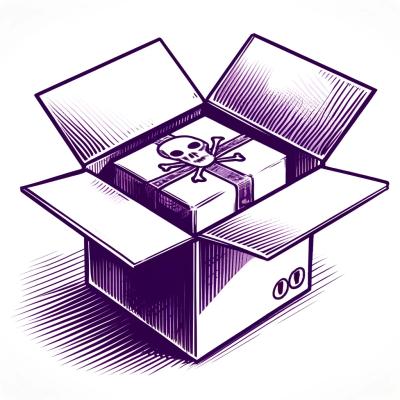
Security News
Research
Data Theft Repackaged: A Case Study in Malicious Wrapper Packages on npm
The Socket Research Team breaks down a malicious wrapper package that uses obfuscation to harvest credentials and exfiltrate sensitive data.
In large-scale surveys, often complex random mechanisms are used to select samples. Estimates derived from such samples must reflect the random mechanism. Samplics is a python package that implements a set of sampling techniques for complex survey designs. These survey sampling techniques are organized into the following four sub-packages.
Sampling provides a set of random selection techniques used to draw a sample from a population. It also provides procedures for calculating sample sizes. The sampling subpackage contains:
Weighting provides the procedures for adjusting sample weights. More specifically, the weighting subpackage allows the following:
Estimation provides methods for estimating the parameters of interest with uncertainty measures that are consistent with the sampling design. The estimation subpackage implements the following types of estimation methods:
Small Area Estimation (SAE). When the sample size is not large enough to produce reliable / stable domain level estimates, SAE techniques can be used to model the output variable of interest to produce domain level estimates. This subpackage provides Area-level and Unit-level SAE methods.
For more details, visit https://samplics-org.github.io/samplics/
Let's assume that we have a population and we would like to select a sample from it. The goal is to calculate the sample size for an expected proportion of 0.80 with a precision (half confidence interval) of 0.10.
from samplics.sampling import SampleSize
sample_size = SampleSize(parameter = "proportion")
sample_size.calculate(target=0.80, half_ci=0.10)
Furthermore, the population is located in four natural regions i.e. North, South, East, and West. We could be interested in calculating sample sizes based on region specific requirements e.g. expected proportions, desired precisions and associated design effects.
from samplics.sampling import SampleSize
sample_size = SampleSize(parameter="proportion", method="wald", strat=True)
expected_proportions = {"North": 0.95, "South": 0.70, "East": 0.30, "West": 0.50}
half_ci = {"North": 0.30, "South": 0.10, "East": 0.15, "West": 0.10}
deff = {"North": 1, "South": 1.5, "East": 2.5, "West": 2.0}
sample_size = SampleSize(parameter = "proportion", method="Fleiss", strat=True)
sample_size.calculate(target=expected_proportions, half_ci=half_ci, deff=deff)
To select a sample of primary sampling units using PPS method,
we can use code similar to the snippets below. Note that we first use the datasets
module to import the example dataset.
# First we import the example dataset
from samplics.datasets import load_psu_frame
psu_frame_dict = load_psu_frame()
psu_frame = psu_frame_dict["data"]
# Code for the sample selection
from samplics.sampling import SampleSelection
from samplics.utils import SelectMethod
psu_sample_size = {"East":3, "West": 2, "North": 2, "South": 3}
pps_design = SampleSelection(
method=SelectMethod.pps_sys,
strat=True,
wr=False
)
psu_frame["psu_prob"] = pps_design.inclusion_probs(
psu_frame["cluster"],
psu_sample_size,
psu_frame["region"],
psu_frame["number_households_census"]
)
The initial weighting step is to obtain the design sample weights. In this example, we show a simple example of two-stage sampling design.
import pandas as pd
from samplics.datasets import load_psu_sample, load_ssu_sample
from samplics.weighting import SampleWeight
# Load PSU sample data
psu_sample_dict = load_psu_sample()
psu_sample = psu_sample_dict["data"]
# Load PSU sample data
ssu_sample_dict = load_ssu_sample()
ssu_sample = ssu_sample_dict["data"]
full_sample = pd.merge(
psu_sample[["cluster", "region", "psu_prob"]],
ssu_sample[["cluster", "household", "ssu_prob"]],
on="cluster"
)
full_sample["inclusion_prob"] = full_sample["psu_prob"] * full_sample["ssu_prob"]
full_sample["design_weight"] = 1 / full_sample["inclusion_prob"]
To adjust the design sample weight for nonresponse, we can use code similar to:
import numpy as np
from samplics.weighting import SampleWeight
# Simulate response
np.random.seed(7)
full_sample["response_status"] = np.random.choice(
["ineligible", "respondent", "non-respondent", "unknown"],
size=full_sample.shape[0],
p=(0.10, 0.70, 0.15, 0.05),
)
# Map custom response statuses to teh generic samplics statuses
status_mapping = {
"in": "ineligible",
"rr": "respondent",
"nr": "non-respondent",
"uk":"unknown"
}
# adjust sample weights
full_sample["nr_weight"] = SampleWeight().adjust(
samp_weight=full_sample["design_weight"],
adjust_class=full_sample["region"],
resp_status=full_sample["response_status"],
resp_dict=status_mapping
)
To estimate population parameters using Taylor-based and replication-based methods, we can use code similar to:
# Taylor-based
from samplics.datasets import load_nhanes2
nhanes2_dict = load_nhanes2()
nhanes2 = nhanes2_dict["data"]
from samplics.estimation import TaylorEstimator
zinc_mean_str = TaylorEstimator("mean")
zinc_mean_str.estimate(
y=nhanes2["zinc"],
samp_weight=nhanes2["finalwgt"],
stratum=nhanes2["stratid"],
psu=nhanes2["psuid"],
remove_nan=True,
)
# Replicate-based
from samplics.datasets import load_nhanes2brr
nhanes2brr_dict = load_nhanes2brr()
nhanes2brr = nhanes2brr_dict["data"]
from samplics.estimation import ReplicateEstimator
ratio_wgt_hgt = ReplicateEstimator("brr", "ratio").estimate(
y=nhanes2brr["weight"],
samp_weight=nhanes2brr["finalwgt"],
x=nhanes2brr["height"],
rep_weights=nhanes2brr.loc[:, "brr_1":"brr_32"],
remove_nan=True,
)
To predict small area parameters, we can use code similar to:
import numpy as np
import pandas as pd
# Area-level basic method
from samplics.datasets import load_expenditure_milk
milk_exp_dict = load_expenditure_milk()
milk_exp = milk_exp_dict["data"]
from samplics.sae import EblupAreaModel
fh_model_reml = EblupAreaModel(method="REML")
fh_model_reml.fit(
yhat=milk_exp["direct_est"],
X=pd.get_dummies(milk_exp["major_area"], drop_first=True),
area=milk_exp["small_area"],
error_std=milk_exp["std_error"],
intercept=True,
tol=1e-8,
)
fh_model_reml.predict(
X=pd.get_dummies(milk_exp["major_area"], drop_first=True),
area=milk_exp["small_area"],
intercept=True,
)
# Unit-level basic method
from samplics.datasets import load_county_crop, load_county_crop_means
# Load County Crop sample data
countycrop_dict = load_county_crop()
countycrop = countycrop_dict["data"]
# Load County Crop Area Means sample data
countycropmeans_dict = load_county_crop_means()
countycrop_means = countycropmeans_dict["data"]
from samplics.sae import EblupUnitModel
eblup_bhf_reml = EblupUnitModel()
eblup_bhf_reml.fit(
countycrop["corn_area"],
countycrop[["corn_pixel", "soybeans_pixel"]],
countycrop["county_id"],
)
eblup_bhf_reml.predict(
Xmean=countycrop_means[["ave_corn_pixel", "ave_corn_pixel"]],
area=np.linspace(1, 12, 12),
)
pip install samplics
Python 3.7 or newer is required and the main dependencies are numpy, pandas, scpy, and statsmodel.
If you would like to contribute to the project, please read contributing to samplics
created by Mamadou S. Diallo - feel free to contact me!
FAQs
Select, weight and analyze complex sample data
We found that samplics demonstrated a healthy version release cadence and project activity because the last version was released less than a year ago. It has 1 open source maintainer collaborating on the project.
Did you know?
Socket for GitHub automatically highlights issues in each pull request and monitors the health of all your open source dependencies. Discover the contents of your packages and block harmful activity before you install or update your dependencies.
Security News
Research
The Socket Research Team breaks down a malicious wrapper package that uses obfuscation to harvest credentials and exfiltrate sensitive data.
Research
Security News
Attackers used a malicious npm package typosquatting a popular ESLint plugin to steal sensitive data, execute commands, and exploit developer systems.
Security News
The Ultralytics' PyPI Package was compromised four times in one weekend through GitHub Actions cache poisoning and failure to rotate previously compromised API tokens.