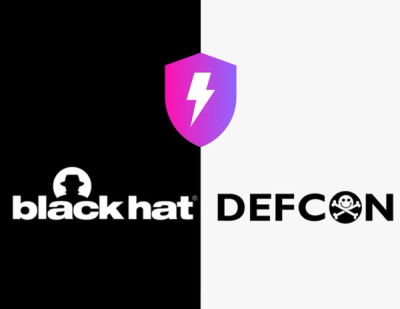
Security News
Meet Socket at Black Hat and DEF CON 2025 in Las Vegas
Meet Socket at Black Hat & DEF CON 2025 for 1:1s, insider security talks at Allegiant Stadium, and a private dinner with top minds in software supply chain security.
This tool is designed to compute the theoretical amount of multiply-add operations in neural networks. It can also compute the number of parameters and print per-layer computational cost of a given network.
ptflops
has two backends, pytorch
and aten
. pytorch
backend is a legacy one, it considers nn.Modules
only. However,
it's still useful, since it provides a better par-layer analytics for CNNs. In all other cases it's recommended to use
aten
backend, which considers aten operations, and therefore it covers more model architectures (including transformers).
The default backend is aten
. Please, don't use pytorch
backend for transformer architectures.
aten
backendverbose=True
to see the operations which were not considered during complexity computation.nn.Module
.
Deeper modules at the second level of nesting are not shown in the per-layer statistics.ignore_modules
option forces ptflops
to ignore the listed modules. This can be useful
for research purposes. For instance, one can drop all convolutions from the counting process
specifying ignore_modules=[torch.ops.aten.convolution, torch.ops.aten._convolution]
.pytorch
backendExperimental support:
torch.nn.functional.*
and tensor.*
operations. Therefore unsupported operations are
not contributing to the final complexity estimation. See ptflops/pytorch_ops.py:FUNCTIONAL_MAPPING,TENSOR_OPS_MAPPING
to check supported ops.
Sometimes considering functional style conflicts with hooks for nn.Module
(for instance, custom ones). In that case, counting with these ops can be disabled by
passing backend_specific_config={"count_functional" : False}
.ptflops
launches a given model on a random tensor and estimates amount of computations during inference. Complicated models can have several inputs, some of them could be optional. To construct non-trivial input one can use the input_constructor
argument of the get_model_complexity_info
. input_constructor
is a function that takes the input spatial resolution as a tuple and returns a dict with named input arguments of the model. Next, this dict would be passed to the model as a keyword arguments.verbose
parameter allows to get information about modules that don't contribute to the final numbers.ignore_modules
option forces ptflops
to ignore the listed modules. This can be useful
for research purposes. For instance, one can drop all convolutions from the counting process
specifying ignore_modules=[torch.nn.Conv2d]
.Pytorch >= 2.0. Use pip install ptflops==0.7.2.2
to work with torch 1.x.
From PyPI:
pip install ptflops
From this repository:
pip install --upgrade git+https://github.com/sovrasov/flops-counter.pytorch.git
import torchvision.models as models
import torch
from ptflops import get_model_complexity_info
with torch.cuda.device(0):
net = models.densenet161()
macs, params = get_model_complexity_info(net, (3, 224, 224), as_strings=True, backend='pytorch'
print_per_layer_stat=True, verbose=True)
print('{:<30} {:<8}'.format('Computational complexity: ', macs))
print('{:<30} {:<8}'.format('Number of parameters: ', params))
macs, params = get_model_complexity_info(net, (3, 224, 224), as_strings=True, backend='aten'
print_per_layer_stat=True, verbose=True)
print('{:<30} {:<8}'.format('Computational complexity: ', macs))
print('{:<30} {:<8}'.format('Number of parameters: ', params))
If ptflops was useful for your paper or tech report, please cite me:
@online{ptflops,
author = {Vladislav Sovrasov},
title = {ptflops: a flops counting tool for neural networks in pytorch framework},
year = 2018-2024,
url = {https://github.com/sovrasov/flops-counter.pytorch},
}
Thanks to @warmspringwinds and Horace He for the initial version of the script.
Model | Input Resolution | Params(M) | MACs(G) (pytorch ) | MACs(G) (aten ) |
---|---|---|---|---|
alexnet | 224x224 | 61.10 | 0.72 | 0.71 |
convnext_base | 224x224 | 88.59 | 15.43 | 15.38 |
densenet121 | 224x224 | 7.98 | 2.90 | |
efficientnet_b0 | 224x224 | 5.29 | 0.41 | |
efficientnet_v2_m | 224x224 | 54.14 | 5.43 | |
googlenet | 224x224 | 13.00 | 1.51 | |
inception_v3 | 224x224 | 27.16 | 5.75 | 5.71 |
maxvit_t | 224x224 | 30.92 | 5.48 | |
mnasnet1_0 | 224x224 | 4.38 | 0.33 | |
mobilenet_v2 | 224x224 | 3.50 | 0.32 | |
mobilenet_v3_large | 224x224 | 5.48 | 0.23 | |
regnet_y_1_6gf | 224x224 | 11.20 | 1.65 | |
resnet18 | 224x224 | 11.69 | 1.83 | 1.81 |
resnet50 | 224x224 | 25.56 | 4.13 | 4.09 |
resnext50_32x4d | 224x224 | 25.03 | 4.29 | |
shufflenet_v2_x1_0 | 224x224 | 2.28 | 0.15 | |
squeezenet1_0 | 224x224 | 1.25 | 0.84 | 0.82 |
vgg16 | 224x224 | 138.36 | 15.52 | 15.48 |
vit_b_16 | 224x224 | 86.57 | 17.61 (wrong) | 16.86 |
wide_resnet50_2 | 224x224 | 68.88 | 11.45 |
Model | Input Resolution | Params(M) | MACs(G)
FAQs
Flops counter for neural networks in pytorch framework
We found that ptflops demonstrated a healthy version release cadence and project activity because the last version was released less than a year ago. It has 1 open source maintainer collaborating on the project.
Did you know?
Socket for GitHub automatically highlights issues in each pull request and monitors the health of all your open source dependencies. Discover the contents of your packages and block harmful activity before you install or update your dependencies.
Security News
Meet Socket at Black Hat & DEF CON 2025 for 1:1s, insider security talks at Allegiant Stadium, and a private dinner with top minds in software supply chain security.
Security News
CAI is a new open source AI framework that automates penetration testing tasks like scanning and exploitation up to 3,600× faster than humans.
Security News
Deno 2.4 brings back bundling, improves dependency updates and telemetry, and makes the runtime more practical for real-world JavaScript projects.