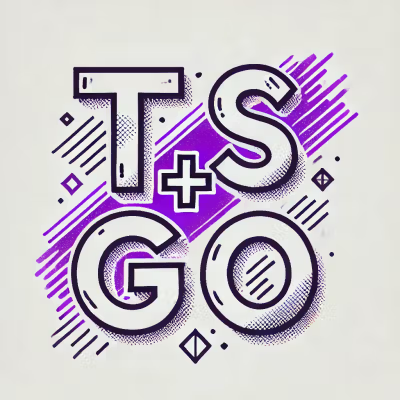
Security News
TypeScript is Porting Its Compiler to Go for 10x Faster Builds
TypeScript is porting its compiler to Go, delivering 10x faster builds, lower memory usage, and improved editor performance for a smoother developer experience.
pip install season-polar-plots
This is a package to create polar plots for displaying seasonal trends in time series data. Requires
>>> from season_polar_plots import SeasonData
Parameters | |
---|---|
data | pandas Series or single column DataFrame with datetime index |
year_start | (int) start year of period to be analyzed |
year_end | (int) end year of period to be analyzed |
t_res | (str) 'daily' or 'monthly': temporal resolution. Monthly values can be aggregated from daily values if 'monthly' is chosen (see sp_plot() and get_mgrid() function) |
Parameters | |
---|---|
label | (str) label for time series variable |
mode | (str) 'all' uses all data points; daily resolution: 'min' or 'max' filter time series on annual extreme values; monthly resolution: 'sum', 'mean' / 'min', 'max' aggregate / filter data for each month |
rd_years | (bool) as default, years are plotted in radius direction; rd_years=False plots variable in radius direction |
col | (str) color gradient (default 'viridis_r') |
a | (float) transparency alpha (0-1) |
psize | (float) marker size for daily data points |
pmarker | (MarkerStyle) marker style for daily data points |
nylabels | (int) number of (year) labels in radius direction |
off | (int or float) off-set from circle center |
rlab_angle | (float) angle of the radius axis labels |
linreg | (bool) if True plots linear regression (day in year ~ year) in polar projection (only for daily extreme values) |
start_month | (int) start month for linear regression |
Returns: Plot; if linreg=True prints R² and p-values for slope and intercept
Parameters | |
---|---|
mode | (str) 'min' or 'max': filter time series on annual extreme values |
Returns: DataFrame (containing nr of day in year of extreme values, extreme values)
Parameters | |
---|---|
mode | (str) 'all' if data is already in monthly resolution; 'sum', 'mean' / 'min', 'max' aggregate / filter data for each month |
Returns: DataFrame (containing monthly data)
Parameters | |
---|---|
mode | (str) 'all' to use all data points, 'min' or 'max' to filter on extreme values |
plot | (bool) if True plots the data as circular histogram and fitted von-Mises distribution |
print_par | (bool) if True prints the fitted von-Mises parameters kappa and mu |
bins | (int) number of bins for histogram |
col_hist | (str) color of the histogram |
col_vm | (str) color of the fitted von Mises distribution |
off | (float) off-set from circle center |
a | (float) transparency alpha (0-1) |
rwidth | (float) relative width of histogram bins |
Returns: Plot; kappa, mu, mu_nday
FAQs
Create polar plots to display seasonal trends in time series data.
We found that season-polar-plots demonstrated a healthy version release cadence and project activity because the last version was released less than a year ago. It has 1 open source maintainer collaborating on the project.
Did you know?
Socket for GitHub automatically highlights issues in each pull request and monitors the health of all your open source dependencies. Discover the contents of your packages and block harmful activity before you install or update your dependencies.
Security News
TypeScript is porting its compiler to Go, delivering 10x faster builds, lower memory usage, and improved editor performance for a smoother developer experience.
Research
Security News
The Socket Research Team has discovered six new malicious npm packages linked to North Korea’s Lazarus Group, designed to steal credentials and deploy backdoors.
Security News
Socket CEO Feross Aboukhadijeh discusses the open web, open source security, and how Socket tackles software supply chain attacks on The Pair Program podcast.