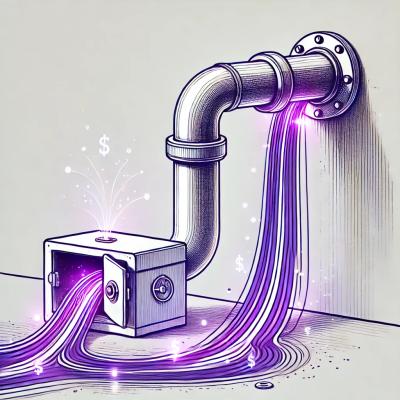
Research
Security News
Malicious npm Package Targets Solana Developers and Hijacks Funds
A malicious npm package targets Solana developers, rerouting funds in 2% of transactions to a hardcoded address.
Generate ai qr codes with stable diffusion and controlnet with standardised methods
alpha version, expect breaking changes
call diffusers pipeline or Automatic1111 webui api to generate qrcodes.
import sdqrcode
sd_qr_images, generator = sdqrcode.init_and_generate_sd_qrcode(config="default_diffusers")
Engine | Colab |
---|---|
Diffusers | |
Automatic1111 |
There is multiple methodes availables to generate ai qr code with differents controlnets models and params. Some parameters might works better with some stable diffusion checkpoints and it's a pain to find somethings that works consistanly. This repo aims to easily try and evaluate differents methods, models, params and share them with a simple config file
The idea is to use controlnet to guide the generation:
With this method, small modifications of the weight
, start
and end
parameters can have huge impacts on the generation.
click to expand, cherry picked, will add more results later
pip install sdqrcode # Automatic1111 engine
#or
pip install sdqrcode[diffusers] # Diffusers engine
pip install git+https://github.com/huggingface/diffusers # require dev version of diffusers
import sdqrcode
# init with a default config
generator = sdqrcode.init(config = "default_diffusers")
# or with a custom config
generator = sdqrcode.init(config = "/path/to/config.yaml")
# or you can also set custom config params (base model, controlnet models, steps, ...)
generator = sdqrcode.init(config = "default_diffusers", model_name_or_path="Lykon/DreamShaper")
# Then you can generate according to the config
images = generator.generate_sd_qrcode()
# or with some custom parameters (you can't set the models at this stage)
images = generator.generate_sd_qrcode(
prompt = "A beautiful minecraft landscape",
steps = 30,
cfg_scale = 7 ,
width = 768,
height = 768,
seed = -1,
controlnet_weights = [0.35, 0.65], # [weight_cn_1, weight_cn_2, ...]
controlnet_startstops = [(0,1), (0.35, 0.7)], # [(start_cn_1, end_cn_1), ... ]. (0.35, 0.7) means apply CN after 35% of total steps until 70% of total steps
qrcode_text = "https://koll.ai" ,
qrcode_error_correction = "high",
qrcode_box_size = 10,
qrcode_border = 4,
qrcode_fill_color = "black",
qrcode_back_color = "white",
)
import sdqrcode
# Use an auto config and define the auto_* params in init to use Automatic1111 backend
generator = sdqrcode.init(
config_name_or_path = "default_auto",
auto_api_hostname = "auto_hostname",
auto_api_port=7860,
auto_api_https = True,
auto_api_username = "auto_user",
auto_api_password = "auto_pass"
)
# Then you can generate like the diffusers version
images = generator.generate_sd_qrcode()
# get available models
generator.engine.api.util_get_model_names()
# get available controlnet modules
generator.engine.api.controlnet_module_list()
# get available controlnet models
generator.engine.api.controlnet_model_list()
import sdqrcode
sdqrcode.CONFIGS()
This lib uses a yaml file to describe the qrcode generation process. You can change any parameters to experiment. Exemple:
global:
mode: txt2img
prompt: "a beautiful minecraft landscape, lights and shadows"
negative_prompt: "ugly"
model_name_or_path: "SG161222/Realistic_Vision_V2.0"
steps: 20
scheduler_name: Euler a
cfg_scale: 7
width: 768
height: 768
seed: -1
batch_size: 1
input_image: qrcode # img2img mode only
denoising_strength: 0.7 # img2img mode only
controlnet_units:
brightness:
model: ioclab/control_v1p_sd15_brightness
cn_input_image: qrcode
module: none #not implemented yet for diffusers
weight: 0.35
start: 0.0
end: 1.0
tile:
model: lllyasviel/control_v11f1e_sd15_tile
module: none #not implemented yet for diffusers
cn_input_image: qrcode
weight: 0.5
start: 0.35
end: 0.70
qrcode:
text: "https://koll.ai"
error_correction: high # [low, medium, quart, high]
box_size: 10
border: 4
fill_color: black
back_color: white
global
mode
: txt2img or img2img (str)
prompt
: the prompt to use (str)
negative_prompt
: the negative prompt to use (str)
model_name_or_path
: stable diffusion checkpoint to use (str)
steps
: the number of steps (int)
scheduler_name
: the scheduler to use (str)
DDIM
, Euler
, Euler a
, LMS
, DPM2 Karras
, DPM2 a Karras
, Heun
, DDPM
, UniPC
, PNDM
, DEI
, DPM++ SDE
, DPM++ 2S a
, DPM++ 2M
, DPM++ SDE Karras
, DPM++ 2S a Karras
, DPM++ 2M Karras
cfg_scale
: the cfg scale (float)
width
: the width of the output image (int)
height
: the height of the output image (int)
seed
: the seed to use, -1 for random (int)
batch_size
: the batch size (int)
input_image
: local path or url of the input image, or qrcode
img2img only (str)
denoising_strength
: the denoising strength, img2img only (float)
controlnet_units
: the controlnet units to use The unit name (tile, brightness, in above exemple) is used for better readability and does not impact the generation
model
: the controlnet model to use (str)
module
: the controlnet module to use (str)
cn_input_image
: (str) can be
qrcode
to use the qrcode as input imageweight
: the weight of the controlnet (float)start
: when the controlnet starts applying, in fract of total steps (ex: 0.35 means "start after 35% of total steps are done") (float)end
: when the controlnet stops applying, in fract of total steps (ex: 0.7 means "end after 70% of total steps are done") (float)qrcode
: the qrcode parameters
text
: the text to encode (str)error_correction
: the error correction level (str)box_size
: the box size (int)border
: the border size (int)fill_color
: the fill color (str)back_color
: the back color (str)This method seem to be the best for me, I use it with the model realistic_visionV2. It uses Controlnet Brightness and Controlnet Tile Here are my firsts thoughts:
There are multiple controlnet models that can be used:
Please don't hesitate to submit a PR to improve the code or submit a config
You can checkout our website to discover more of our projects such as:
FAQs
Generate ai qr codes with stable diffusion and controlnet with standardised methods
We found that sdqrcode demonstrated a healthy version release cadence and project activity because the last version was released less than a year ago. It has 1 open source maintainer collaborating on the project.
Did you know?
Socket for GitHub automatically highlights issues in each pull request and monitors the health of all your open source dependencies. Discover the contents of your packages and block harmful activity before you install or update your dependencies.
Research
Security News
A malicious npm package targets Solana developers, rerouting funds in 2% of transactions to a hardcoded address.
Security News
Research
Socket researchers have discovered malicious npm packages targeting crypto developers, stealing credentials and wallet data using spyware delivered through typosquats of popular cryptographic libraries.
Security News
Socket's package search now displays weekly downloads for npm packages, helping developers quickly assess popularity and make more informed decisions.