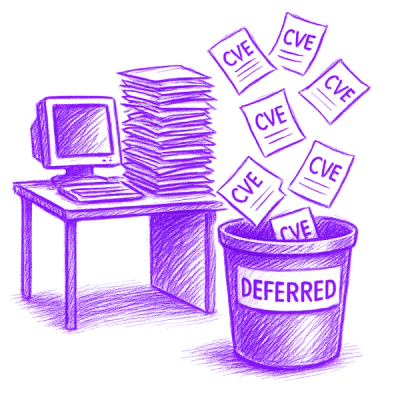
Security News
NVD Quietly Sweeps 100K+ CVEs Into a “Deferred” Black Hole
NVD now marks all pre-2018 CVEs as "Deferred," signaling it will no longer enrich older vulnerabilities, further eroding trust in its data.
SO4GP stands for: "Some Optimizations for Gradual Patterns". SO4GP applies optimizations such as swarm intelligence, HDF5 chunks, cluster analysis and many others in order to improve the efficiency of extracting gradual patterns. It provides Python algorithm implementations for these optimization techniques. The algorithm implementations include:
A GP (Gradual Pattern) is a set of gradual items (GI) and its quality is measured by its computed support value. For example given a data set with 3 columns (age, salary, cars) and 10 objects. A GP may take the form: {age+, salary-} with a support of 0.8. This implies that 8 out of 10 objects have the values of column age 'increasing' and column 'salary' decreasing.
Before running so4gp, make sure you install the following Python Packages
:
pip3 install numpy>=1.23.2 pandas>=1.4.4 python-dateutil>=2.8.2 ypstruct>=0.0.2 scikit-learn>=1.1.2
In order to run each algorithm for the purpose of extracting GPs, follow the instructions that follow.
First and foremost, import the so4gp python package via:
import so4gp as sgp
This is the classical approach (initially proposed by Anne Laurent) for mining gradual patterns. All the remaining algorithms are variants of this algorithm.
mine_obj = sgp.GRAANK(data_source=f_path, min_sup=0.5, eq=False)
gp_json = mine_obj.discover()
print(gp_json)
where you specify the parameters as follows:
file in csv format
or a Pandas DataFrame
}default = 0.5
default = False
In this approach, it is assumed that every column can be converted into gradual item (GI). If the GI is valid (i.e. its computed support is greater than the minimum support threshold) then it is either increasing or decreasing (+ or -), otherwise it is irrelevant (x). Therefore, a pheromone matrix is built using the number of columns and the possible variations (increasing, decreasing, irrelevant) or (+, -, x). The algorithm starts by randomly generating GP candidates using the pheromone matrix, each candidate is validated by confirming that its computed support is greater or equal to the minimum support threshold. The valid GPs are used to update the pheromone levels and better candidates are generated.
mine_obj = sgp.AntGRAANK(data_src)
gp_json = mine_obj.discover()
print(gp_json)
where you specify the parameters as follows:
file in csv format
or a Pandas DataFrame
}default = 0.5
default = 1
default = 0.5
In this approach, it is assumed that every GP candidate may be represented as a binary gene (or individual) that has a unique position and cost. The cost is derived from the computed support of that candidate, the higher the support value the lower the cost. The aim of the algorithm is search through a population of individuals (or candidates) and find those with the lowest cost as efficiently as possible.
mine_obj = sgp.GeneticGRAANK(data_src)
gp_json = mine_obj.discover()
print(gp_json)
where you specify the parameters as follows:
file in csv format
or a Pandas DataFrame
}default = 0.5
default = 1
default = 5
default = 0.5
default = 1
default = 0.9
default = 0.9
In this approach, it is assumed that every GP candidate may be represented as a particle that has a unique position and fitness. The fitness is derived from the computed support of that candidate, the higher the support value the higher the fitness. The aim of the algorithm is search through a population of particles (or candidates) and find those with the highest fitness as efficiently as possible.
mine_obj = sgp.ParticleGRAANK(data_src)
gp_json = mine_obj.discover()
print(gp_json)
where you specify the parameters as follows:
file in csv format
or a Pandas DataFrame
}default = 0.5
default = 1
default = 5
default = 0.9
default = 0.01
default = 0.9
In this approach, it is assumed that every GP candidate may be represented as a position that has a cost value associated with it. The cost is derived from the computed support of that candidate, the higher the support value the lower the cost. The aim of the algorithm is search through group of positions and find those with the lowest cost as efficiently as possible.
mine_obj = sgp.HillClimbingGRAANK(data_src, min_sup)
gp_json = mine_obj.discover()
print(gp_json)
where you specify the parameters as follows:
file in csv format
or a Pandas DataFrame
}default = 0.5
default = 1
default = 0.5
In this approach, it is assumed that every GP candidate may be represented as a position that has a cost value associated with it. The cost is derived from the computed support of that candidate, the higher the support value the lower the cost. The aim of the algorithm is search through group of positions and find those with the lowest cost as efficiently as possible.
import so4gp as sgp
mine_obj = sgp.RandomGRAANK(data_src, min_sup)
gp_json = mine_obj.discover()
print(gp_json)
where you specify the parameters as follows:
file in csv format
or a Pandas DataFrame
}default = 0.5
default = 1
We borrow the net-win concept used in the work 'Clustering Using Pairwise Comparisons' proposed by R. Srikant to the problem of extracting gradual patterns (GPs). In order to mine for GPs, each feature yields 2 gradual items which we use to construct a bitmap matrix comparing each row to each other (i.e., (r1,r2), (r1,r3), (r1,r4), (r2,r3), (r2,r4), (r3,r4)).
In this approach, we convert the bitmap matrices into 'net-win vectors'. Finally, we apply spectral clustering to determine which gradual items belong to the same group based on the similarity of net-win vectors. Gradual items in the same cluster should have almost similar score vector.
import so4gp as sgp
mine_obj = sgp.ClusterGP(data_source=data_src, min_sup=0.5, e_prob=0.1)
gp_json = mine_obj.discover()
print(gp_json)
where you specify the parameters as follows:
file in csv format
or a Pandas DataFrame
}default = 0.5
default = 0.5
default = 10
The default output is the format of JSON:
{
"Algorithm": "RS-GRAANK",
"Best Patterns": [
[["Age+", "Salary+"], 0.6],
[["Expenses-", "Age+", "Salary+"], 0.6]
],
"Iterations": 20
}
FAQs
Some Python optimization algorithms for mining gradual patterns.
We found that so4gp demonstrated a healthy version release cadence and project activity because the last version was released less than a year ago. It has 1 open source maintainer collaborating on the project.
Did you know?
Socket for GitHub automatically highlights issues in each pull request and monitors the health of all your open source dependencies. Discover the contents of your packages and block harmful activity before you install or update your dependencies.
Security News
NVD now marks all pre-2018 CVEs as "Deferred," signaling it will no longer enrich older vulnerabilities, further eroding trust in its data.
Research
Security News
Lazarus-linked threat actors expand their npm malware campaign with new RAT loaders, hex obfuscation, and over 5,600 downloads across 11 packages.
Security News
Safari 18.4 adds support for Iterator Helpers and two other TC39 JavaScript features, bringing full cross-browser coverage to key parts of the ECMAScript spec.