
To do these, you must install Torchvision and Protobuf first.
cd /pathto/ncnn/tools/pnnx/python
pytest tests
import torch
import torchvision.models as models
import pnnx
net = models.resnet18(pretrained=True)
x = torch.rand(1, 3, 224, 224)
# You could try disabling checking when torch tracing raises error
# opt_net = pnnx.export(net, "resnet18.pt", x, check_trace=False)
opt_net = pnnx.export(net, "resnet18.pt", x)
import torch
import pnnx
x = torch.rand(1, 3, 224, 224)
opt_net = pnnx.convert("resnet18.pt", x)
model
(torch.nn.Model): model to be exported.
ptpath
(str): the torchscript name.
inputs
(torch.Tensor of list of torch.Tensor) expected inputs of the model.
inputs2
(torch.Tensor of list of torch.Tensor) alternative inputs of the model. Usually, it is used with input_shapes to resolve dynamic shape.
input_shapes
(Optional, list of int or list of list with int type inside) shapes of model inputs.
It is used to resolve tensor shapes in model graph. for example, [1,3,224,224] for the model with only
1 input, [[1,3,224,224],[1,3,224,224]] for the model that have 2 inputs.
input_types
(Optional, str or list of str) types of model inputs, it should have the same length with input_shapes
.
for example, "f32" for the model with only 1 input, ["f32", "f32"] for the model that have 2 inputs.
typename | torch type |
---|---|
f32 | torch.float32 or torch.float |
f64 | torch.float64 or torch.double |
f16 | torch.float16 or torch.half |
u8 | torch.uint8 |
i8 | torch.int8 |
i16 | torch.int16 or torch.short |
i32 | torch.int32 or torch.int |
i64 | torch.int64 or torch.long |
c32 | torch.complex32 |
c64 | torch.complex64 |
c128 | torch.complex128 |
input_shapes2
(Optional, list of int or list of list with int type inside) shapes of alternative model inputs,
the format is identical to input_shapes
. Usually, it is used with input_shapes to resolve dynamic shape (-1)
in model graph.
input_types2
(Optional, str or list of str) types of alternative model inputs.
device
(Optional, str, default="cpu") device type for the input in TorchScript model, cpu or gpu.
customop
(Optional, str or list of str) list of Torch extensions (dynamic library) for custom operators.
For example, "/home/nihui/.cache/torch_extensions/fused/fused.so" or
["/home/nihui/.cache/torch_extensions/fused/fused.so",...].
moduleop
(Optional, str or list of str) list of modules to keep as one big operator.
for example, "models.common.Focus" or ["models.common.Focus","models.yolo.Detect"].
optlevel
(Optional, int, default=2) graph optimization level
option | optimization level |
---|---|
0 | do not apply optimization |
1 | do not apply optimization |
2 | optimization more for inference |
pnnxparam
(Optional, str, default="*.pnnx.param", * is the model name): PNNX graph definition file.
pnnxbin
(Optional, str, default="*.pnnx.bin"): PNNX model weight.
pnnxpy
(Optional, str, default="*_pnnx.py"): PyTorch script for inference, including model construction
and weight initialization code.
pnnxonnx
(Optional, str, default="*.pnnx.onnx"): PNNX model in onnx format.
ncnnparam
(Optional, str, default="*.ncnn.param"): ncnn graph definition.
ncnnbin
(Optional, str, default="*.ncnn.bin"): ncnn model weight.
ncnnpy
(Optional, str, default="*_ncnn.py"): pyncnn script for inference.
ptpath
(str): torchscript model to be converted.
Other parameters are consistent with pnnx.export
FAQs
pnnx is an open standard for PyTorch model interoperability.
We found that pnnx demonstrated a healthy version release cadence and project activity because the last version was released less than a year ago. It has 2 open source maintainers collaborating on the project.
Did you know?
Socket for GitHub automatically highlights issues in each pull request and monitors the health of all your open source dependencies. Discover the contents of your packages and block harmful activity before you install or update your dependencies.
Security News
Crates.io adds Trusted Publishing support, enabling secure GitHub Actions-based crate releases without long-lived API tokens.
Research
/Security News
Undocumented protestware found in 28 npm packages disrupts UI for Russian-language users visiting Russian and Belarusian domains.
Research
/Security News
North Korean threat actors deploy 67 malicious npm packages using the newly discovered XORIndex malware loader.