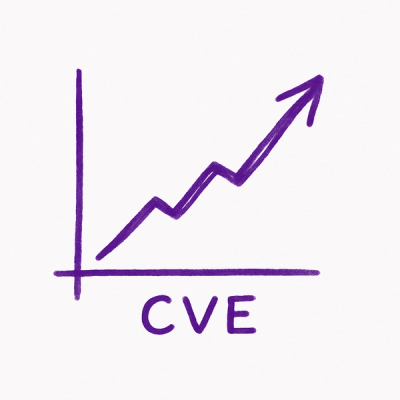
Security News
New CVE Forecasting Tool Predicts 47,000 Disclosures in 2025
CVEForecast.org uses machine learning to project a record-breaking surge in vulnerability disclosures in 2025.
What this repo do:
I build this repo while I'm writing my paper for INTERSPEECH 2022: Neural Vocoder is All You Need for Speech Super-resolution. The model mentioned in this paper, NVSR, will also be open-sourced here.
Install via pip:
pip3 install ssr_eval
Please make sure you have already installed sox.
A basic example: Evaluate on a system that do nothing:
from ssr_eval import test
test()
"averaged": {
"proc_fft_24000_44100": {
"lsd": 5.152331300436993,
"log_sispec": 5.8051057146229095,
"sispec": 30.23394207533686,
"ssim": 0.8484425044157442
}
}
Here we report four metrics:
:warning: LSD is the most widely used metric for super-resolution. And I include another three metrics just in case you need them.
Below is the code of test()
from ssr_eval import SSR_Eval_Helper, BasicTestee
# You need to implement a class for the model to be evaluated.
class MyTestee(BasicTestee):
def __init__(self) -> None:
super().__init__()
# You need to implement this function
def infer(self, x):
"""A testee that do nothing
Args:
x (np.array): [sample,], with model_input_sr sample rate
target (np.array): [sample,], with model_output_sr sample rate
Returns:
np.array: [sample,]
"""
return x
def test():
testee = MyTestee()
# Initialize a evaluation helper
helper = SSR_Eval_Helper(
testee,
test_name="unprocessed", # Test name for storing the result
input_sr=44100, # The sampling rate of the input x in the 'infer' function
output_sr=44100, # The sampling rate of the output x in the 'infer' function
evaluation_sr=48000, # The sampling rate to calculate evaluation metrics.
setting_fft={
"cutoff_freq": [
12000
], # The cutoff frequency of the input x in the 'infer' function
},
save_processed_result=True
)
# Perform evaluation
## Use all eight speakers in the test set for evaluation (limit_test_speaker=-1)
## Evaluate on 10 utterance for each speaker (limit_test_nums=10)
helper.evaluate(limit_test_nums=10, limit_test_speaker=-1)
The code will automatically handle stuffs like downloading test sets. The evaluation result will be saved in the ./results directory.
We provide several pretrained baselines. For example, to run the NVSR baseline, you can click the link in the following table for more details.
Table.1 Log-spectral distance (LSD) on different input sampling-rate (Evaluated on 44.1kHz).
Method | One for all | Params | 2kHz | 4kHz | 8kHz | 12kHz | 16kHz | 24kHz | 32kHz | AVG |
---|---|---|---|---|---|---|---|---|---|---|
NVSR [Pretrained Model] | Yes | 99.0M | 1.04 | 0.98 | 0.91 | 0.85 | 0.79 | 0.70 | 0.60 | 0.84 |
WSRGlow(24kHz→48kHz) | No | 229.9M | - | - | - | - | - | 0.79 | - | - |
WSRGlow(12kHz→48kHz) | No | 229.9M | - | - | - | 0.87 | - | - | - | - |
WSRGlow(8kHz→48kHz) | No | 229.9M | - | - | 0.98 | - | - | - | - | - |
WSRGlow(4kHz→48kHz) | No | 229.9M | - | 1.12 | - | - | - | - | - | - |
Nu-wave(24kHz→48kHz) | No | 3.0M | - | - | - | - | - | 1.22 | - | - |
Nu-wave(12kHz→48kHz) | No | 3.0M | - | - | - | 1.40 | - | - | - | - |
Nu-wave(8kHz→48kHz) | No | 3.0M | - | - | 1.42 | - | - | - | - | - |
Nu-wave(4kHz→48kHz) | No | 3.0M | - | 1.42 | - | - | - | - | - | - |
Unprocessed | - | - | 5.69 | 5.50 | 5.15 | 4.85 | 4.54 | 3.84 | 2.95 | 4.65 |
Click the link of the model for more details.
Here "one for all" means model can process flexible input sampling rate.
The following code demonstrate the full options in the SSR_Eval_Helper:
testee = MyTestee()
helper = SSR_Eval_Helper(testee, # Your testsee object with 'infer' function implemented
test_name="unprocess", # The name of this test. Used for saving the log file in the ./results directory
test_data_root="./your_path/vctk_test", # The directory to store the test data, which will be automatically downloaded.
input_sr=44100, # The sampling rate of the input x in the 'infer' function
output_sr=44100, # The sampling rate of the output x in the 'infer' function
evaluation_sr=48000, # The sampling rate to calculate evaluation metrics.
save_processed_result=False, # If True, save model output in the dataset directory.
# (Recommend/Default) Use fourier method to simulate low-resolution effect
setting_fft = {
"cutoff_freq": [1000, 2000, 4000, 6000, 8000, 12000, 16000], # The cutoff frequency of the input x in the 'infer' function
},
# Use lowpass filtering to simulate low-resolution effect. All possible combinations will be evaluated.
setting_lowpass_filtering = {
"filter":["cheby","butter","bessel","ellip"], # The type of filter
"cutoff_freq": [1000, 2000, 4000, 6000, 8000, 12000, 16000],
"filter_order": [3,6,9] # Filter orders
},
# Use subsampling method to simulate low-resolution effect
setting_subsampling = {
"cutoff_freq": [1000, 2000, 4000, 6000, 8000, 12000, 16000],
},
# Use mp3 compression method to simulate low-resolution effect
setting_mp3_compression = {
"low_kbps": [32, 48, 64, 96, 128],
},
)
helper.evaluate(limit_test_nums=10, # For each speaker, only evaluate on 10 utterances.
limit_test_speaker=-1 # Evaluate on all the speakers.
)
:warning: I recommand all the users to use fourier method (setting_fft) to simulate low-resolution effect for the convinence of comparing between different system.
We build the test sets using VCTK (version 0.92), a multi-speaker English corpus that contains 110 speakers with different accents.
If you find this repo useful for your research, please consider citing:
@misc{liu2022neural,
title={Neural Vocoder is All You Need for Speech Super-resolution},
author={Haohe Liu and Woosung Choi and Xubo Liu and Qiuqiang Kong and Qiao Tian and DeLiang Wang},
year={2022},
eprint={2203.14941},
archivePrefix={arXiv},
primaryClass={eess.AS}
}
[1] Liu, Haohe, et al. "VoiceFixer: Toward General Speech Restoration with Neural Vocoder." arXiv preprint arXiv:2109.13731 (2021).
FAQs
This package is written for the evaluation of speech super-resolution algorithms.
We found that ssr-eval demonstrated a healthy version release cadence and project activity because the last version was released less than a year ago. It has 1 open source maintainer collaborating on the project.
Did you know?
Socket for GitHub automatically highlights issues in each pull request and monitors the health of all your open source dependencies. Discover the contents of your packages and block harmful activity before you install or update your dependencies.
Security News
CVEForecast.org uses machine learning to project a record-breaking surge in vulnerability disclosures in 2025.
Security News
Browserslist-rs now uses static data to reduce binary size by over 1MB, improving memory use and performance for Rust-based frontend tools.
Research
Security News
Eight new malicious Firefox extensions impersonate games, steal OAuth tokens, hijack sessions, and exploit browser permissions to spy on users.