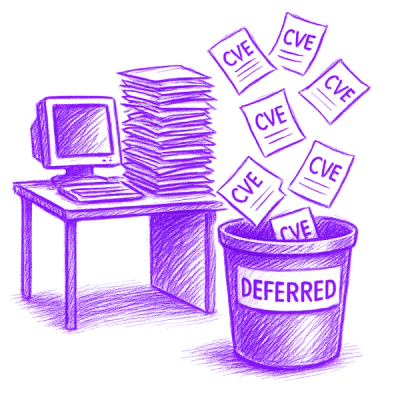
Security News
NVD Quietly Sweeps 100K+ CVEs Into a “Deferred” Black Hole
NVD now marks all pre-2018 CVEs as "Deferred," signaling it will no longer enrich older vulnerabilities, further eroding trust in its data.
Scikit-multilearn is a BSD-licensed library for multi-label classification that is built on top of the well-known scikit-learn ecosystem.
scikit-multilearn is a Python module capable of performing multi-label learning tasks. It is built on-top of various scientific Python packages (numpy, scipy) and follows a similar API to that of scikit-learn.
Native Python implementation. A native Python implementation for a variety of multi-label classification algorithms. To see the list of all supported classifiers, check this link.
Interface to Meka. A Meka wrapper class is implemented for reference purposes and integration. This provides access to all methods available in MEKA, MULAN, and WEKA — the reference standard in the field.
Builds upon giants! Team-up with the power of numpy and scikit. You can use scikit-learn's base classifiers as scikit-multilearn's classifiers. In addition, the two packages follow a similar API.
In most cases you will want to follow the requirements defined in the requirements/*.txt files in the package.
scipy
numpy
future
scikit-learn
liac-arff # for loading ARFF files
requests # for dataset module
networkx # for networkX base community detection clusterers
python-louvain # for networkX base community detection clusterers
keras
python-igraph # for igraph library based clusterers
python-graphtool # for graphtool base clusterers
Note: Installing graphtool is complicated, please see: graphtool install instructions
To install scikit-multilearn, simply type the following command:
$ pip install scikit-multilearn
This will install the latest release from the Python package index. If you
wish to install the bleeding-edge version, then clone this repository and
run setup.py
:
$ git clone https://github.com/scikit-multilearn/scikit-multilearn.git
$ cd scikit-multilearn
$ python setup.py
Before proceeding to classification, this library assumes that you have a dataset with the following matrices:
x_train
, x_test
: training and test feature matrices of size (n_samples, n_features)
y_train
, y_test
: training and test label matrices of size (n_samples, n_labels)
Suppose we wanted to use a problem-transformation method called Binary Relevance, which treats each label as a separate single-label classification problem, to a Support-vector machine (SVM) classifier, we simply perform the following tasks:
# Import BinaryRelevance from skmultilearn
from skmultilearn.problem_transform import BinaryRelevance
# Import SVC classifier from sklearn
from sklearn.svm import SVC
# Setup the classifier
classifier = BinaryRelevance(classifier=SVC(), require_dense=[False,True])
# Train
classifier.fit(X_train, y_train)
# Predict
y_pred = classifier.predict(X_test)
More examples and use-cases can be seen in the documentation. For using the MEKA wrapper, check this link.
This project is open for contributions. Here are some of the ways for you to contribute:
In case you want to implement your own multi-label classifier, please read our Developer's Guide to help you integrate your implementation in our API.
To make a contribution, just fork this repository, push the changes in your fork, open up an issue, and make a Pull Request!
We're also available in Slack! Just go to our slack group.
If you used scikit-multilearn in your research or project, please cite our work:
@ARTICLE{2017arXiv170201460S,
author = {{Szyma{\'n}ski}, P. and {Kajdanowicz}, T.},
title = "{A scikit-based Python environment for performing multi-label classification}",
journal = {ArXiv e-prints},
archivePrefix = "arXiv",
eprint = {1702.01460},
year = 2017,
month = feb
}
FAQs
Scikit-multilearn is a BSD-licensed library for multi-label classification that is built on top of the well-known scikit-learn ecosystem.
We found that scikit-multilearn demonstrated a healthy version release cadence and project activity because the last version was released less than a year ago. It has 2 open source maintainers collaborating on the project.
Did you know?
Socket for GitHub automatically highlights issues in each pull request and monitors the health of all your open source dependencies. Discover the contents of your packages and block harmful activity before you install or update your dependencies.
Security News
NVD now marks all pre-2018 CVEs as "Deferred," signaling it will no longer enrich older vulnerabilities, further eroding trust in its data.
Research
Security News
Lazarus-linked threat actors expand their npm malware campaign with new RAT loaders, hex obfuscation, and over 5,600 downloads across 11 packages.
Security News
Safari 18.4 adds support for Iterator Helpers and two other TC39 JavaScript features, bringing full cross-browser coverage to key parts of the ECMAScript spec.